Power Quality Disturbance Identification Algorithm Based on Empirical Wavelet Transform And Time-Domain Kurtosis Feature Analysis.
ISIE(2023)
摘要
In the process of power quality (PQ) disturbance recognition, there is redundancy in feature extraction of the disturbance signal, so it will lead to complex structure of recognition model, difficult training and low recognition accuracy. To address the above problems, a new method of recognition and classification based on empirical wavelet transform (EWT) with time-domain kurtosis feature analysis and LSTM neural network is proposed. Firstly, EWT is used to obtain the multi-resolution analysis (MRA) components; secondly, the MRA segments are filtered to reduce redundancy by adding windows to the decomposed time-domain signals and calculating the kurtosis so as to determine the starting and ending positions of the effective feature information; to facilitate the subsequent neural network training, the energy entropy is calculated for the filtered MRA segments to obtain the energy entropy sequence. Finally, the LSTM neural network is used for recognition analysis to identify the types of disturbance signals. The simulation results show that the proposed optimized screening method has lower feature vector redundancy, lower complexity of the LSTM recognition model, shorter training time, and an average recognition accuracy of 98.22%, which is 8.04% better than the recognition results of traditional neural networks and 11.04% better than the recognition results of vector machines, compared with the traditional power quality disturbance recognition method.
更多查看译文
关键词
power quality,EWT,kurtosis,energy entropy,LSTM
AI 理解论文
溯源树
样例
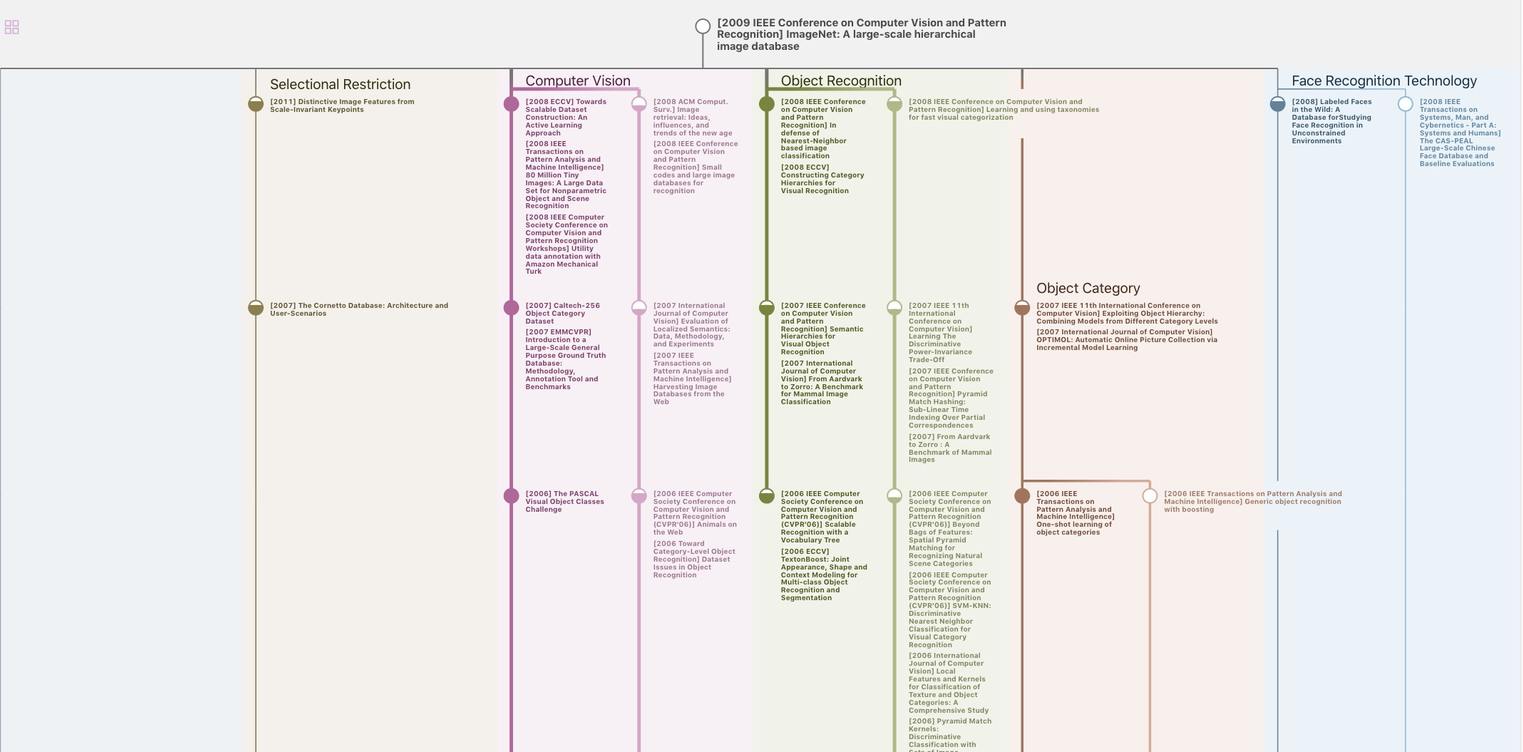
生成溯源树,研究论文发展脉络
Chat Paper
正在生成论文摘要