A many-objective evolutionary algorithm based on novel fitness estimation and grouping layering
Neural Computing and Applications(2023)
摘要
Many-objective optimization problems pose a great challenge for traditional Pareto-based multi-objective evolutionary algorithms (MOEAs), due to the loss of selection pressure (pressure that drives the population evolution) and the difficulty of diversity maintenance. To relieve the above challenge, this paper proposes a many-objective evolutionary algorithm based on novel fitness estimation and grouping layering (called MaOEA-FEGL). The novel fitness estimation mechanism can increase selection pressure and maintain diversity simultaneously by integrating the designed cos function-based convergence measure and adaptive mapping angle distance-based diversity measure. Meanwhile, a grouping layering strategy is proposed for further enhancing convergence and diversity, which divides individuals into different groups. Then, the individuals in each group are stratified into different layers by the R2 indicator. Based on the above mechanism and strategy, a two-round environmental selection strategy is designed to select elite individuals for the next generation. In addition, a variable classification-based initialization technique is designed to generate a high-quality initialization population and further provide a good start for the subsequent population evolution. Experimental studies on two well-known benchmark suites and two real-world engineering applications with objective numbers varying from 3 to 20 demonstrate that our algorithm is highly competitive compared with some state-of-the-art methods.
更多查看译文
关键词
Many-objective optimization,Evolutionary algorithm,Fitness estimation,Grouping layering,Variable classification initialization
AI 理解论文
溯源树
样例
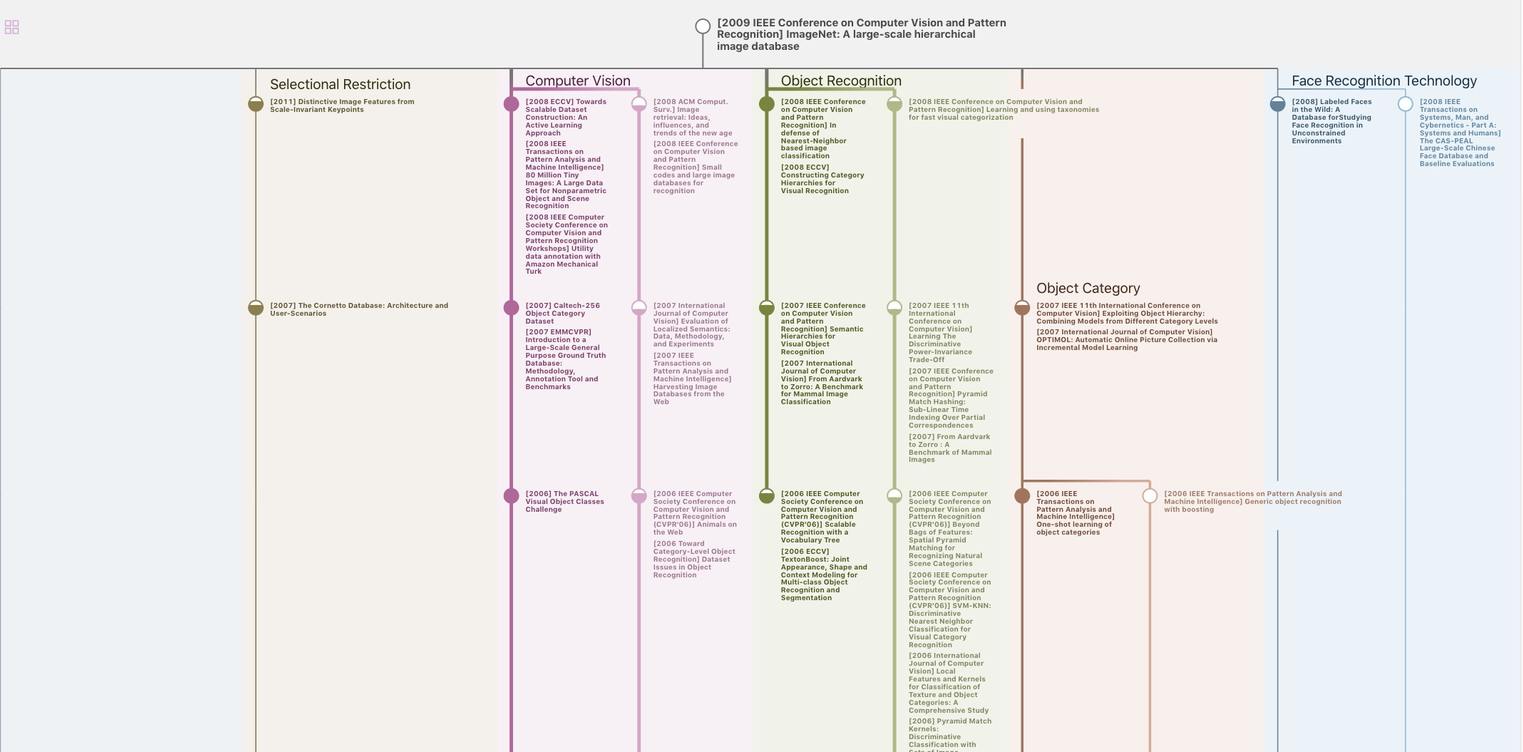
生成溯源树,研究论文发展脉络
Chat Paper
正在生成论文摘要