Eliciting Model Steering Interactions from Users via Data and Visual Design Probes
IEEE transactions on visualization and computer graphics(2023)
摘要
Domain experts increasingly use automated data science tools to incorporate machine learning (ML) models in their work but struggle to "debug" these models when they are incorrect. For these experts, semantic interactions can provide an accessible avenue to guide and refine ML models without having to programmatically dive into its technical details. In this research, we conduct an elicitation study using data and visual design probes to examine if and how experts with a spectrum of ML expertise use semantic interactions to update a simple classification model. We use our design probes to facilitate an interactive dialogue with 20 participants and codify their interactions as a set of target-interaction pairs. Interestingly, our findings revealed that many targets of semantic interactions do not directly map to ML model parameters, but instead aim to augment the data a model uses for training. We also identify reasons that participants would hesitate to interact with ML models, including burdens of cognitive load and concerns of injecting bias. Unexpectedly participants also saw the value of using semantic interactions to work collaboratively with members of their team. Participants with less ML expertise found this to be a useful mechanism for communicating their concerns to ML experts. This was an especially important observation, as our study also shows the different needs that correspond to diverse ML expertise. Collectively, we demonstrate that design probes are effective tools for proactively gathering the affordances that should be offered in an interactive machine learning system.
更多查看译文
关键词
Design Probes,Interactive Machine Learning,Model Steering,Semantic Interactions
AI 理解论文
溯源树
样例
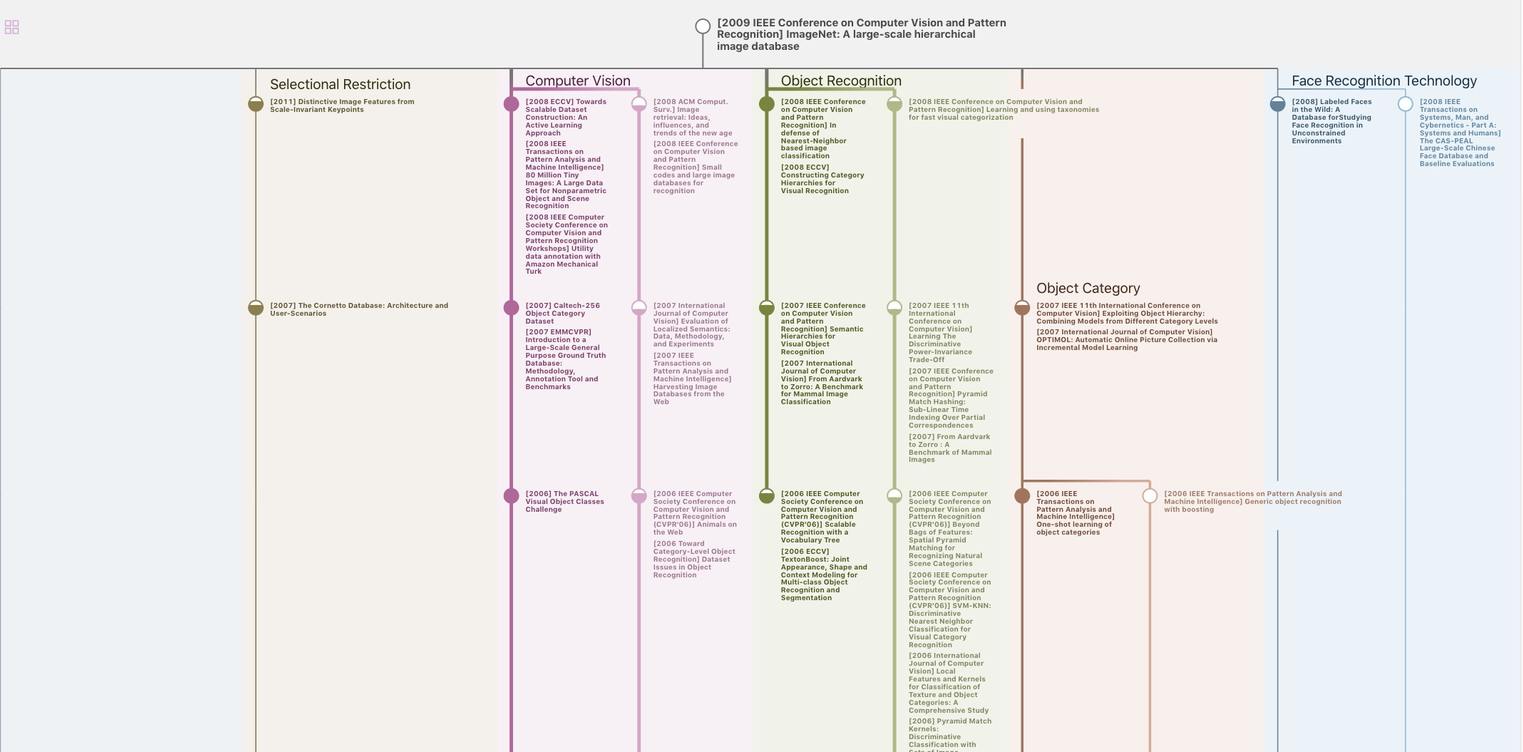
生成溯源树,研究论文发展脉络
Chat Paper
正在生成论文摘要