Accuracy of conventional fusion algorithms for precipitation estimates across the Chinese mainland
Journal of Hydroinformatics(2023)
摘要
Multi-source data-fusion approaches have been developed for estimating regional precipitation. However, studies considering the specific upper limits of the improved gridded rainfall data for different fusion approaches are limited. Here, the potential ranges of accuracy improvement for satellite and reanalysis rainfall products were addressed using various machine learning fusion approaches, including multivariate linear regression (MLR), feedforward neural network (FNN), random forest (RF), and long short-term memory (LSTM), over the Chinese mainland. All four fusion methods reduce errors in the original precipitation products. The upper limits of accuracy improvement in terms of correlation coefficient (CC) and root mean square error (RMSE) were 30.65 and 15.27%, respectively. M-RF showed the best average CC (0.828) and RMSE (4.62 mm/day) in the four seasons. LSTM performed the best under light rainfall events, whereas MLR and RF exhibited better performance under moderate and heavy rainfall events, respectively. Overall, these results serve as a basis for the fusion approach and technique selection, based on the comprehensive validation in different climate zones, altitudes, and seasons over the Chinese mainland. HIGHLIGHTS Machine learning fusion approaches were used for precipitation product estimation.; Four different models were used: MLR, feedforward neural network, RF, and LSTM.; All four fusion methods reduced errors in the original precipitation products.; LSTM showed the best performance for light rainfall events.; MLR and RF performed better for moderate and heavy rainfall events.;
更多查看译文
关键词
accuracy improvement,data fusion,machine learning,precipitation,rainfall information
AI 理解论文
溯源树
样例
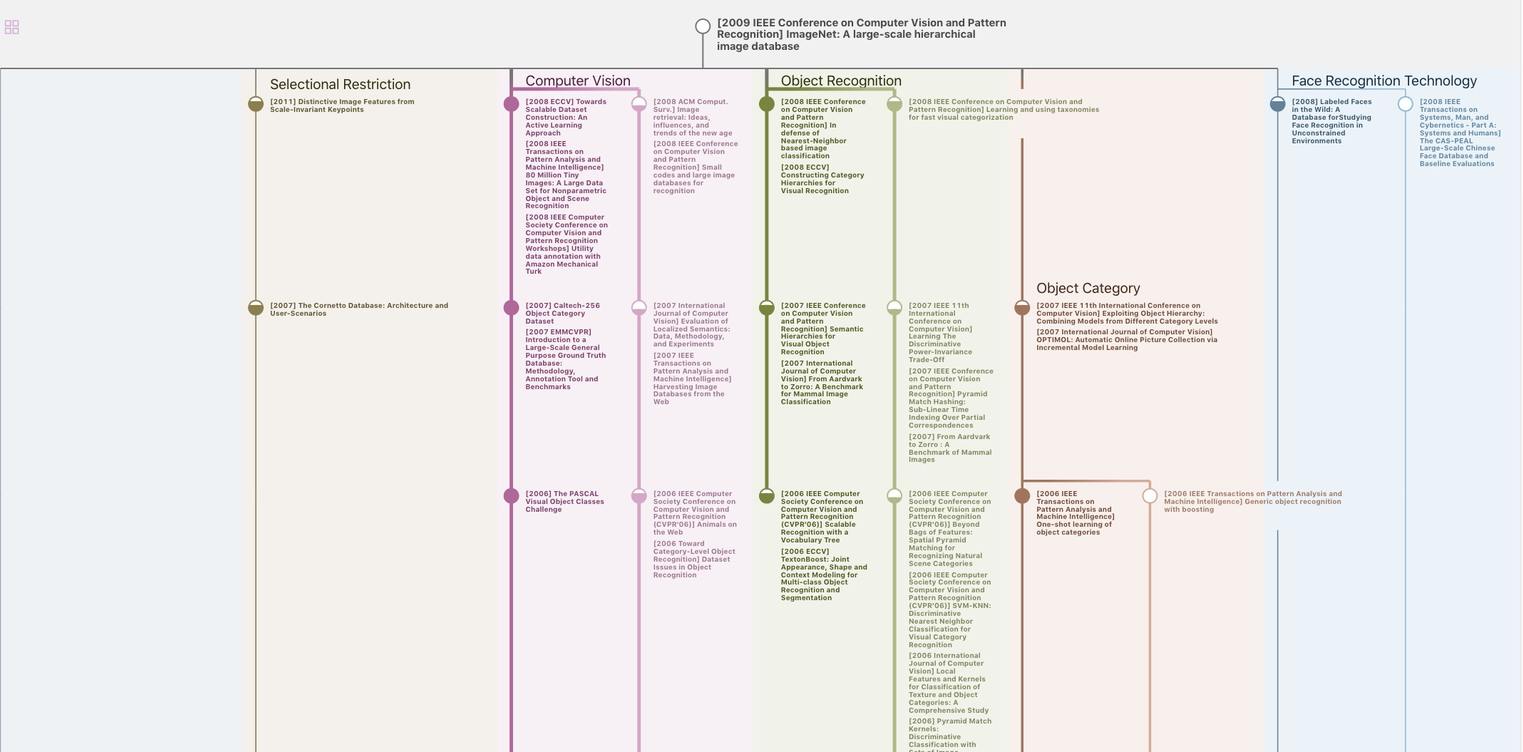
生成溯源树,研究论文发展脉络
Chat Paper
正在生成论文摘要