BC-PINN: an adaptive physics informed neural network based on biased multiobjective coevolutionary algorithm
Neural Comput. Appl.(2023)
摘要
Physics informed neural network (PINN) has become a promising method for solving partial differential equations (PDEs). The loss function of PINN is a weighted sum of multiple items. This makes it easy to fall into local optima, especially the gradient pathologies when solving high frequency problems. The value of penalty coefficients has a crucial impact on the prediction results. Therefore, a new PINN with adaptive penalty coefficients iteratively optimized by biased multiobjective coevolutionary algorithm (BC-PINN) is presented. In BC-PINN, a two-stage optimization mechanism is used to search for parameters of neural network and penalty coefficients respectively. This method involves constructing the fitness function of penalty coefficients based on the biased dominance ranking by data item and regularization item. Compared with the previous works of others, the accuracy of fitting the initial conditions and boundary conditions is considered to be given priority, which is more conducive to PINN converging to the particular solution of PDE. In addition, the set of penalty coefficients is divided into multiple populations to improve the optimization efficiency through coevolutionary algorithm. The empirical results show that: (1) Our method can improve the gradient pathologies and effectively capture the high-frequency features. (2) Compared to the original PINN, it reduces the MSE by 1–6 orders of magnitude in our benchmark functions.
更多查看译文
关键词
adaptive physics,neural network,bc-pinn
AI 理解论文
溯源树
样例
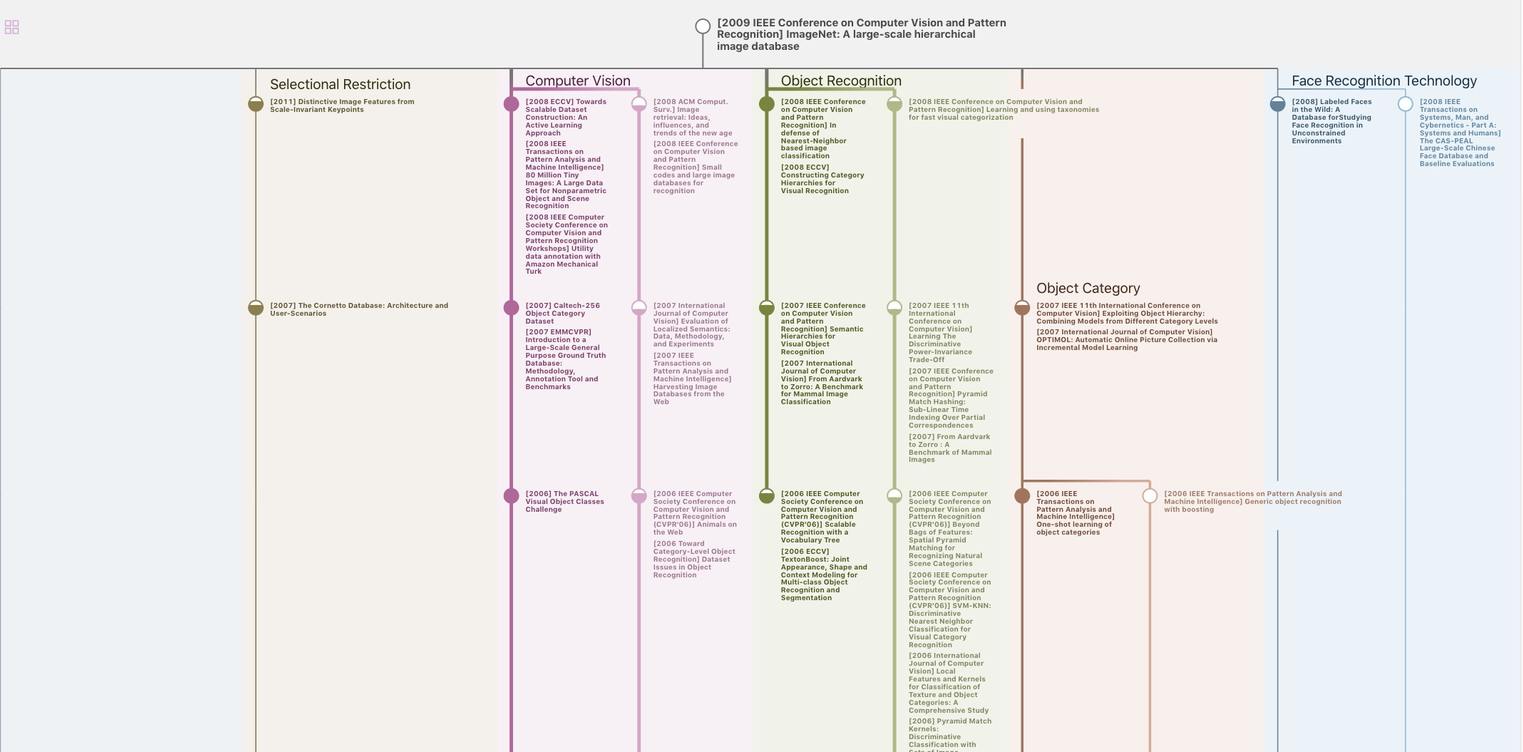
生成溯源树,研究论文发展脉络
Chat Paper
正在生成论文摘要