Dual Graph Networks for Pose Estimation in Crowded Scenes
International Journal of Computer Vision(2024)
摘要
Pose estimation in crowded scenes is key to understanding human behavior in real-life applications. Most existing CNN-based pose estimation methods often depend on the appearance of visible parts as cues to localize human joints. However, occlusion is typical in crowded scenes, and invisible body parts have no valid features for joint localization. Introducing prior information about the human pose structure to infer the locations of occluded parts is a natural solution to this problem. In this paper, we argue that learning structural information based on human joints alone is not enough to address human body variations and could be prone to overfitting. From a perspective on the human pose as a dual representation of joints and limbs, we propose a pose refinement network , coined as dual graph network (DGN), to jointly learn its structural information of body joints and limbs by incorporating the cooperative constraints between two branches. Specifically, our DGN has two coupled graph convolutional network (GCN) branches to model the structure information of joints and limbs. Each stage in the branch is composed of a feature aggregator and a GCN module for inter-branch information fusion and intra-branch context extraction, respectively. In addition, to enhance the modeling capacity of GCN, we design an adaptive GCN layer (AGL) embedded in the GCN module to handle each pose instance based on its graph structure. We also propose a heatmap-guided sampling to leverage the features of the body parts to provide rich visual features for the inference of occluded parts. We perform extensive experiments on five challenging datasets to demonstrate the effectiveness of our DGN on pose estimation. Our DGN obtains significant performance improvement from 67.9 to 72.4 mAP in the CrowdPose dataset with the same CNN-based pose estimator and training strategy as the OPEC-Net. It shows that, compared to the OPEC-Net only considering joints, our DGN has a clear advantage due to the joint consideration of both joints and limbs. Meanwhile, our DGN is also helpful for pose estimation in general datasets (i.e., COCO and Pose track) with less occlusion and mutual interference, demonstrating the generalization power of DGN on refining human poses.
更多查看译文
关键词
2D pose estimation,Graph convolutional networks,Dual GCN,Adaptive adjacency matrix
AI 理解论文
溯源树
样例
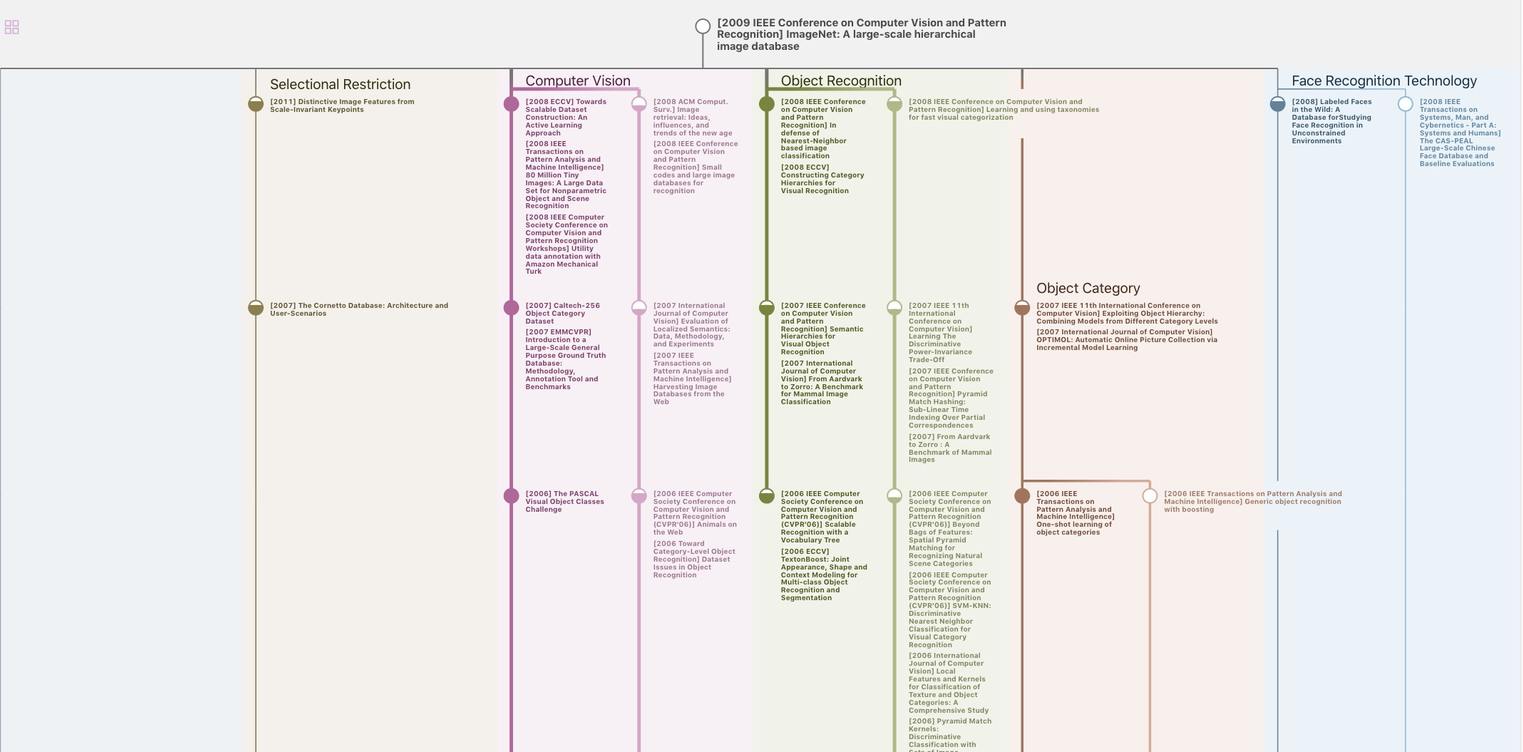
生成溯源树,研究论文发展脉络
Chat Paper
正在生成论文摘要