Robotic Grasp Pose Detection Method Based on Multiscale Features.
Int. J. Humanoid Robotics(2023)
摘要
A robotic grasp detection algorithm based on multiscale features is proposed for autonomous robotic grasping in an unstructured environment. The grasp detection model borrowed the YOLOv3 object detection algorithm and retained the original idea of multiscale detection to improve the perception ability of the grasp rectangle on different scales. Squeeze and excitation blocks were embedded into the Residual Networks (ResNet) structure of the original model, with deformable convolution (DC) introduced, so that the model attained stronger feature extraction ability to cope with more complex grasp detection tasks. Meanwhile, the prediction of the direction angle was transformed into a combination of classification and regression, achieving the prediction of the direction angle of the grabbing frame under different postures. The model was simulated on the Cornell grasp dataset. The results demonstrate that the algorithm in this study can effectively balance the accuracy and efficiency of detection and can migrate the prediction of the grasp rectangle to new objects. The results of online grasp experiments on a Baxter robot show that the average grasp success rate of 93% is achieved for 10 different objects, demonstrating the practical feasibility of the algorithm.
更多查看译文
关键词
Deep learning, grasp detection, multiscale feature, unstructured environment, YOLOv3
AI 理解论文
溯源树
样例
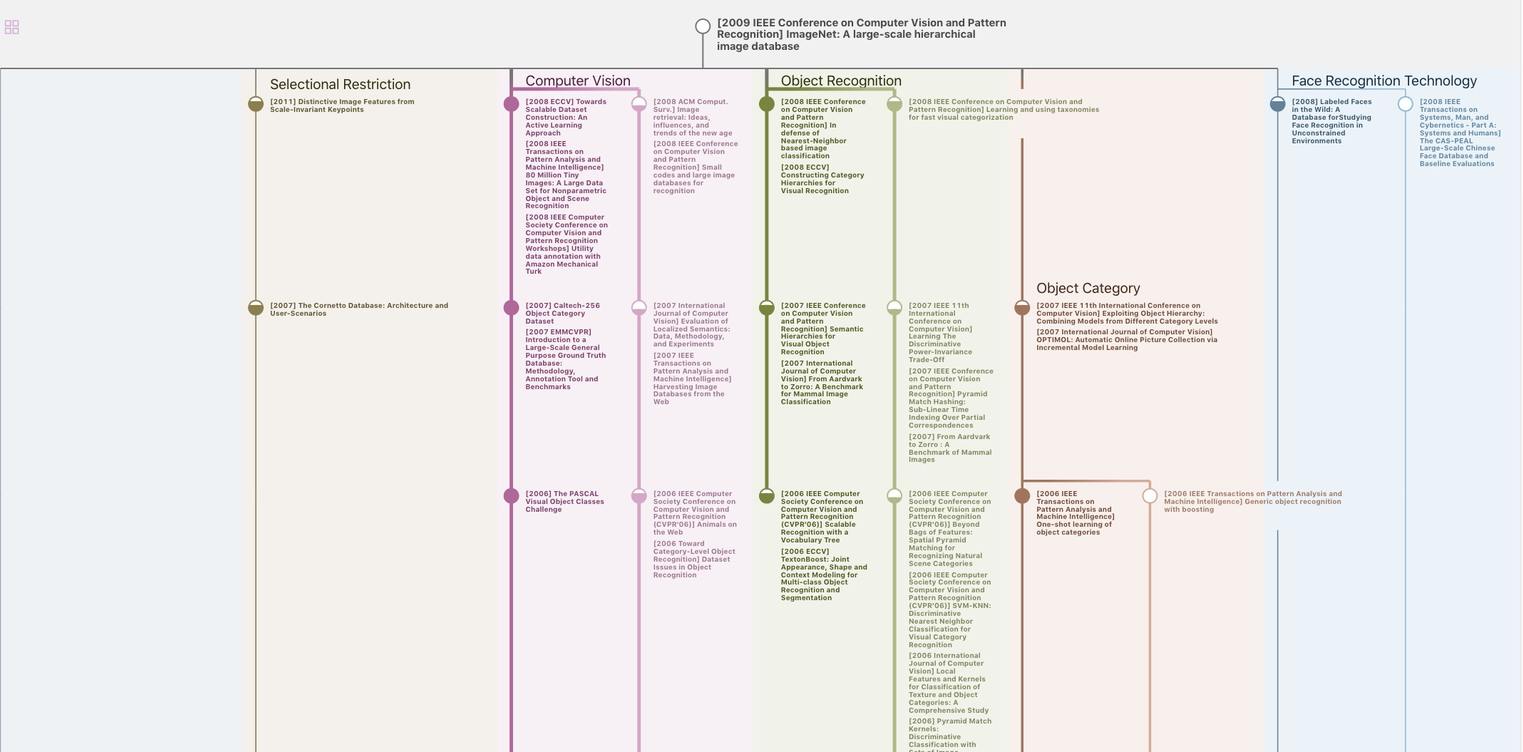
生成溯源树,研究论文发展脉络
Chat Paper
正在生成论文摘要