Boosting Adversarial Transferability via Gradient Relevance Attack.
ICCV(2023)
摘要
Plentiful adversarial attack researches have revealed the fragility of deep neural networks (DNNs), where the imperceptible perturbations can cause drastic changes in the output. Among the diverse types of attack methods, gradient-based attacks are powerful and easy to implement, arousing wide concern for the security problem of DNNs. However, under the black-box setting, the existing gradient-based attacks have much trouble in breaking through DNN models with defense technologies, especially those adversarially trained models. To make adversarial examples more transferable, in this paper, we explore the fluctuation phenomenon on the plus-minus sign of the adversarial perturbations’ pixels during the generation of adversarial examples, and propose an ingenious Gradient Relevance Attack (GRA). Specifically, two gradient relevance frameworks are presented to better utilize the information in the neighbor-hood of the input, which can correct the update direction adaptively. Then we adjust the update step at each iteration with a decay indicator to counter the fluctuation. Experiment results on a subset of the ILSVRC 2012 validation set forcefully verify the effectiveness of GRA. Furthermore, the attack success rates of 68.7% and 64.8% on Tencent Cloud and Baidu AI Cloud further indicate that GRA can craft adversarial examples with the ability to transfer across both datasets and model architectures. Code is released at https://github.com/RYC-98/GRA.
更多查看译文
AI 理解论文
溯源树
样例
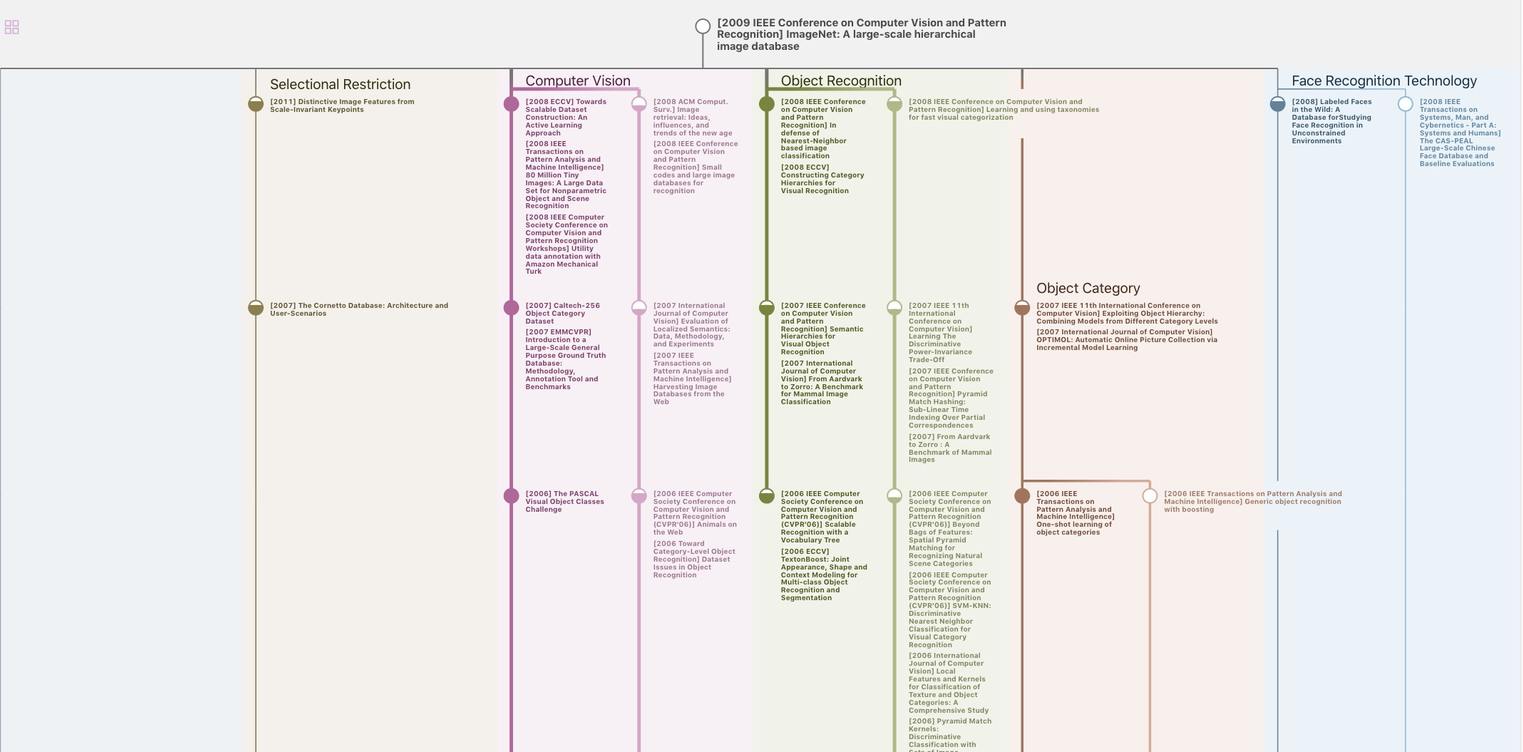
生成溯源树,研究论文发展脉络
Chat Paper
正在生成论文摘要