Privacy-aware Human Activity Recognition with Smart Glasses for Digital Therapeutics
UbiComp/ISWC '23 Adjunct: Adjunct Proceedings of the 2023 ACM International Joint Conference on Pervasive and Ubiquitous Computing & the 2023 ACM International Symposium on Wearable Computing(2023)
摘要
Human Activity Recognition (HAR) holds valuable potential as a digital biomarker, offering insights into individuals' physical activities and well-being. Leveraging wearable devices to monitor human activities can serve as an integral component of digital therapeutics, offering valuable insights into an individual's health and behavior. However, privacy concerns in HAR systems pose a significant challenge as users' data can be at risk of exposure and necessitates robust protection measures. To mitigate these concerns, the adoption of privacy-preserving techniques, such as federated learning, becomes necessary. This paper addresses the topic of Privacy-aware Human Activity Recognition (HAR) with Smart Glasses and explores a federated learning approach to achieve accurate recognition while preserving data privacy. The study highlights the trade-off between model complexity, recognition performance, and data privacy. Initially, a comparison was conducted between a complex deep learning network (STResNet) and a simpler deep learning network (DANA). The complex network exhibited an F1-Macro score of 0.87, while the simpler network achieved 0.82. Subsequently, the simpler network was further evaluated by comparing it to its federated learning implementation. The results revealed that the simpler network attained an F1-Macro score of 0.85, whereas federated learning achieved a slightly lower score of 0.80. These findings shed light on the performance disparities between the different approaches.
更多查看译文
AI 理解论文
溯源树
样例
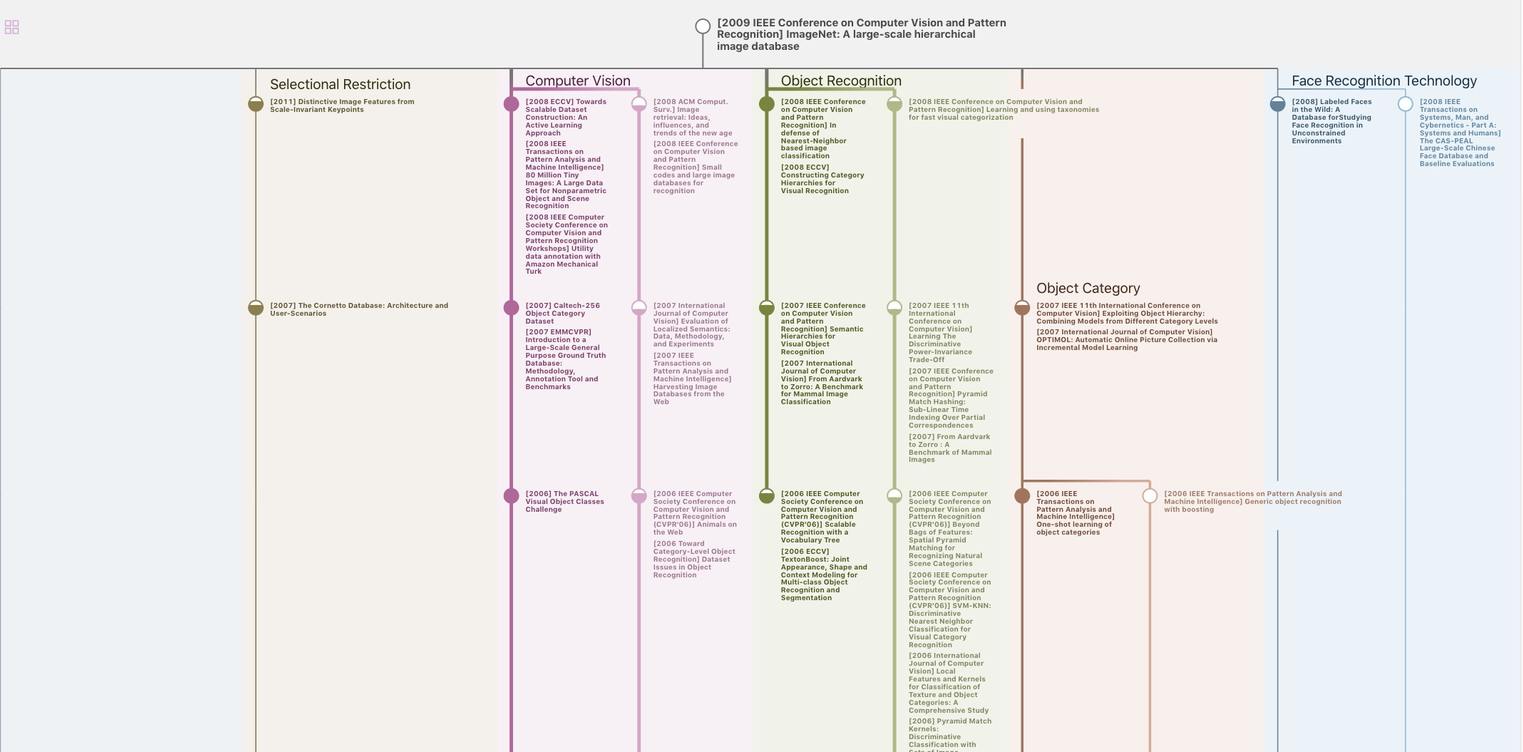
生成溯源树,研究论文发展脉络
Chat Paper
正在生成论文摘要