Workie-Talkie: Accelerating Federated Learning by Overlapping Computing and Communications via Contrastive Regularization.
Proceedings of the IEEE/CVF International Conference on Computer Vision (ICCV)(2023)
摘要
Federated learning (FL) over mobile edge devices is a promising distributed learning paradigm for various mobile applications. However, practical deployment of FL over mobile devices is very challenging because (i) conventional FL incurs huge training latency for mobile edge devices due to interleaved local computing and communications of model updates, (ii) there are heterogeneous training data across mobile edge devices, and (iii) mobile edge devices have hardware heterogeneity in terms of computing and communication capabilities.To address aforementioned challenges, in this paper, we propose a novel "workie-talkie" FL scheme, which can accelerate FL’s training by overlapping local computing and wireless communications via contrastive regularization (FedCR). FedCR can reduce FL’s training latency and almost eliminate straggler issues since it buries/embeds the time consumption of communications into that of local training. To resolve the issue of model staleness and data heterogeneity co-existing, we introduce class-wise contrastive regularization to correct the local training in FedCR. Besides, we jointly exploit contrastive regularization and subnetworks to further extend our FedCR approach to accommodate edge devices with hardware heterogeneity. We deploy FedCR in our FL testbed and conduct extensive experiments. The results show that FedCR outperforms its status quo FL approaches on various datasets and models.
更多查看译文
AI 理解论文
溯源树
样例
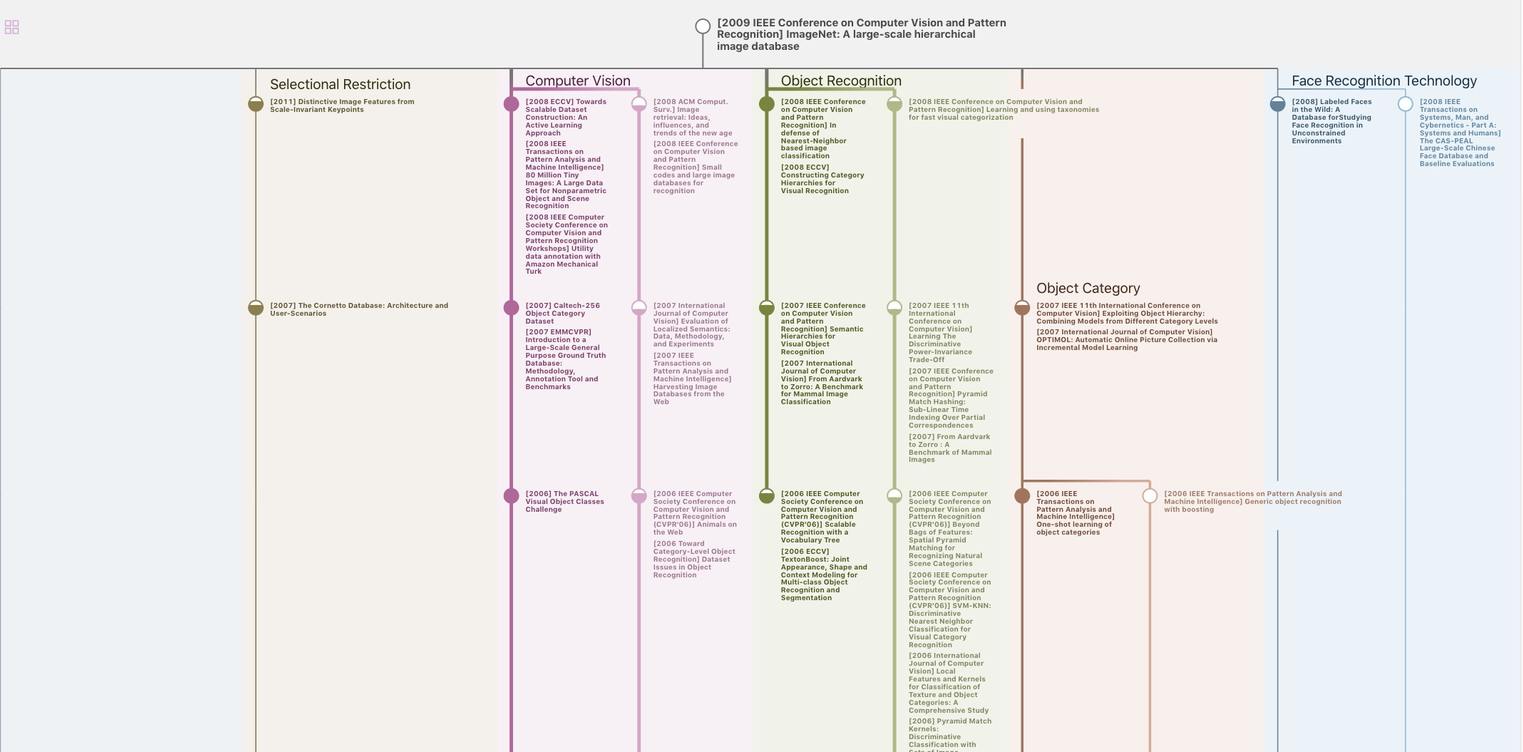
生成溯源树,研究论文发展脉络
Chat Paper
正在生成论文摘要