Language Models as Controlled Natural Language Semantic Parsers for Knowledge Graph Question Answering
ECAI 2023(2023)
摘要
We propose the use of controlled natural language as a target for knowledge graph question answering (KGQA) semantic parsing via language models as opposed to using formal query languages directly. Controlled natural languages are close to (human) natural languages, but can be unambiguously translated into a formal language such as SPARQL. Our research hypothesis is that the pre-training of large language models (LLMs) on vast amounts of textual data leads to the ability to parse into controlled natural language for KGQA with limited training data requirements. We devise an LLM-specific approach for semantic parsing to study this hypothesis. Our approach takes advantage of the hallucination and self-reflection capabilities of LLMs for relation linking. To conduct our study, we created a dataset that allows the comparison of one formal and two different controlled natural languages. Our analysis shows that training data requirements are indeed substantially reduced when using controlled natural languages, which is of paramount importance since collecting and maintaining high-quality KGQA semantic parsing training data is very expensive and time-consuming.
更多查看译文
关键词
knowledge,language
AI 理解论文
溯源树
样例
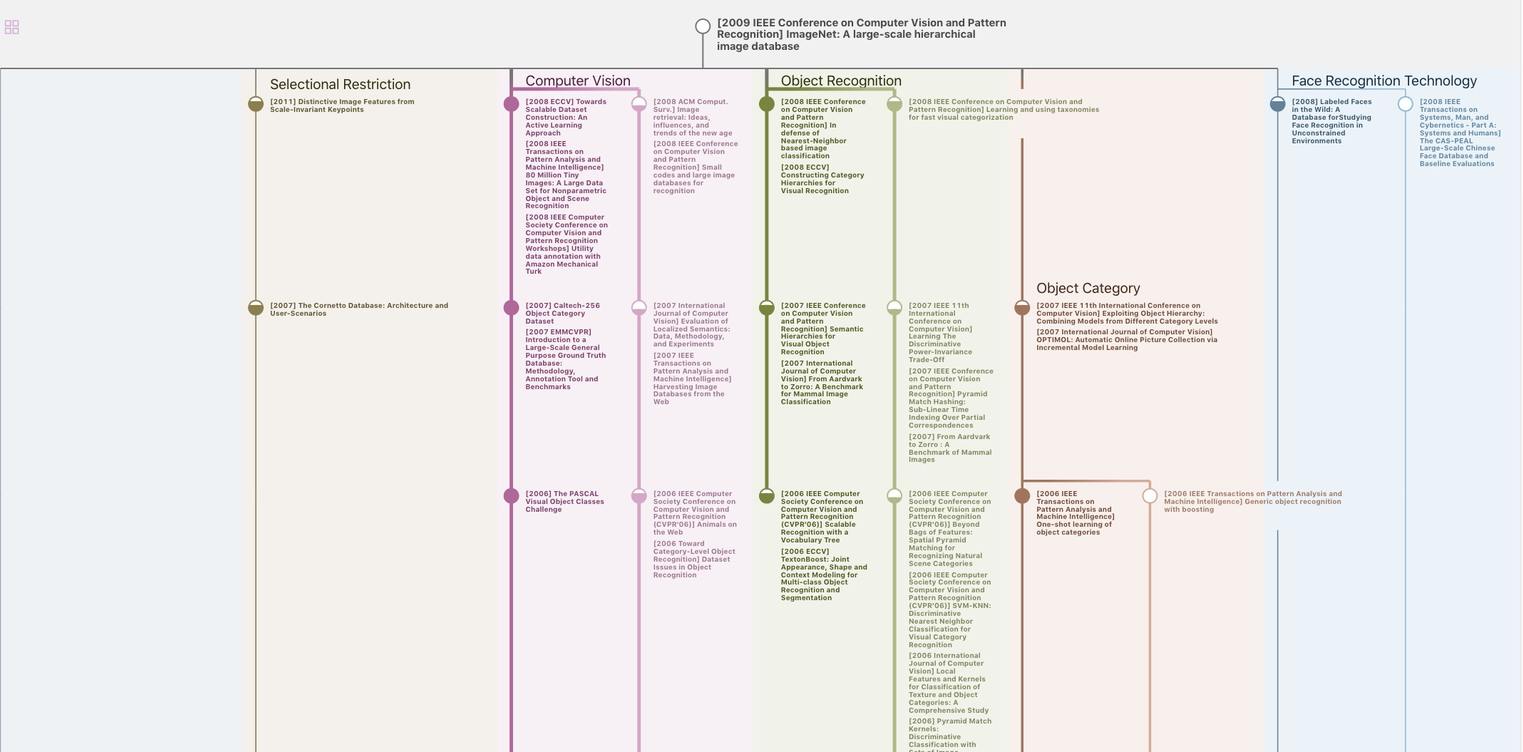
生成溯源树,研究论文发展脉络
Chat Paper
正在生成论文摘要