Emulating Human Cognitive Processes for Expert-Level Medical Question-Answering with Large Language Models
CoRR(2023)
摘要
In response to the pressing need for advanced clinical problem-solving tools in healthcare, we introduce BooksMed, a novel framework based on a Large Language Model (LLM). BooksMed uniquely emulates human cognitive processes to deliver evidence-based and reliable responses, utilizing the GRADE (Grading of Recommendations, Assessment, Development, and Evaluations) framework to effectively quantify evidence strength. For clinical decision-making to be appropriately assessed, an evaluation metric that is clinically aligned and validated is required. As a solution, we present ExpertMedQA, a multispecialty clinical benchmark comprised of open-ended, expert-level clinical questions, and validated by a diverse group of medical professionals. By demanding an in-depth understanding and critical appraisal of up-to-date clinical literature, ExpertMedQA rigorously evaluates LLM performance. BooksMed outperforms existing state-of-the-art models Med-PaLM 2, Almanac, and ChatGPT in a variety of medical scenarios. Therefore, a framework that mimics human cognitive stages could be a useful tool for providing reliable and evidence-based responses to clinical inquiries.
更多查看译文
关键词
large language models,medical
AI 理解论文
溯源树
样例
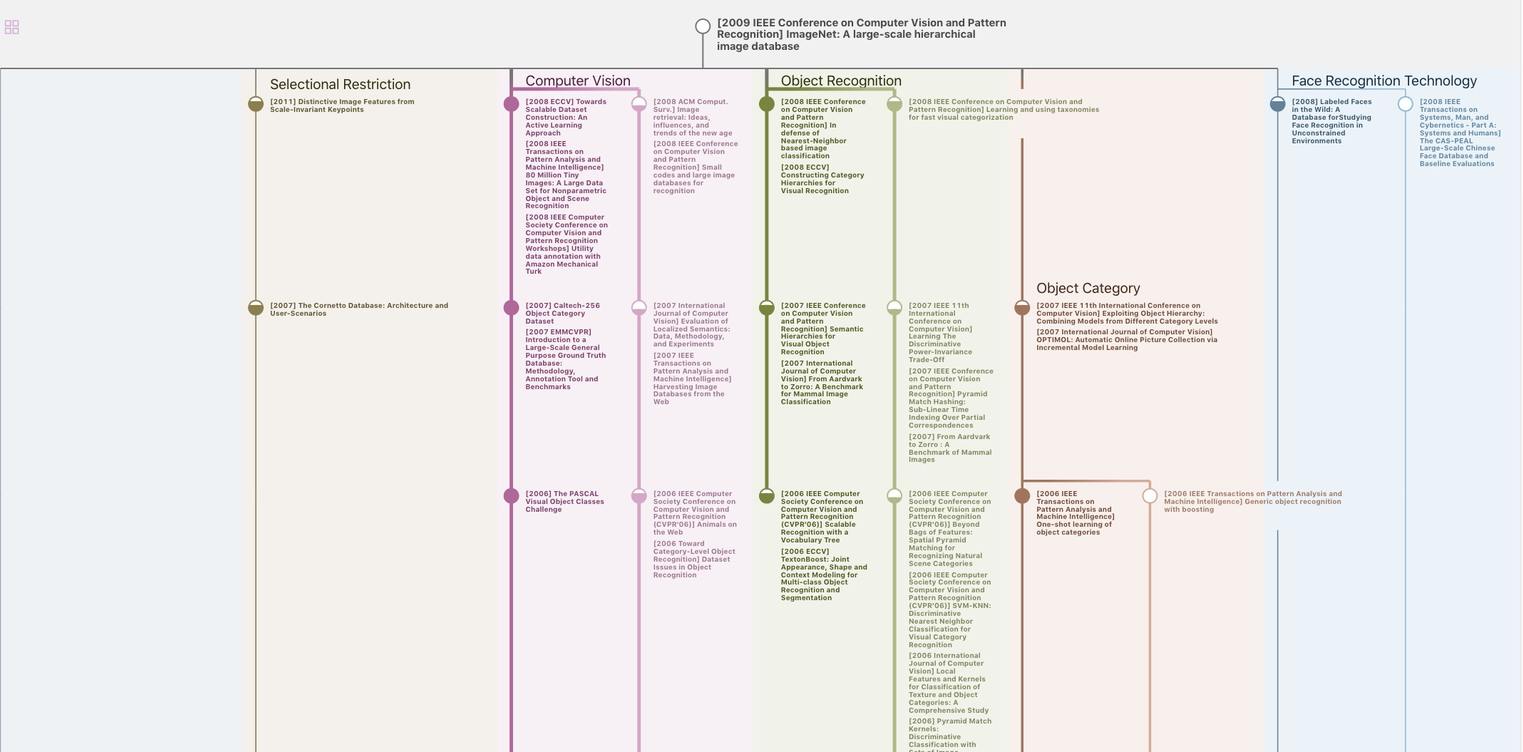
生成溯源树,研究论文发展脉络
Chat Paper
正在生成论文摘要