Federated Learning and Genetic Mutation for Multi-Resident Activity Recognition.
e-Science(2023)
摘要
Multi-Resident activity recognition refers to the task of recognizing activities performed by multiple individuals living in the same residence. It involves using sensors or other monitoring devices to capture data about the activities taking place in the living space, and then using Machine Learning (ML) or Deep Learning (DL) algorithms to analyze and classify these activities. Federated Learning (FL) is a technique that enables multiple devices to collaboratively train a model without sharing their data with each other, while Genetic Mutation (GM) is a technique used in evolutionary algorithms to introduce random changes to the genetic code of individuals in a population. Our proposed framework involves the use FL and GM for Human Activity Recognition (HAR). The approach was evaluated on the ARAS dataset, collected from two houses with different activity patterns. Two Recurrent Neural Network (RNN) models, Gated Recurrent Unit (GRU) and Long-Short Term Memory (LSTM), were employed for the activity classification task and a genetic mutation operator was applied to the weights of the models before federated averaging. The results indicate that FL is suitable for privacy preserving activity recognition, it can help with early deployment and even improve the performance of the models in some cases.
更多查看译文
关键词
activity recognition,multi-resident,deep learning,federated learning,genetic mutation,LSTM,GRU,ARAS
AI 理解论文
溯源树
样例
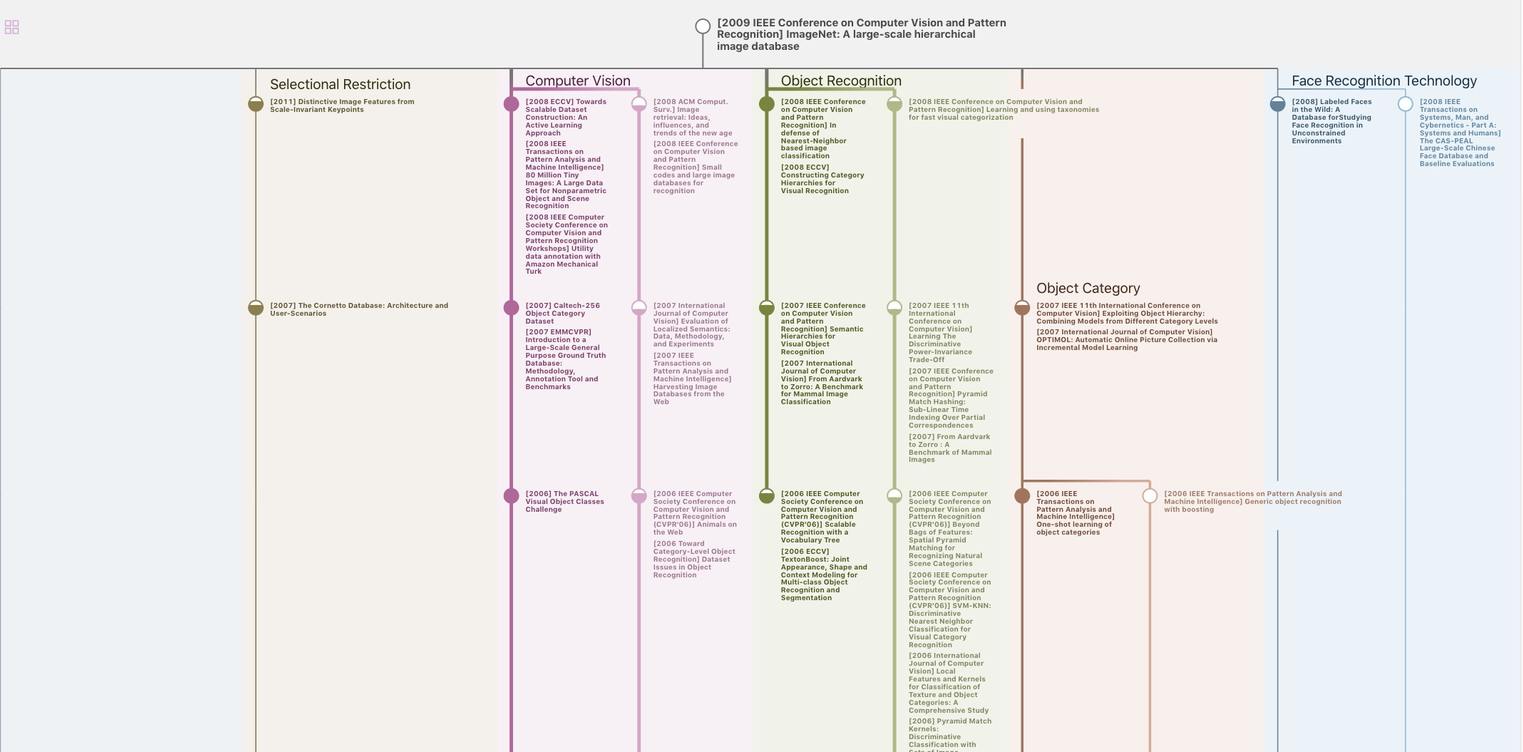
生成溯源树,研究论文发展脉络
Chat Paper
正在生成论文摘要