Image encoding selection based on Pearson correlation coefficient for time series anomaly detection
Alexandria Engineering Journal(2023)
摘要
Recently, anomaly detection in time-series data has received great attention from researchers due to its importance in problem-solving and predicting future system events. The majority of researchers combine convolutional neural networks (CNN) with image-encoding methods, including Gramian Angular Field (GAF) and Markov Transition Field (MTF). The selection of the right image-encoding method typically involves an iterative evaluation process that requires extensive computational time. This study proposes descriptive statistical analysis and Pearson correlation to find the correlation between statistical features in the encoded data from each image encoding and CNN accuracy. This information is essential for determining the image encoding method without image conversion processes and CNN trials, thus accelerating the computational time. The method tested on the initial dataset showed that the standard-deviation difference has the highest correlation with CNN accuracy (with a Pearson correlation coefficient of r = 0.944), followed by the mean difference (r = 0.705) and the count difference (r = 0.455), also resulting in a 95.35 % reduction in the computation time. The validity of this result was confirmed through the evaluation of several new datasets with different anomaly patterns, making this proposed approach suitable for selecting an appropriate image-encoding method.
更多查看译文
关键词
Time series,Anomaly detection,Image-encoding,Convolutional neural network,Pearson correlation
AI 理解论文
溯源树
样例
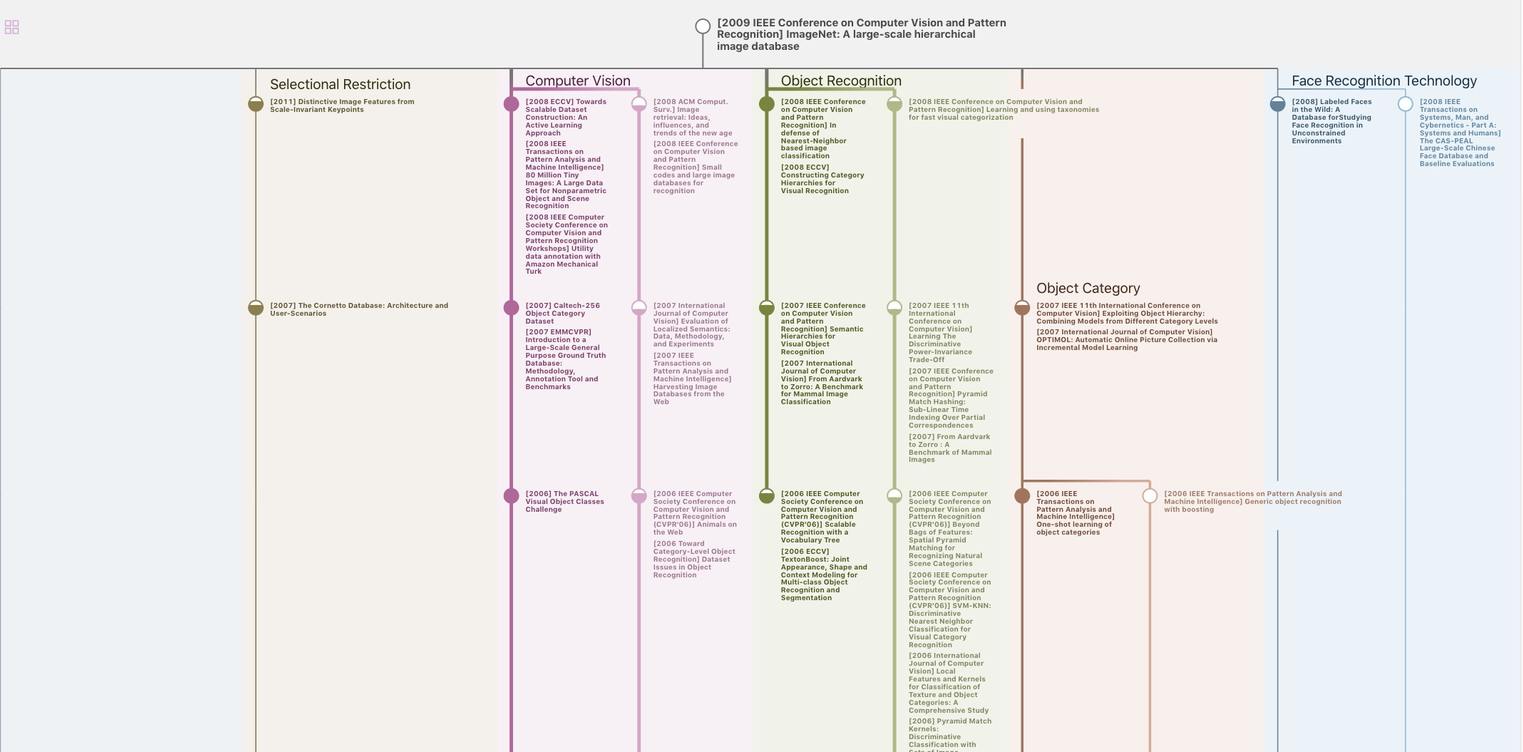
生成溯源树,研究论文发展脉络
Chat Paper
正在生成论文摘要