Story Ending Generation using Commonsense Casual Reasoning and Graph Convolutional Networks
ECAI 2023(2023)
摘要
Story Ending Generation is the task of generating a coherent and sensible ending for a given story. The key challenges of this task are i) how to obtain a good understanding of context, ii) how to capture hidden information between lines, and iii) how to obtain causal progression. However, recent machine learning-based models can only partially address these challenges due to the lack of causal entailment and consistency. The key novelty in our proposed approach is to capture the hidden story by generating transitional commonsense sentences between each adjacent context sentence, which substantially enriches causal and consistent story flow. Specifically, we adopt a soft causal relation using people's everyday commonsense knowledge to mimic the cognitive understanding process of readers. We then enrich the story with causal reasoning and utilize dependency parsing to capture long-range text relations. Finally, we apply multi-level Graph Convolutional Networks to deliver enriched contextual information across different layers. Both automatic and human evaluation results show that our proposed model can significantly improve the quality of generated story endings.
更多查看译文
关键词
commonsense casual reasoning,generation,networks
AI 理解论文
溯源树
样例
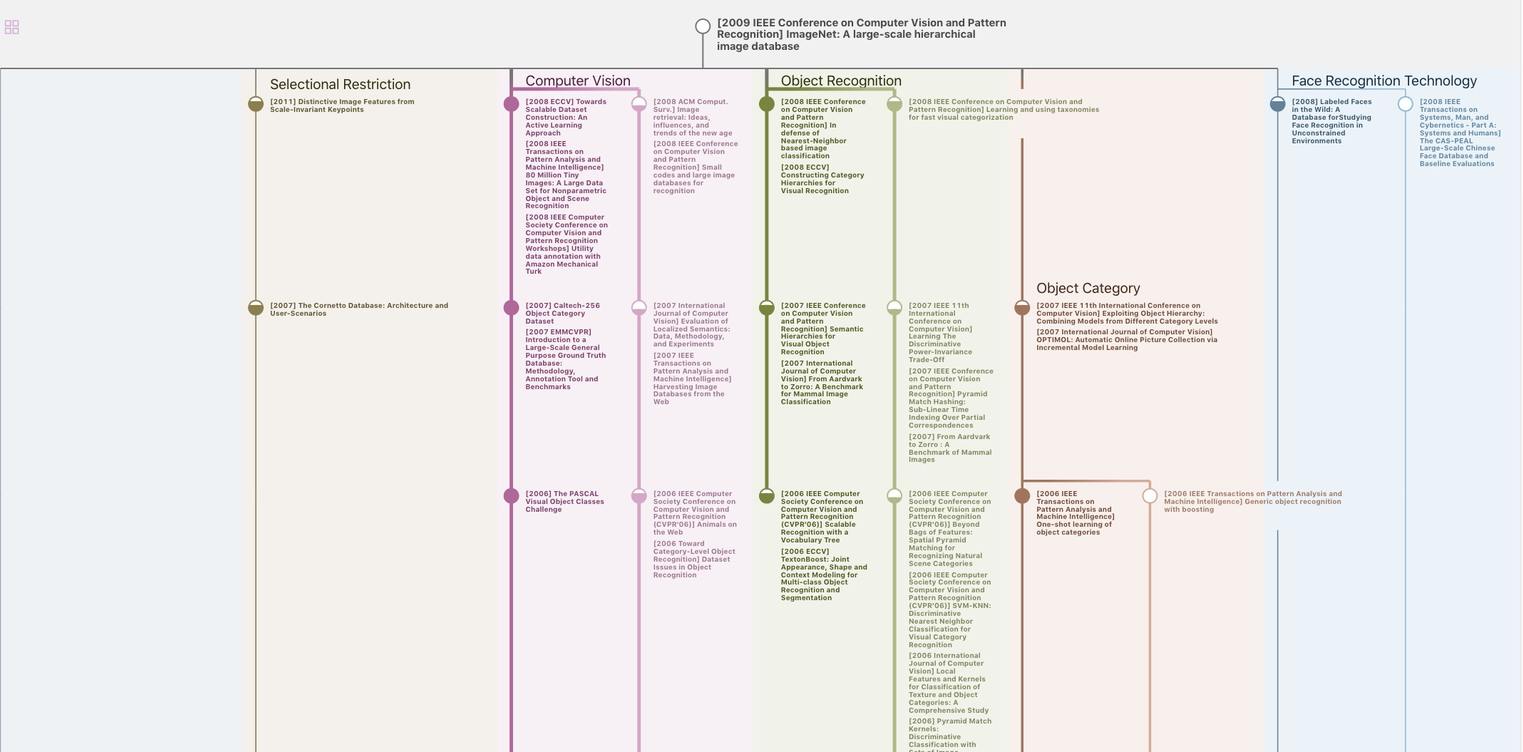
生成溯源树,研究论文发展脉络
Chat Paper
正在生成论文摘要