MSFF-CDNet: A Multiscale Feature Fusion Change Detection Network for Bi-Temporal High-Resolution Remote Sensing Image.
IEEE Geosci. Remote. Sens. Lett.(2023)
摘要
Change detection (CD) is an important application of remote sensing (RS) technology, which discovers changes by observing bi-temporal RS images. The rise of deep learning provides new solutions for CD. However, due to the insufficient extraction and utilization of deep features from RS images, existing deep learning-based CD methods are difficult to fully integrate such deep features, resulting in unstable performance, especially low sensitivity to multiscale changes. In this letter, a multiscale feature fusion CD network (MSFF-CDNet) is proposed to enhance feature fusion, by integrating a mask-guided change fusion module (MGCF) to achieve the fusion of the consistency and difference of multiscale features. Also, a CD refinement module (CDRM) is implemented to assist the encoding-decoding structure to achieve CD at a finer scale. By training with a hybrid loss function, the MSFF-CDNet is able to learn transformation relationships of bi-temporal RS images and their change maps. Besides, using a deep supervised (DS) learning strategy further improves the fitting performance and robustness. The method is experimented on two open-source datasets (i.e., CDD and LEVIR-CD datasets). Compared to state-of-the-art (SOTA) CD methods, the proposed method outperforms all metrics and its intersection over union (IoU) reaches 92.39% and 85.89%, respectively.
更多查看译文
关键词
remote sensing,fusion,detection,msff-cdnet,multi-scale,bi-temporal,high-resolution
AI 理解论文
溯源树
样例
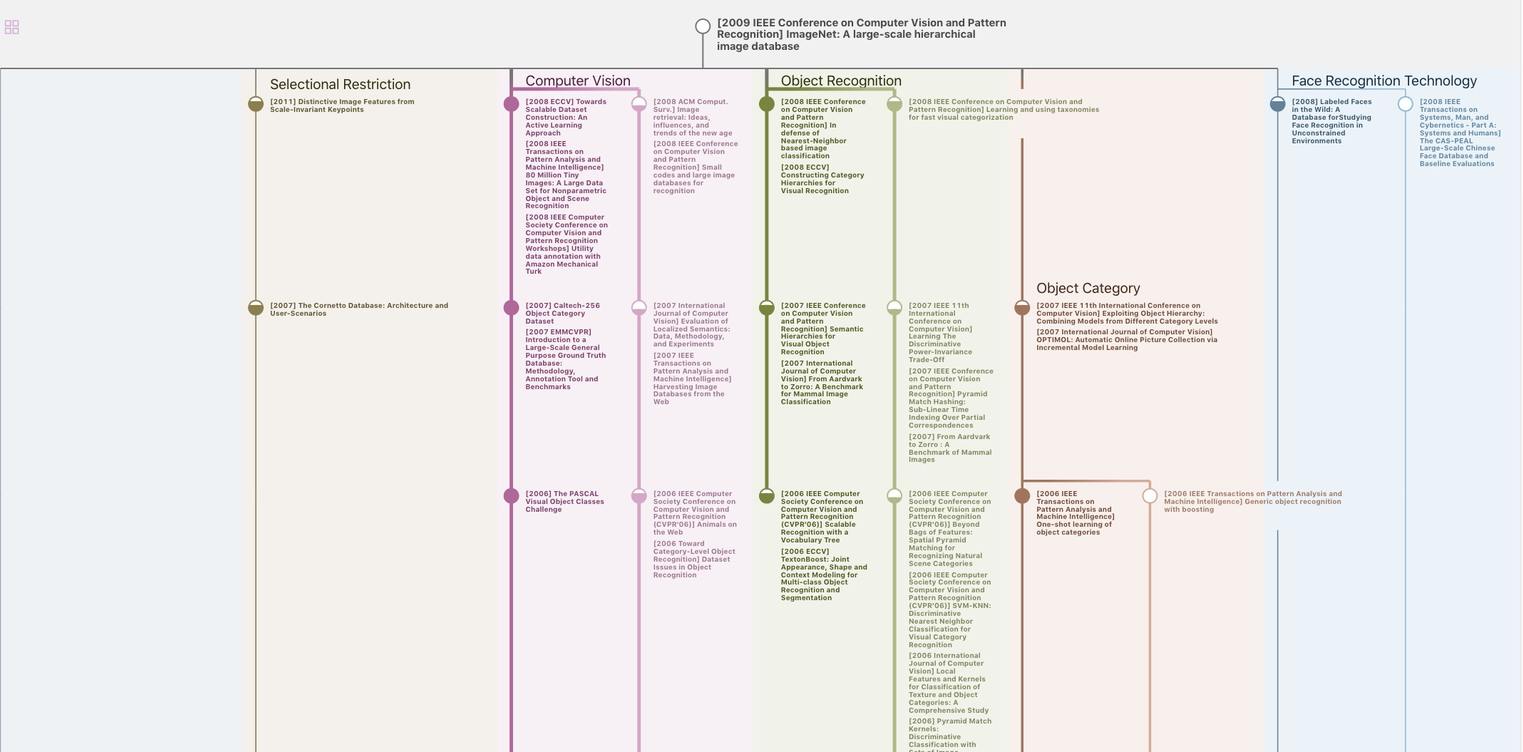
生成溯源树,研究论文发展脉络
Chat Paper
正在生成论文摘要