Probabilistic motion planning for non-Euclidean and multi-vehicle problems
Robotics Auton. Syst.(2023)
摘要
Trajectory planning tasks for non-holonomic or collaborative systems are naturally modeled by state spaces with non-Euclidean metrics. However, existing proofs of convergence for sample-based motion planners only consider the setting of Euclidean state spaces. We resolve this issue by formulating a flexible framework and set of assumptions for which the widely-used PRM*, RRT, and RRT* algorithms remain asymptotically optimal in the non-Euclidean setting. The framework is compatible with collaborative trajectory planning: given a fleet of robotic systems that individually satisfy our assumptions, we show that the corresponding collaborative system again satisfies the assumptions and therefore has guaranteed convergence for the trajectory-finding methods. Our joint state space construction builds in a coupling parameter 1 <= p <= 8, which interpolates between a preference for minimizing total energy at one extreme and a preference for minimizing the travel time at the opposite extreme. We illustrate our theory with trajectory planning for simple coupled systems, fleets of Reeds-Shepp vehicles, and a highly non-Euclidean fractal space. (c) 2023 Elsevier B.V. All rights reserved.
更多查看译文
关键词
Nonholonomic motion planning,Motion and trajectory planning,Trajectory planning for multiple mobile~robots,Cooperative robots and multi-robot & nbsp,systems
AI 理解论文
溯源树
样例
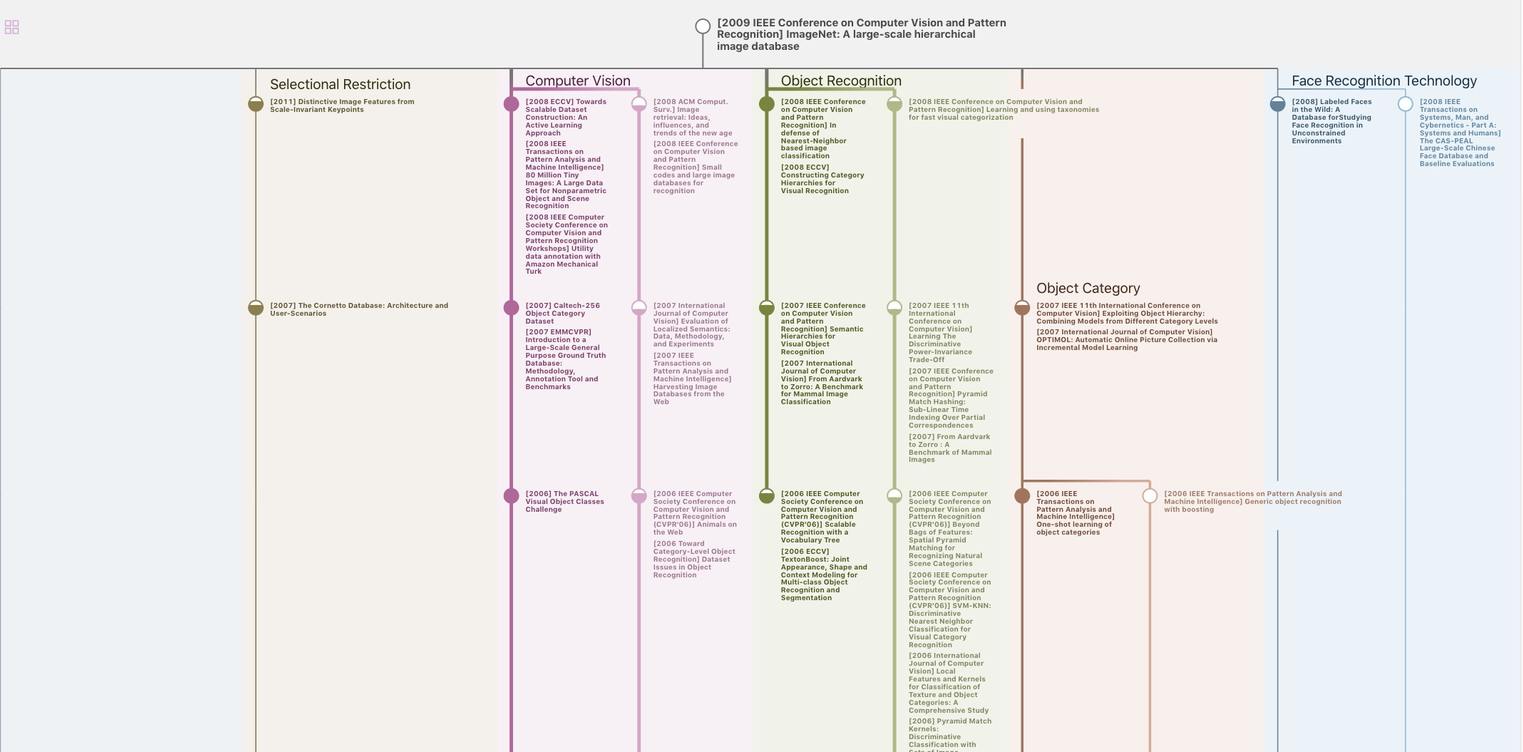
生成溯源树,研究论文发展脉络
Chat Paper
正在生成论文摘要