DFA-Net: Multi-Scale Dense Feature-Aware Network via Integrated Attention for Unmanned Aerial Vehicle Infrared and Visible Image Fusion
DRONES(2023)
摘要
Fusing infrared and visible images taken by an unmanned aerial vehicle (UAV) is a challenging task, since infrared images distinguish the target from the background by the difference in infrared radiation, while the low resolution also produces a less pronounced effect. Conversely, the visible light spectrum has a high spatial resolution and rich texture; however, it is easily affected by harsh weather conditions like low light. Therefore, the fusion of infrared and visible light has the potential to provide complementary advantages. In this paper, we propose a multi-scale dense feature-aware network via integrated attention for infrared and visible image fusion, namely DFA-Net. Firstly, we construct a dual-channel encoder to extract the deep features of infrared and visible images. Secondly, we adopt a nested decoder to adequately integrate the features of various scales of the encoder so as to realize the multi-scale feature representation of visible image detail texture and infrared image salient target. Then, we present a feature-aware network via integrated attention to further fuse the feature information of different scales, which can focus on specific advantage features of infrared and visible images. Finally, we use unsupervised gradient estimation and intensity loss to learn significant fusion features of infrared and visible images. In addition, our proposed DFA-Net approach addresses the challenges of fusing infrared and visible images captured by a UAV. The results show that DFA-Net achieved excellent image fusion performance in nine quantitative evaluation indexes under a low-light environment.
更多查看译文
关键词
infrared and visible fusion, unmanned aerial vehicles, image fusion, multi-scale feature, unsupervised gradient estimation
AI 理解论文
溯源树
样例
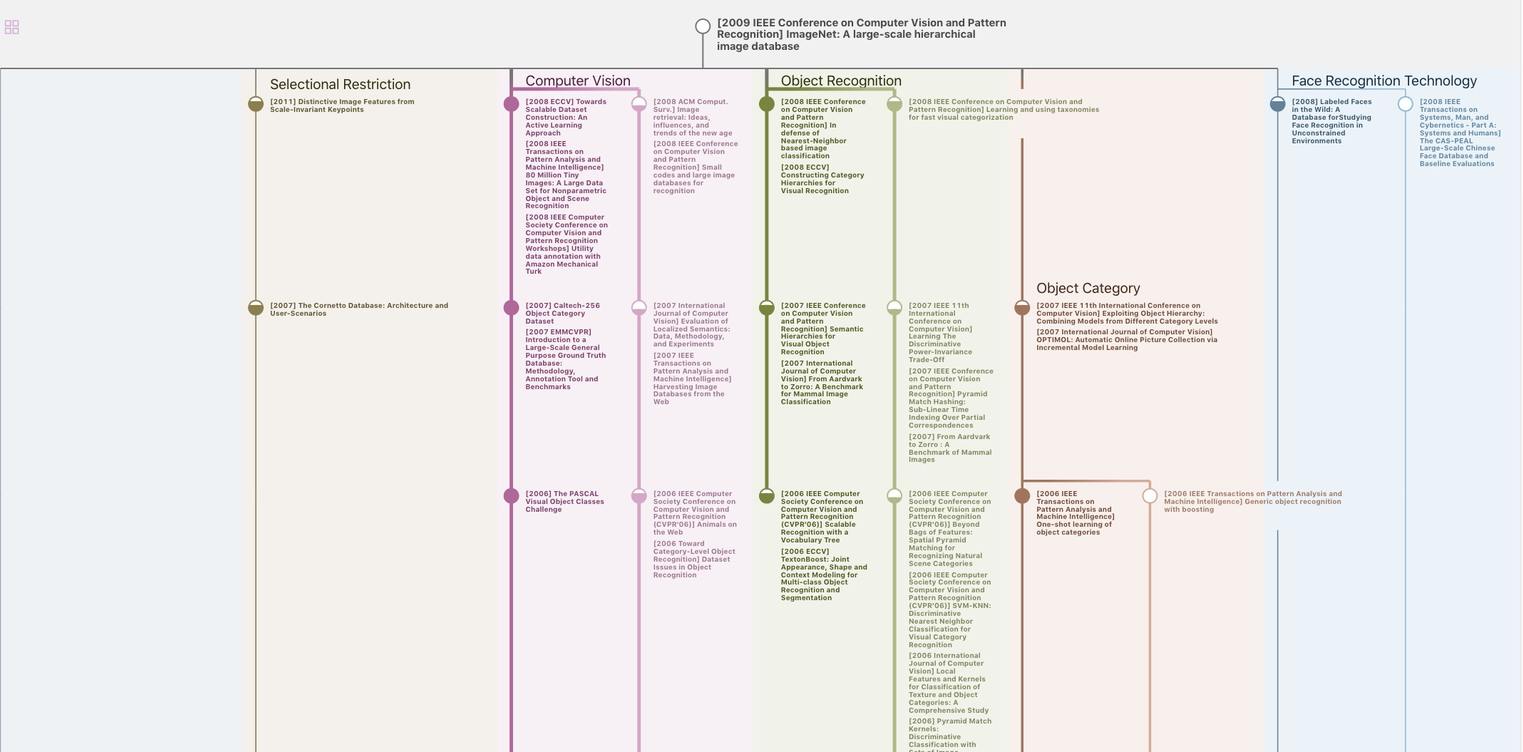
生成溯源树,研究论文发展脉络
Chat Paper
正在生成论文摘要