Multimodal Approaches for Alzheimer's Detection Using Patients' Speech and Transcript.
BI(2023)
摘要
Alzheimer’s disease (AD) is a common form of dementia that severely impacts patient health. As AD impairs the patient’s language understanding and expression ability, the speech of AD patients can serve as an indicator of this disease. This study investigates various methods for detecting AD using patients’ speech and transcripts data from the DementiaBank Pitt database. The proposed approach involves pre-trained language models and Graph Neural Network (GNN) that constructs a graph from the speech transcript, and extracts features using GNN for AD detection. Data augmentation techniques, including synonym replacement and GPT-based augmenter, were used to address the limited sample size issue. Audio data from the patient’s speech was also included in the proposed model, where the WavLM model was used to extract audio features. These features were then fused with text features using various fusion strategies. We also investigated a novel fusion approach, where transcripts data were converted back to audio data and analyzed through a contrastive learning scheme along with the original audio data, with the premise that a single-modal (audio) detection model could be easier to train with better generalizability. We conducted intensive experiments and analysis on the above methods. Our findings shed light on the challenges and potential solutions in AD detection using multi-modal speech data.
更多查看译文
关键词
alzheimers,speech,detection
AI 理解论文
溯源树
样例
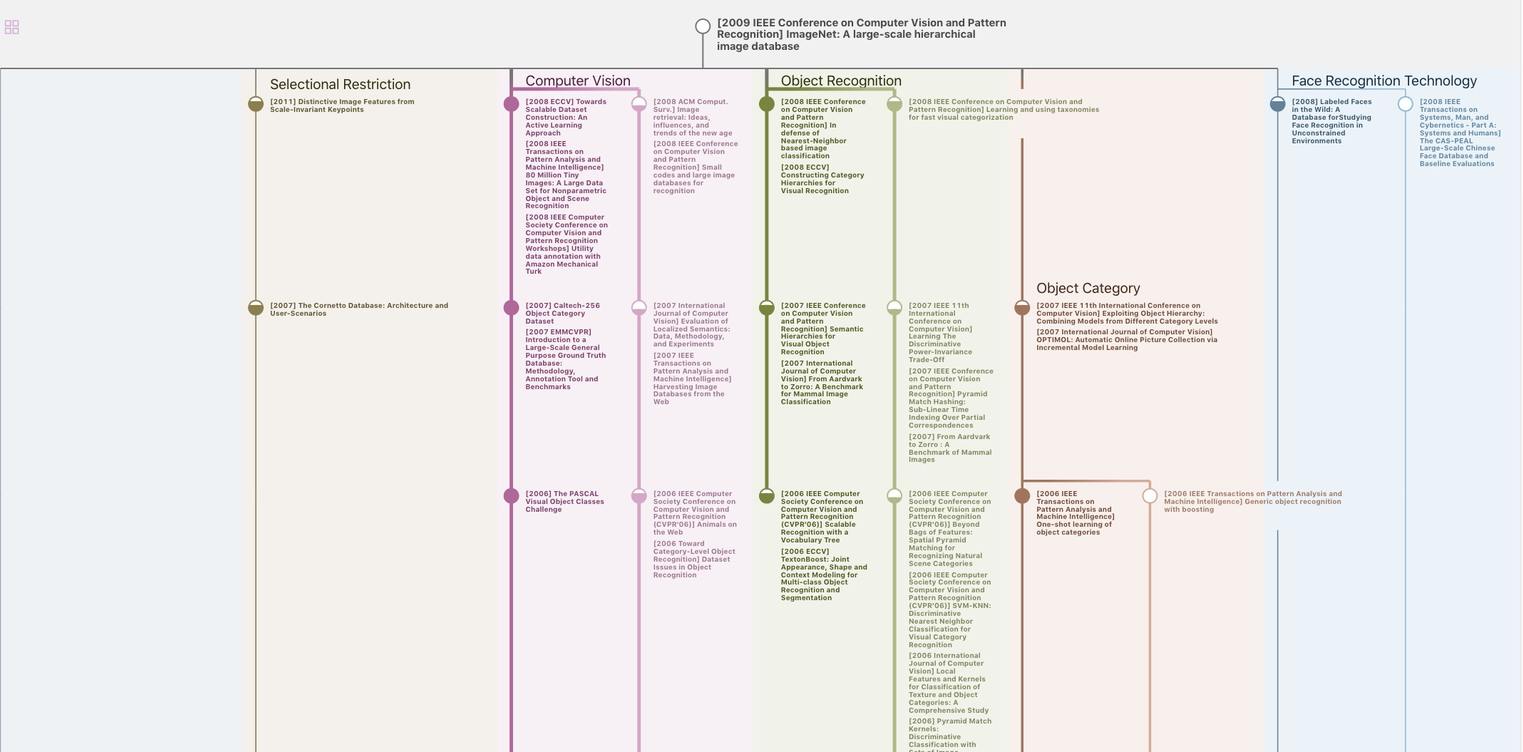
生成溯源树,研究论文发展脉络
Chat Paper
正在生成论文摘要