Investigating the Effect of Orientation Variability in Deep Learning-based Human Activity Recognition
UbiComp/ISWC '23 Adjunct: Adjunct Proceedings of the 2023 ACM International Joint Conference on Pervasive and Ubiquitous Computing & the 2023 ACM International Symposium on Wearable Computing(2023)
摘要
Deep Learning (DL) has enabled considerable increases in the accuracy of classification tasks in several domains, including Human Activity Recognition (HAR). It is well-known that when data distribution changes between the training and test datasets, the accuracy can drop, sometimes significantly. However, some variability sources in HAR, such as sensor orientation, are only sometimes considered when evaluating these models. Therefore, we must understand how much such changes could impact current DL architectures. In this paper, under an orientation variability scenario, we evaluate three common DL architectures, DeepConvLSTM, TinyHAR, and Attend-and-Discriminate, to quantify the performance drop attributed to this shift. Our results show that all architectures show performance drops on average, as expected, but participants are affected differently from them, so they would fall short for some in classification accuracy in real-life settings where orientation can change across the wearing sessions of one participant or across participants. The performance change is related to the difference in distribution distance.
更多查看译文
AI 理解论文
溯源树
样例
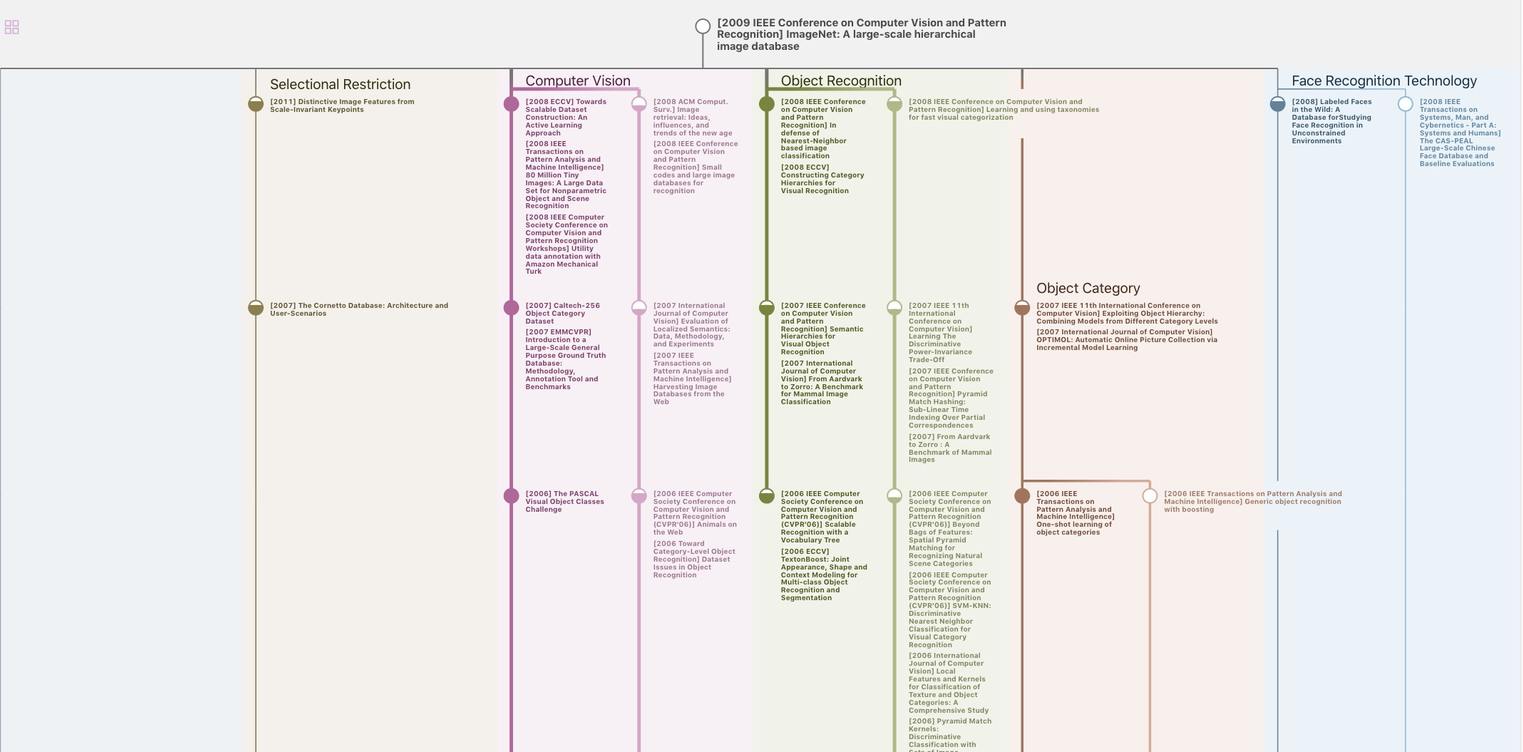
生成溯源树,研究论文发展脉络
Chat Paper
正在生成论文摘要