DMPH-Net: a deep multi-scale pyramid hybrid network for low-light image enhancement with attention mechanism and noise reduction
Signal Image Video Process.(2023)
摘要
iming at the problems of color bias and noise enhancement on the output image by the traditional low-light image enhancement algorithm, we propose a deep multi-scale pyramid hybrid network (DMPH-Net) algorithm that fuses attention mechanism and multi-scale pyramid is proposed in this paper. The algorithm uses DecomNet to decompose reflectance and light components, and uses multi-scale illumination attention module to fuse light and reflectance for the decomposed low-light reflectance to improve the realism and details of reflectance; by using five-layer feature pyramid and kernel selection in PRID-net module to achieve the fusion of contextual information between different scale feature layers, while effectively removing the enhanced of noise, and the added color loss effectively suppresses the color bias of the output image; using multi-scale cascading and channel attention mechanisms to adjust the illumination and fuse the illumination ratios, effectively enhancing the brightness, texture, and other feature information in the image. The DMPH-Net algorithm is experimentally validated on LOL and no-reference LIME, MEF, and NPE datasets, and the objective evaluation metrics PSNR ↑ , SSIM ↑ , LIPIPS ↓ , and NIQE ↓ are 23.3772, 0.8442, 0.1386, and 3.5966 on LOL dataset. The objective evaluation metrics NIQE on the reference-free datasets LIME, NPE, and MEF are 3.0735, 3.1711, and 2.9464, respectively. The experiments show that the DMPH-Net algorithm maintains high image details and textures in image enhancement and denoising, effectively enhances low-light images, and reduces noise and color bias of images. Compared with RUAS, UnRetinex-Net, and other enhancement algorithms, it improves in objective evaluation metrics PSNR, SSIM, LIPIPS, and NIQE.
更多查看译文
关键词
Low-light image enhancement,Retinex model,Pyramidal feature enhancement,Image denoising,Attention mechanism
AI 理解论文
溯源树
样例
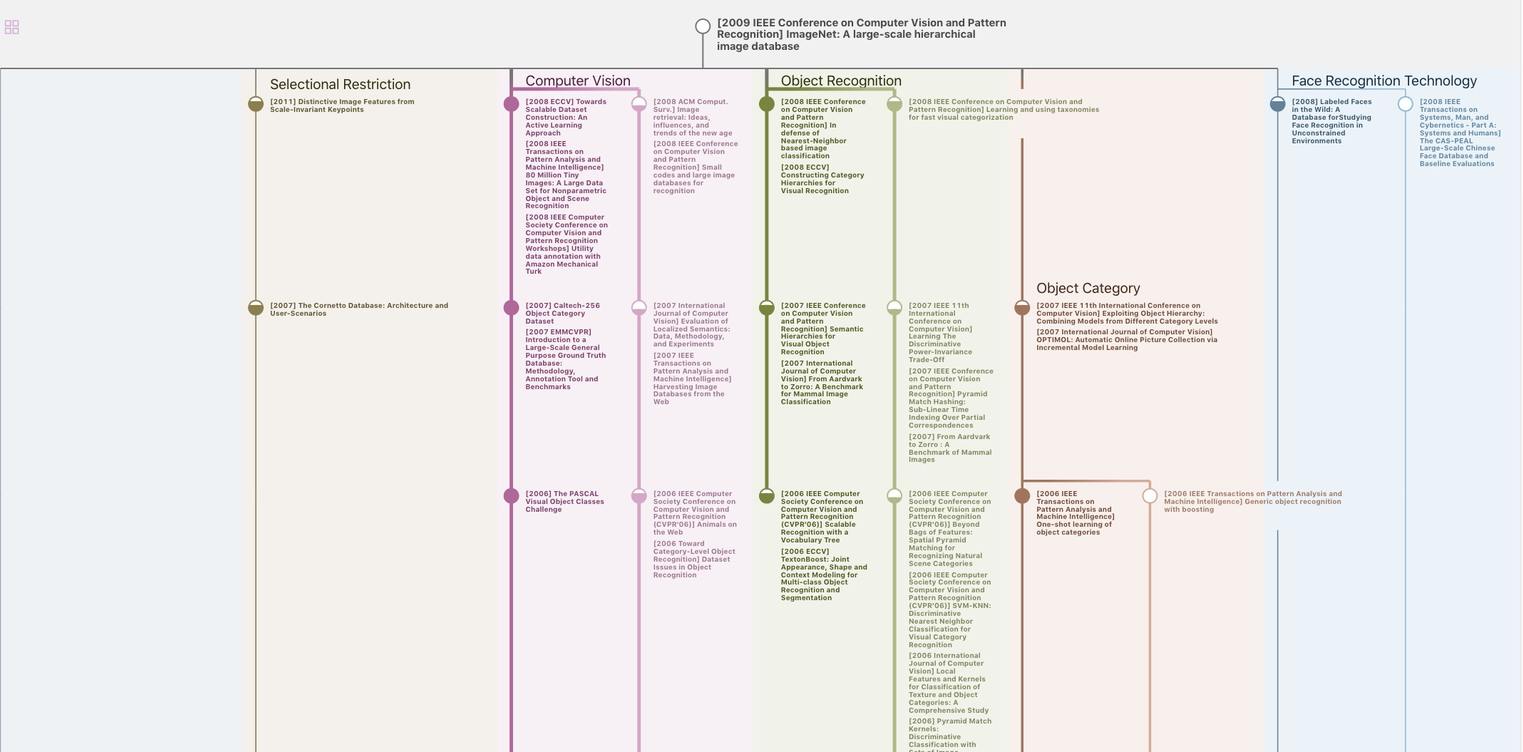
生成溯源树,研究论文发展脉络
Chat Paper
正在生成论文摘要