FLAVR: flow-free architecture for fast video frame interpolation
Mach. Vis. Appl.(2023)
摘要
Many modern frame interpolation approaches rely on explicit bidirectional optical flows between adjacent frames, thus are sensitive to the accuracy of underlying flow estimation in handling occlusions while additionally introducing computational bottlenecks unsuitable for efficient deployment. In this paper, we propose a flow-free approach that is completely end-to-end trainable for multi-frame video interpolation. Our method, FLAVR, leverages 3D spatio-temporal kernels to directly learn motion properties from unlabeled videos and greatly simplifies the process of training, testing and deploying frame interpolation models. As a result, FLAVR delivers up to 6× speed up compared to the current state-of-the-art methods for multi-frame interpolation while consistently demonstrating superior qualitative and quantitative results compared with prior methods on popular benchmarks including Vimeo-90K, SNU-Film, and GoPro. Additionally, we show that frame interpolation is a competitive self-supervised pre-training task for videos by demonstrating various novel applications of FLAVR including action recognition, optical flow estimation, and video object tracking. Code and trained models are available in the project page: https://tarun005.github.io/FLAVR/ . We have provided additional qualitative results for frame interpolation as well as downstream applications in our video available using this web link https://paperid1300.s3.us-west-2.amazonaws.com/FLAVRVideo.mp4 (link).
更多查看译文
关键词
Video frame interpolation,Deep learning,Space-time convolutions
AI 理解论文
溯源树
样例
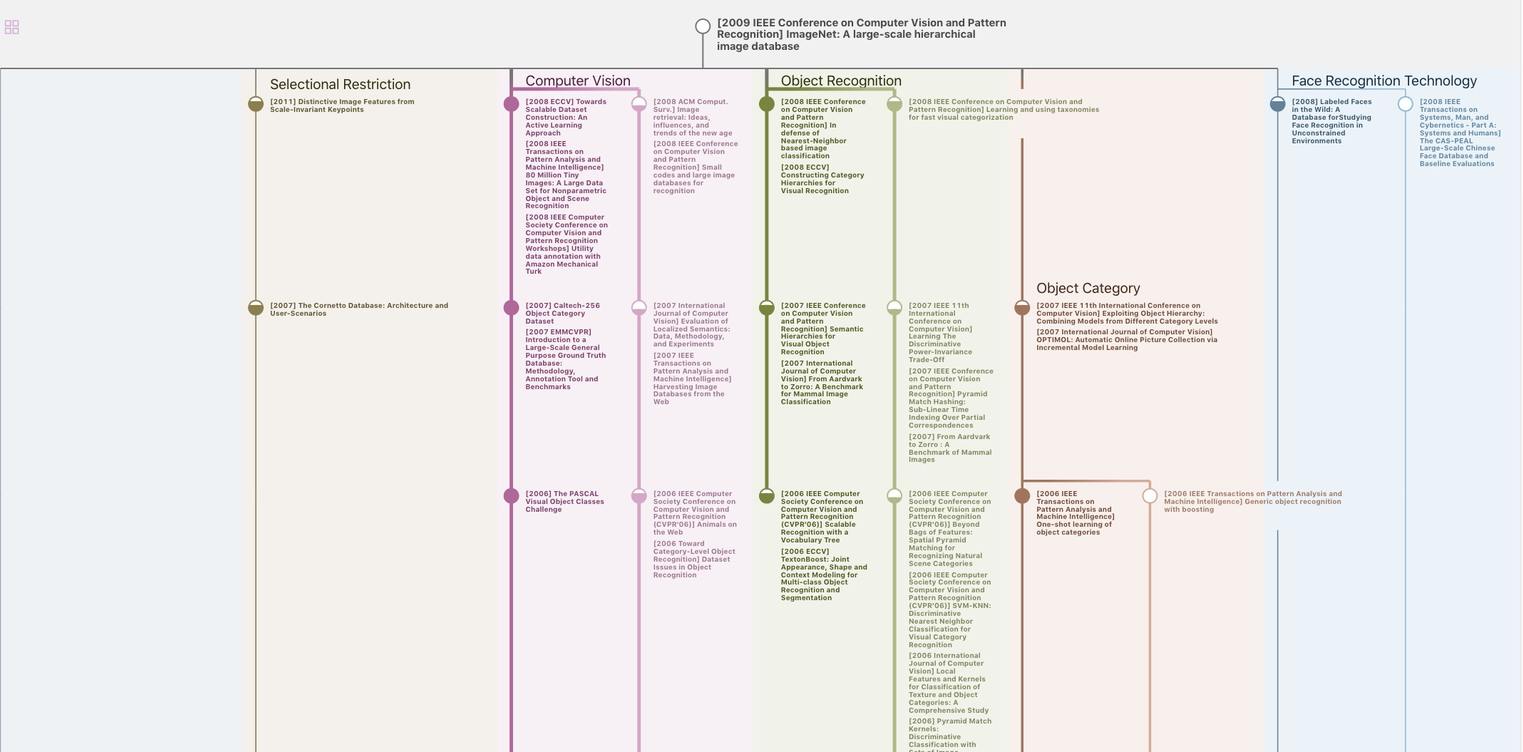
生成溯源树,研究论文发展脉络
Chat Paper
正在生成论文摘要