Hyperbolic Chamfer Distance for Point Cloud Completion.
Proceedings of the IEEE/CVF International Conference on Computer Vision (ICCV)(2023)
摘要
Chamfer distance (CD) is a standard metric to measure the shape dissimilarity between point clouds in point cloud completion, as well as a loss function for (deep) learning. However, it is well known that CD is vulnerable to outliers, leading to the drift towards suboptimal models. In contrast to the literature where most works address such issues in Euclidean space, we propose an extremely simple yet powerful metric for point cloud completion, namely Hyperbolic Chamfer Distance (HyperCD), that computes CD in hyperbolic space. In backpropagation, HyperCD consistently assigns higher weights to the matched point pairs with smaller Euclidean distances. In this way, good point matches are likely to be preserved while bad matches can be updated gradually, leading to better completion results. We demonstrate state-of-the-art performance on the benchmark datasets, i.e. PCN, ShapeNet-55, and ShapeNet34, and show from visualization that HyperCD can significantly improve the surface smoothness. Code is available at: https://github.com/Zhang-VISLab.
更多查看译文
AI 理解论文
溯源树
样例
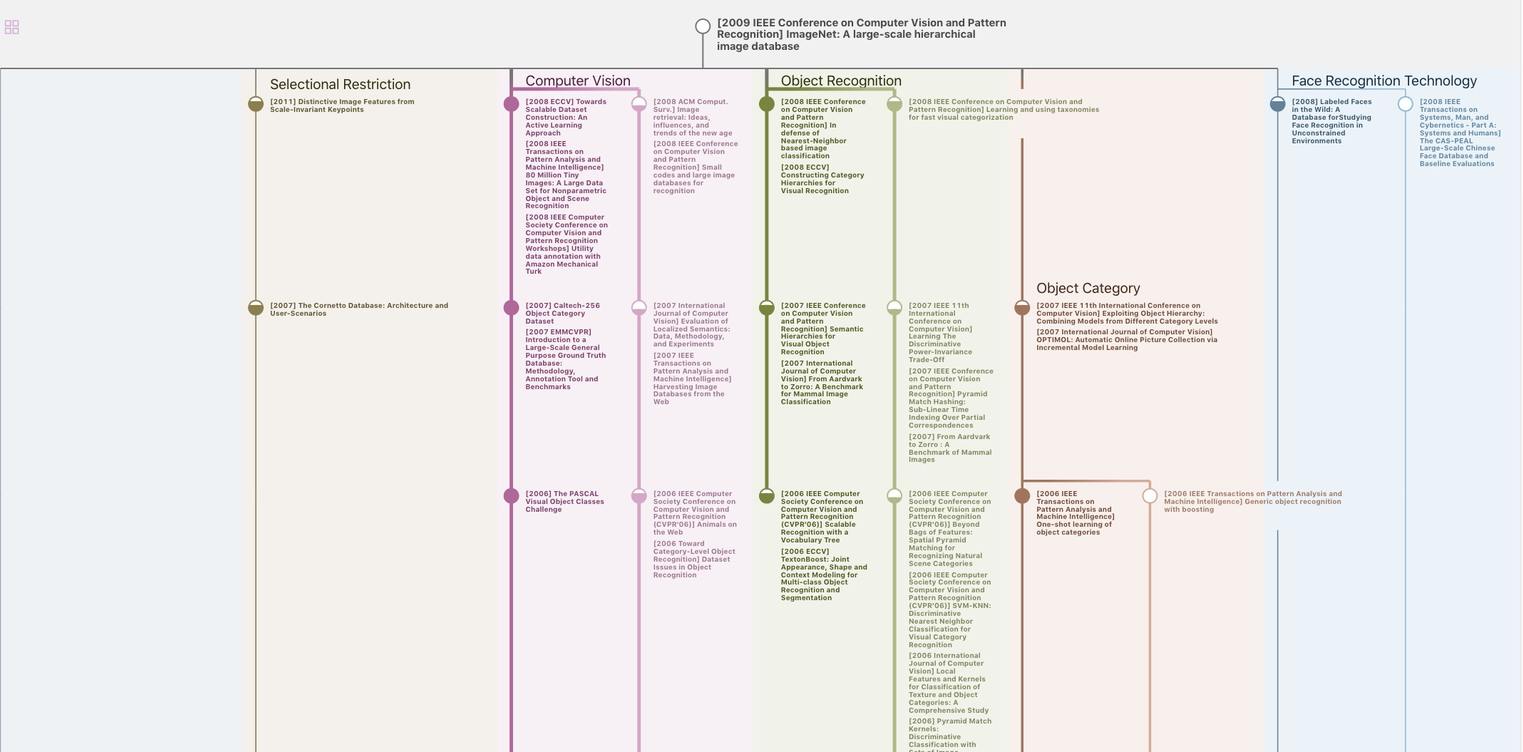
生成溯源树,研究论文发展脉络
Chat Paper
正在生成论文摘要