Analysis of Latent Factors Affecting Video Game Performance Using Machine Learning Methods
ISIE(2023)
摘要
E-sports is the state-of-the-art digital sport where players and teams compete against each other in various gaming disciplines. The most significant part of the industry includes tournaments specifically in multiplayer team-based games where outstanding individual performance is not enough and where a team is perceived as a single mechanism. In such cases, the game performance should not be limited to the individual team member contributions and take into account internal players’ interactions. It is essential to emphasize that e-sports players are humans, therefore, while evaluating their performance it is necessary to take into account the psychological and emotional points. In this work, we propose an approach to the game performance analysis focusing on the factors insufficiently investigated earlier, such as the team interaction efficiency in terms of auxiliary in-game attack tool usage, as well as the teammates’ emotional state described by an arousal-valence model. First, we perform the analysis of a large amount of in-game Counter Strike:Global Offensive discipline tournament data, derive detailed metrics related to the team actions and show their strong correlation with the game outcome characterized by a high level of significance (p-value <0.001). Second, we train the regression models separately for the valence and arousal scales with RMSE 0.497 and 0.561, respectively, and prove their consistency by validation on E-sports audio data. Our findings show that the team performance significantly depends both on the effectiveness of the coordinated grenade use and on the players’ emotional state during the game.
更多查看译文
关键词
Game performance,machine learning,speech emotion recognition,arousal and valence analysis
AI 理解论文
溯源树
样例
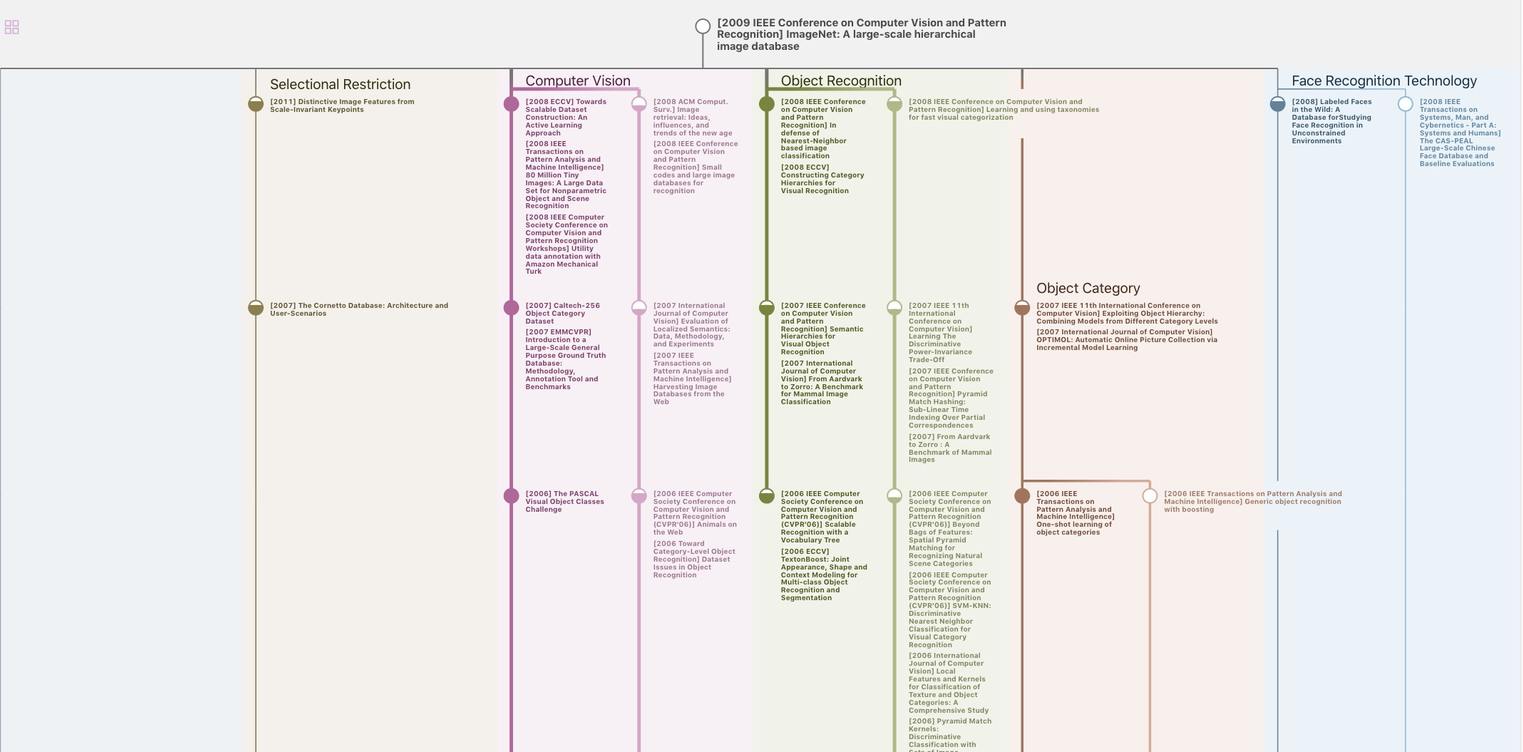
生成溯源树,研究论文发展脉络
Chat Paper
正在生成论文摘要