A weakly supervised anomaly detection method based on deep anomaly scoring network
Signal Image Video Process.(2023)
摘要
Recently most anomaly detection methods mainly use normal samples or unlabeled data for training. Due to the lack of prior anomaly knowledge, normal samples with noisy data are easy to be misjudged as anomalies. Therefore, this paper proposes a weakly supervised anomaly detection model based on a deep anomaly scoring network. In this model, ResNet is used as a feature extraction network, and the Res2Net module is added to ResNet, which extracts multi-scale features at a fine-grained level to improve the multi-scale representation ability of the network. At the same time, efficient channel attention is introduced to enhance feature extraction performance by allocating the attention to feature channels. In addition, the anomaly score network calculates the anomaly score directly according to the extracted feature representation and optimizes the anomaly score in an end-to-end way. Comprehensive experiments on the challenging MVTec AD, KolektorSDD and ELPV datasets show that compared with the current advanced anomaly detection methods, our model achieves better results in anomaly detection and location, and has better robustness.
更多查看译文
关键词
anomaly detection method,anomaly detection
AI 理解论文
溯源树
样例
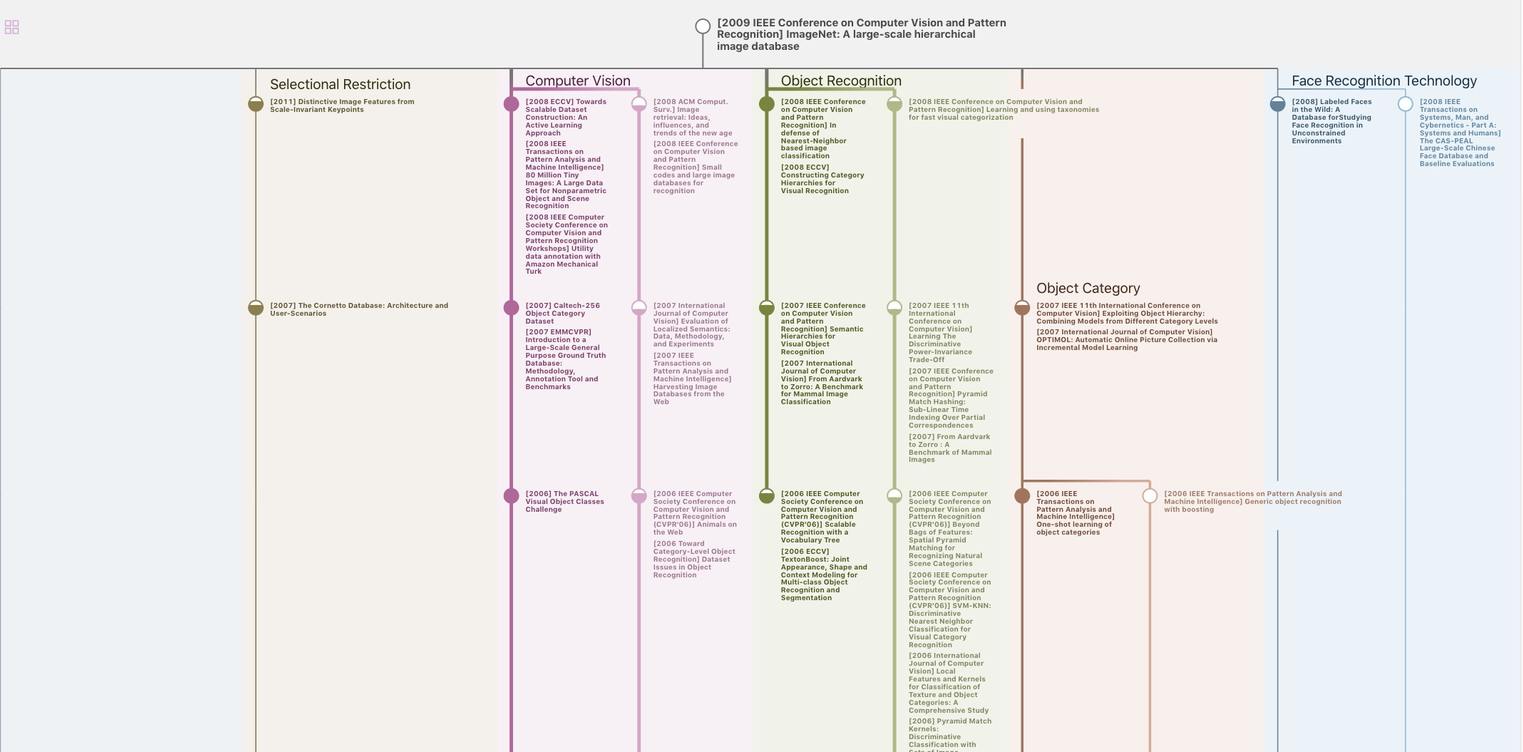
生成溯源树,研究论文发展脉络
Chat Paper
正在生成论文摘要