GrNT: Gate-Regularized Network Training for Improving Multi-Scale Fusion in Medical Image Segmentation.
ISBI(2023)
摘要
Multi-scale fusion is a key for semantic segmentation of medical images. Recent deep learning methods added network complexity to achieve better multi-scale fusion results. However, given the already very expressive architectures (e.g., U-Net), an interesting question is whether additional complexity is necessary for achieving more robust multi-scale fusion performance. In this paper, we proposed a new method for improving the multi-scale fusion performance of a medical image segmentation model. We create a set of binary gates at fusing locations that allow us to control forward and backward flows in training. A gate on-off schedule is imposed so that high-scale (level) features could drive the generation of segmentation, while low-scale features serve as complimentary for reconstructing shape details. Our method is effective, easy to implement, and occurs no extra cost when deploying the trained model. Experiments show that our gate-regularized network training (GrNT) improves widely adopted models (e.g., U-Net, Attention U-Net, and DenseVoxNet) on three segmentation datasets (2017 ISIC Skin Lesion segmentation (2D), MM-WHS CT (3D), and 2016 HVSMR (3D)).
更多查看译文
关键词
2016 HVSMR,attention U-Net,binary gates,deep learning,DenseVoxNet,gate on-off schedule,gate-regularized network training,GrNT,high-scale features,ISIC skin lesion segmentation,low-scale features,medical image segmentation,MM-WHS CT,multiscale fusion performance,network complexity,segmentation datasets,semantic segmentation,shape detail reconstruction
AI 理解论文
溯源树
样例
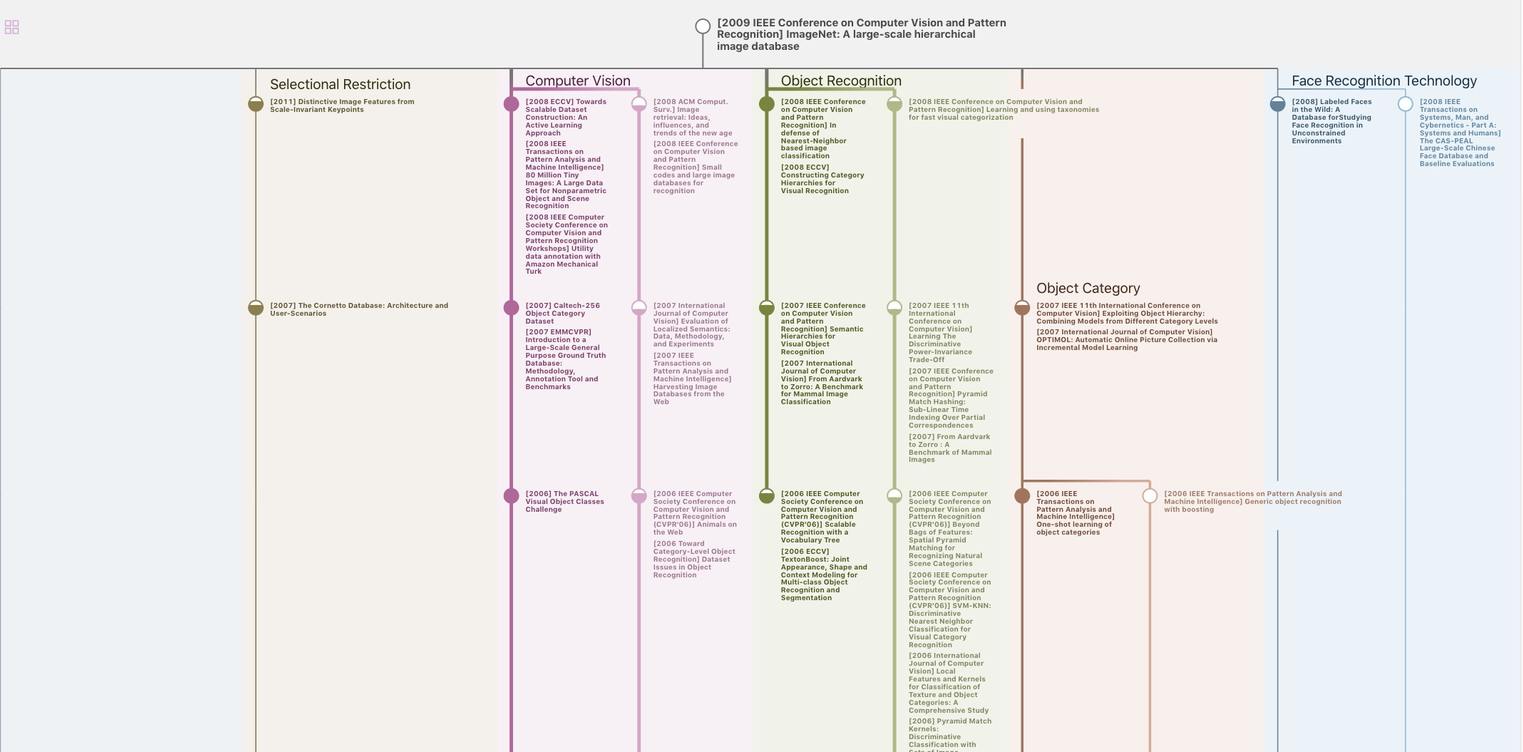
生成溯源树,研究论文发展脉络
Chat Paper
正在生成论文摘要