Anomaly-Based Intrusion on IoT Networks Using AIGAN-a Generative Adversarial Network.
IEEE Access(2023)
摘要
Adversarial attacks have threatened the credibility of machine learning models and cast doubts over the integrity of data. The attacks have created much harm in the fields of computer vision, and natural language processing. In this paper, we focus on the adversarial attack, in particular the poisoning attack, against the network intrusion detection system (NIDS), which is often viewed as the first line of defense against cyber threats. We develop a generative adversarial network (GAN) in AIGAN, which uses deep learning techniques to generate adversarial data and to conduct an anomaly attack on IoT networks. To evaluate the effectiveness of our generator, we measure the similarities between real and fake data using the Jaccard similarity index, in addition comparing the F1-scores from four generic algorithms: multilayer perception, logistic regression, decision tree, random forest. We contrast the performance of ten machine learning classifiers experimented on two real IoT datasets and their fake adversarial samples. Our work highlights a vulnerable side of NIDS created by machine learning when attacked with adversarial perturbation.
更多查看译文
关键词
iot networks,anomaly-based
AI 理解论文
溯源树
样例
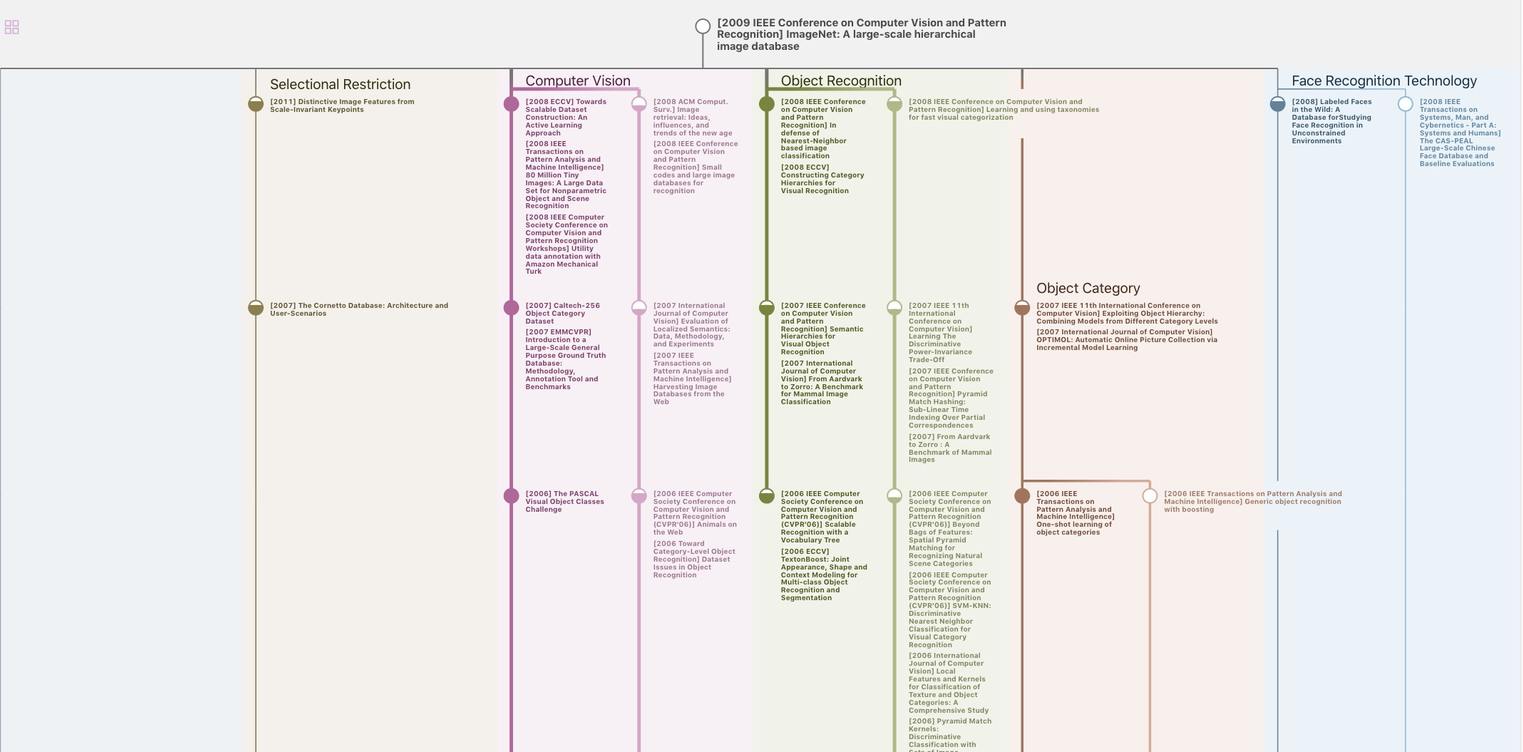
生成溯源树,研究论文发展脉络
Chat Paper
正在生成论文摘要