Addressing Bias in Fine-Grained Classification Datasets: A Strategy for Reliable Evaluation
2023 IEEE 13TH INTERNATIONAL CONFERENCE ON PATTERN RECOGNITION SYSTEMS, ICPRS(2023)
摘要
The high specificity of classes in fine-grained classification tasks leads to a small number of images per class in the common research datasets. Thus, the intra-class variance, such as differences in vehicle colors for fine-grained vehicle classification, may not be properly represented. Consequently, there can be a heavy bias in regards to certain attributes, such as vehicle colors, leading to unintended information leakage to the classifier which can relate the vehicle color to a vehicle model. This, in turn, can result in misleading accuracy estimations as we show in this study. To address this issue, we propose a method to quantify the bias of a train-test split in regard to a specific attribute, providing a metric for the expressiveness of the results. To prevent the bias from resulting in misleading accuracy estimations, we apply a simple splitting scheme that separates the manifestations of the attribute. This split prevents the model from exploiting features which are unrelated to the actual task at hand, leading to more accurate estimations of the model's real-world performance and generalization ability. We demonstrate the effectiveness of our method by examining the vehicle color bias in fine-grained vehicle classification datasets. Our results show that the strong performance of current methods, which render this task as practically solved, is largely due to the exploitation of this bias. We can cope with it to some degree by transforming the images to grayscale, partly restoring the performance of the original split. However, the accuracies are still far lower than indicated by the original split. Additionally, we demonstrate that the original random train-test splits of datasets may show higher accuracies for poorlier generalizing methods, which renders experimentation to find better methods misleading. Therefore, better splitting schemes, such as our attribute-based splitting scheme, are required to obtain trustworthy results in experiments.
更多查看译文
关键词
Fine-grained classification, vehicle make and model recognition, dataset bias, deep learning
AI 理解论文
溯源树
样例
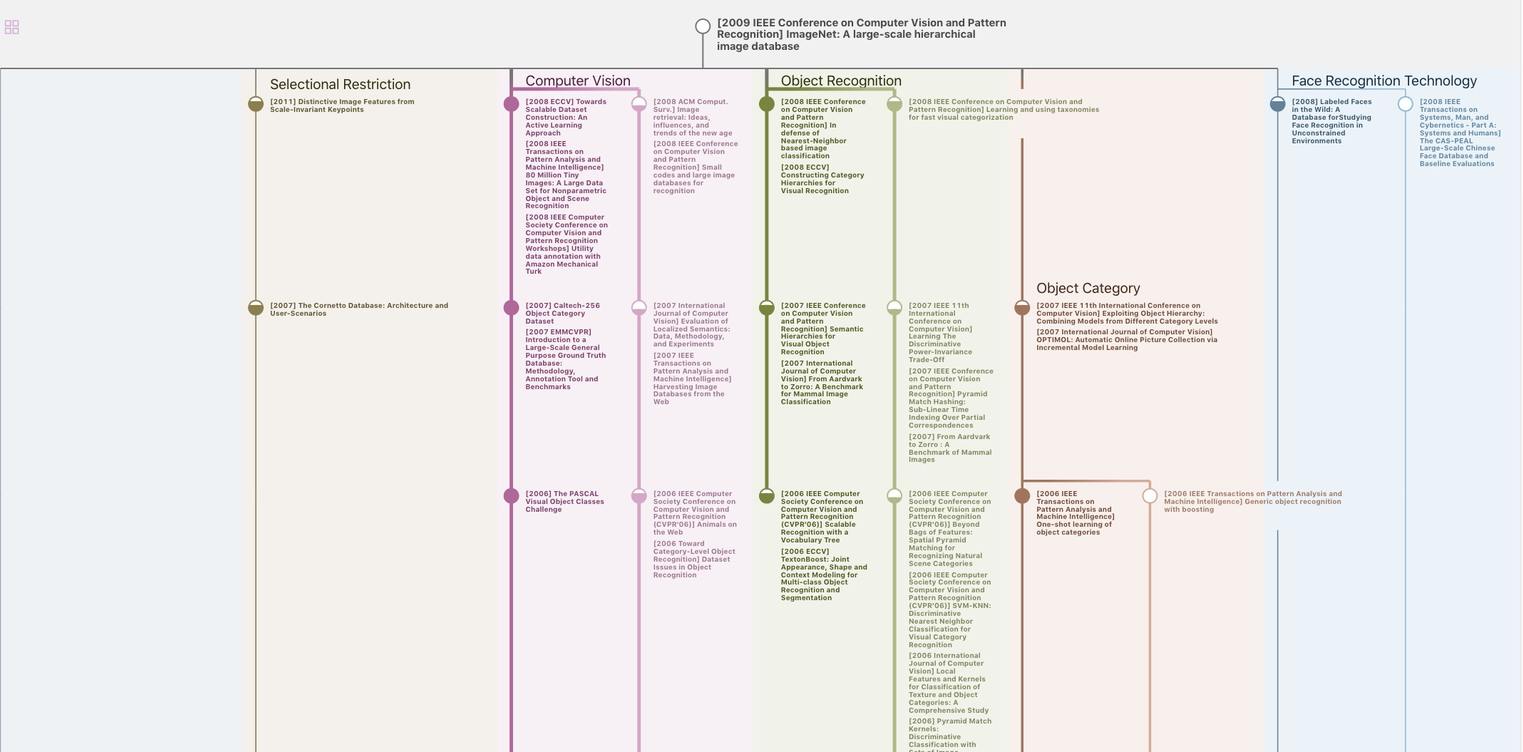
生成溯源树,研究论文发展脉络
Chat Paper
正在生成论文摘要