Graph-coupled time interval network for sequential recommendation
Inf. Sci.(2023)
摘要
Modeling the dynamics of sequential patterns (i.e., sequential recommendation) has obtained great attention, where the key problem is how to infer the next interesting item according to users' historical actions. Owing to high efficiency and accuracy, several Transformer-like frameworks have successfully achieved this task without adopting complicated recurrent or convolutional operations. Nevertheless, they focus only on the user-item bipartite graph and forgo other auxiliary information, which is non-trivial to attain satisfactory performance especially under long-tail distribution scenarios. In modeling short-term user interests, they fail to capture the time intervals between the recent actions and the target timestamp, which may result in the suboptimal performance. To settle such two problems, we propose a novel architecture for the task of sequential recommendation, namely graph-coupled time interval network (GCTN). Specifically, by means of item category information, we devise a category-aware graph propagation module to better learn user and item embeddings. Furthermore, we design a time-aware self-attention mechanism, which explicitly captures the effect of the time interval between two actions for next item prediction. To integrate these two parts into an organic whole, we introduce a personalized gating strategy to differentiate the importance of each part under the special context. Extensive experiments demonstrate the effectiveness and efficiency of GCTN over recent state-of-the-art methods on four real-world datasets, seamlessly combining the advantages of graph neural networks and Transformers.
更多查看译文
关键词
Sequential recommendation,Graph neural network,Time interval,Self-attention mechanism
AI 理解论文
溯源树
样例
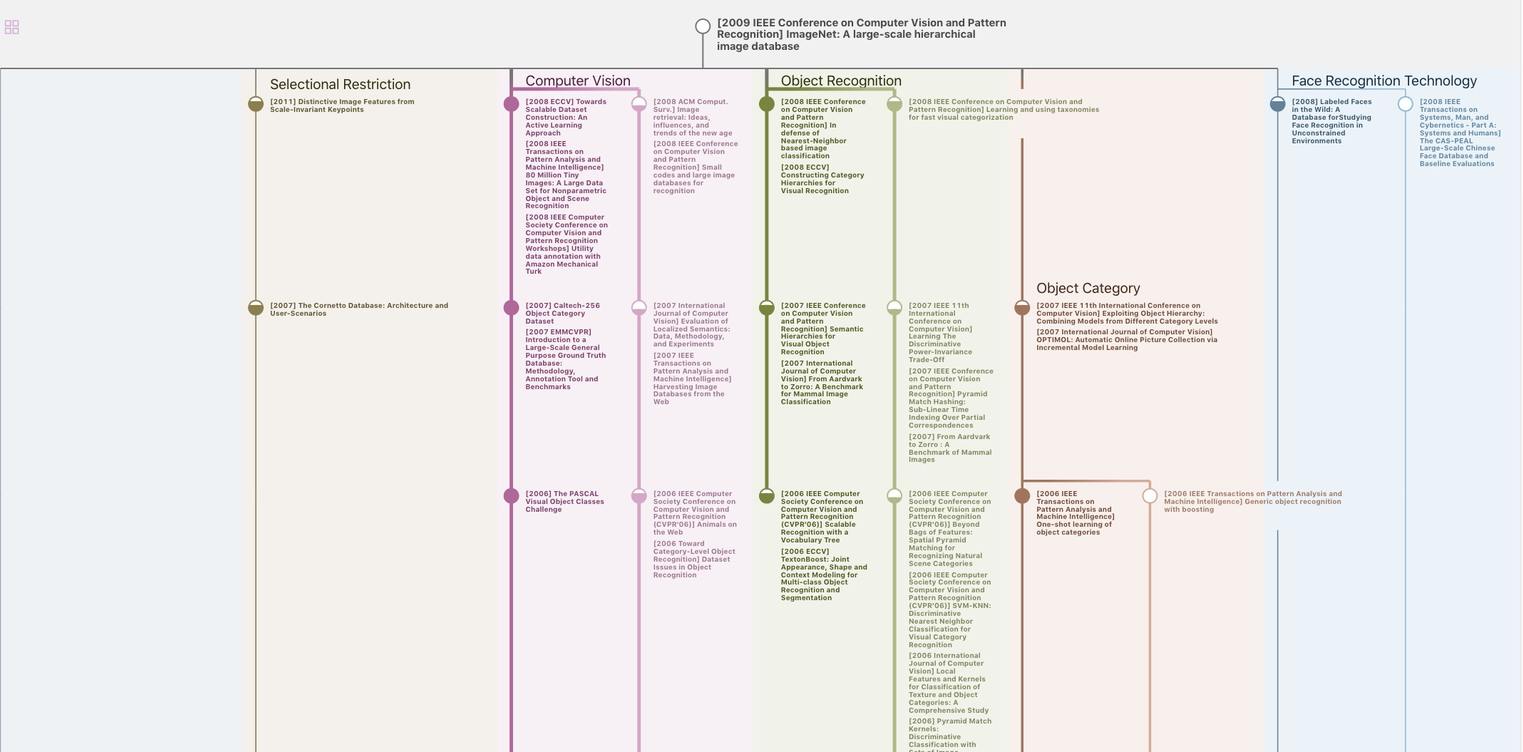
生成溯源树,研究论文发展脉络
Chat Paper
正在生成论文摘要