Towards Highly Compressed CNN Models for Human Activity Recognition in Wearable Devices
2023 Signal Processing: Algorithms, Architectures, Arrangements, and Applications (SPA)(2023)
摘要
The widespread usage of wearables set the foundations for many new applications that process the wearable sensor data. Human Activity Recognition (HAR) is a well-studied application that targets to classify the data captured by multiple wearable sensors into one of a predefined set of activity classes. Earlier approaches in the area relied on Machine Learning (ML) classifiers, while many researchers proposed solutions based on Deep Neural Networks (DNNs), e.g., using Long Short-Term Memory (LSTMs) or Convolution Neural Network (CNNs). Even though the usage of DNNs for HAR is a widely explored field, the problem of compressing (thus reducing the computational requirements) the resulting DNN models has been disregarded by the research community. This is of paramount importance if we consider that wearables are characterized by scarce memory and computational resources. In this work, we first analyze the impact of the size of the various CNN components (focusing on the convolution and dense layers) to the model validation performance. As a second step, we focus on two CNN models with diverse memory characteristics (the lean and the fat model) and we employ three different compression techniques (Filter-Based Pruning –FBP,– Low Rank Factorization –LRF,– and dynamic range quantization) assuming various compressibility scenarios.
更多查看译文
AI 理解论文
溯源树
样例
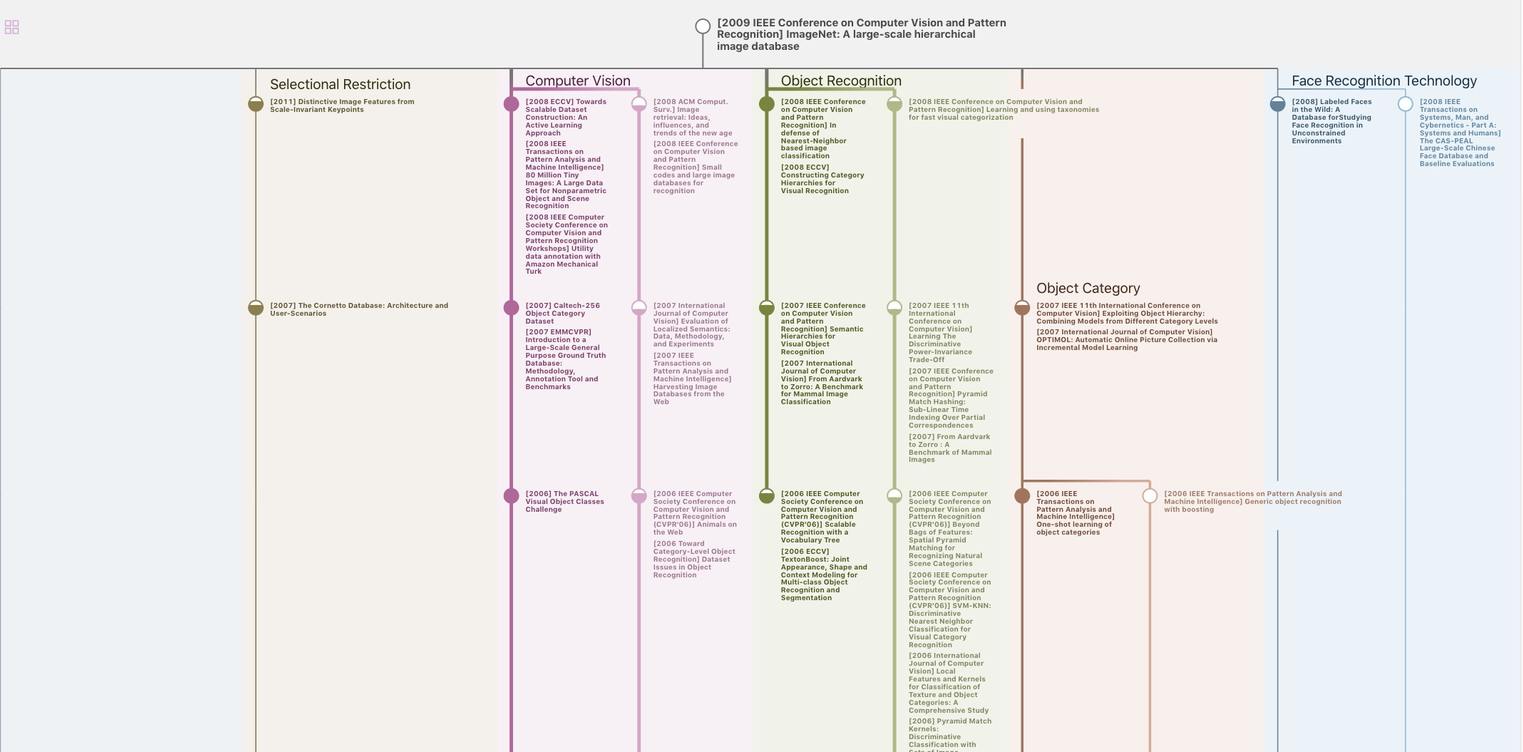
生成溯源树,研究论文发展脉络
Chat Paper
正在生成论文摘要