Node Importance Estimation with Multiview Contrastive Representation Learning.
Int. J. Intell. Syst.(2023)
摘要
Node importance estimation is a fundamental task in graph analysis, which can be applied to various downstream applications such as recommendation and resource allocation. However, existing studies merely work under a single view, which neglects the rich information hidden in other aspects of the graph. Hence, in this work, we propose a Multiview Contrastive Representation Learning (MCRL) model to obtain representations of nodes from multiple perspectives and then infer the node importance. Specifically, we are the first to apply the contrastive learning technique to the node importance analysis task, which enhances the expressiveness of graph representations and lays the foundation for importance estimation. Moreover, based on the improved representations, we generate the entity importance score by attentively aggregating the scores from two different views, i.e., node view and node-edge interaction view. We conduct extensive experiments on real-world datasets, and the experimental results show that MCRL outperforms existing methods on all evaluation metrics.
更多查看译文
关键词
multiview contrastive representation learning,importance
AI 理解论文
溯源树
样例
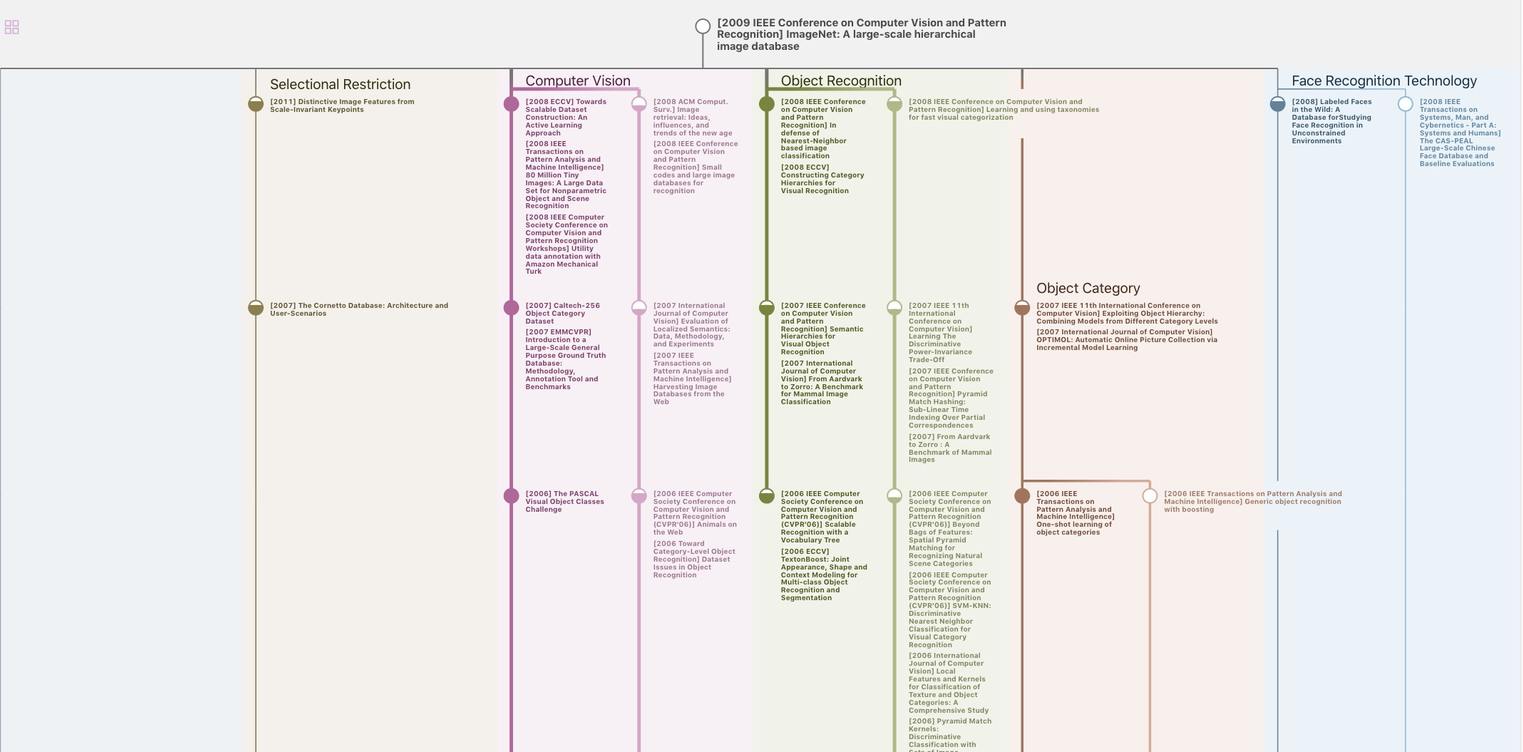
生成溯源树,研究论文发展脉络
Chat Paper
正在生成论文摘要