Error-Exponent of Distributed Hypothesis Testing for Gilbert-Elliot Source Models
2023 12th International Symposium on Topics in Coding (ISTC)(2023)
摘要
This paper considers Distributed Hypothesis Testing (DHT), in which a source X is encoded given that a side information Y is available only to the decoder. The decoder’s task is to make a decision between two hypothesis $\mathcal{H}_{0}$ and $\mathcal{H}_{1}$ related to the joint probability distribution of X and Y. While most works on DHT have adopted an information-theoretic approach by providing generic error exponents for the decision error, we focus on a specific and practical source model: the Gilbert-Elliot (GE) model. The later is a two-states hidden Markov model which describes time-varying correlation between X and Y. Starting from the generic error exponents, we develop a method for numerically estimating the error exponent for the GE model. We provide numerical results to evaluate the impact of the model parameters on the error exponents and explore the tradeoff between two types of error events, namely the testing error and the binning error.
更多查看译文
AI 理解论文
溯源树
样例
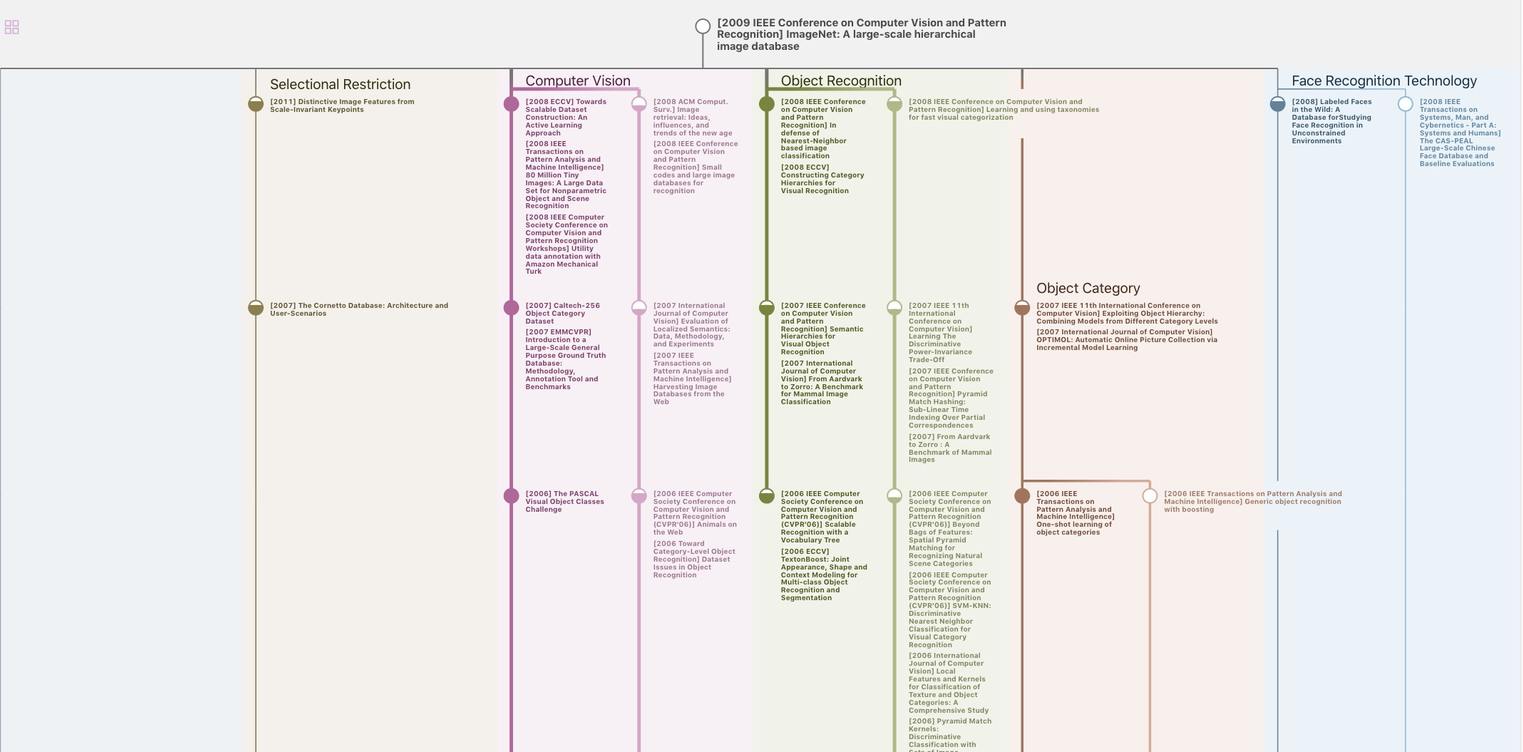
生成溯源树,研究论文发展脉络
Chat Paper
正在生成论文摘要