Super-Resolution Reconstruction for Stereoscopic Omnidirectional Display Systems via Dynamic Convolutions and Cross-View Transformer.
IEEE Trans. Instrum. Meas.(2023)
摘要
Stereoscopic omnidirectional images (SODIs) usually require recording very high-resolution (HR) information whereby it is beneficial to exploit a super-resolution (SR) scheme to super-resolve low-resolution (LR) SODIs. Compared with traditional 2-D SR approaches, the algorithms of SODI SR (SODI-SR) need to deal with two extra aspects: binocular information and panoramic characteristics. In this article, we first build a synthetic-specific SODI-SR dataset with LR-HR image pairs. Then, we propose a dynamic convolutions (Dconvs) and transformer network (Dconv-Trans-Net) for SODI-SR. Specifically, due to the nonuniform sampling of equirectangular projection (ERP), we deploy Dconvs with the structure of atrous spatial pyramid pooling (ASPP) to adaptively select content-aware and weight-aware kernels for patch-wise feature extraction. To capture diverse feature embeddings from left and right views, we utilize a symmetric bidirectional parallax attention module (biPAM) to extract local features along epipolar lines and propose cross-view transformer (CvTrans) to mine global contextual features apart from the epipolar line. Finally, quantitative and qualitative experiments demonstrate that our proposed approach outperforms the state-of-the-art (SOTA) panoramic or stereoscopic SR methods on the constructed SODI-SR dataset with two upscaling factors.
更多查看译文
关键词
Cross-view interactions,dynamic convolutions (Dconvs),stereoscopic omnidirectional images (SODIs),super-resolution (SR),transformer
AI 理解论文
溯源树
样例
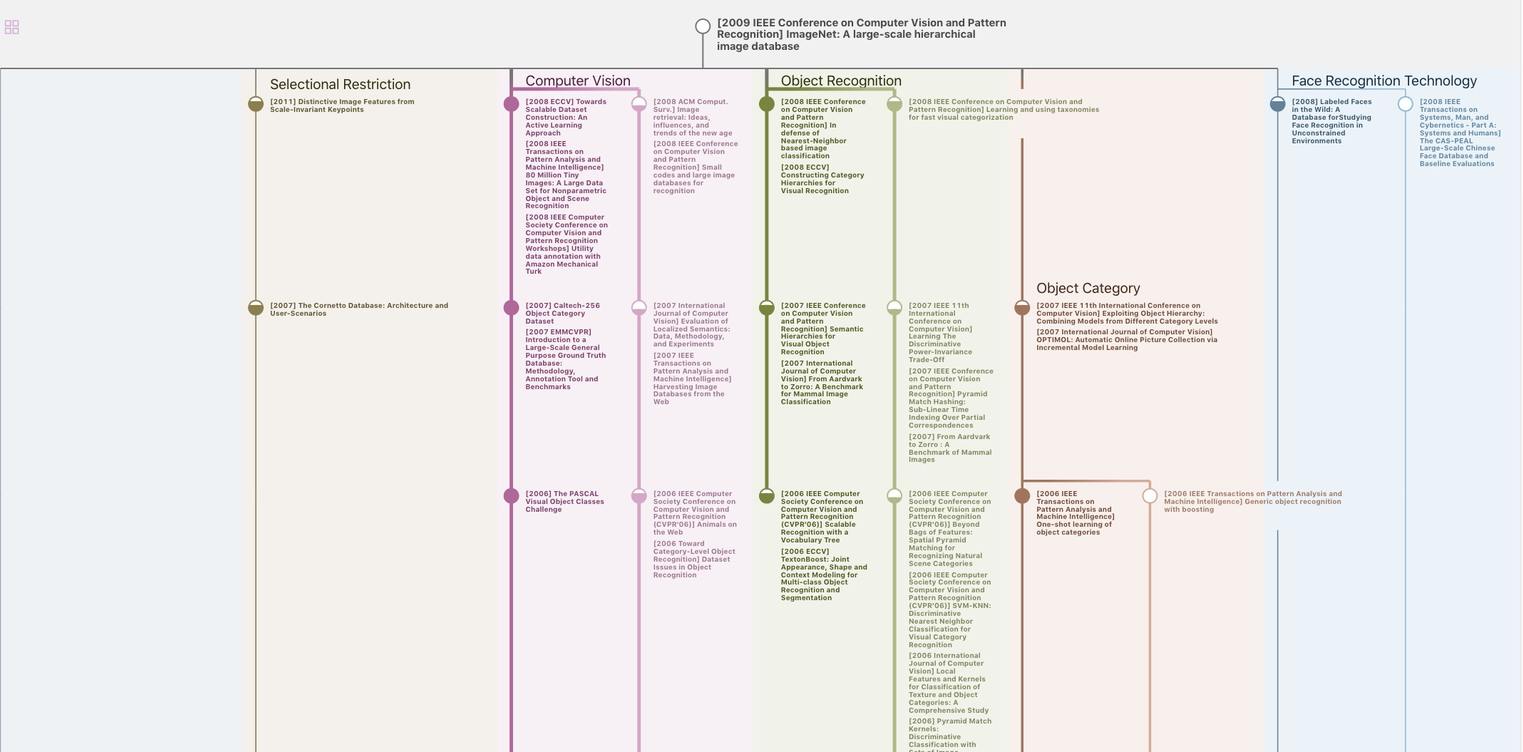
生成溯源树,研究论文发展脉络
Chat Paper
正在生成论文摘要