Comparison Between Explainable AI Algorithms for Alzheimer's Disease Prediction Using EfficientNet Models.
BI(2023)
摘要
Alzheimer’s disease (AD) is a common form of dementia that affects brain regions that control cognition, memory, and language. Globally, more than 55 million people are suffering from dementia. Given these troubling statistics, predicting AD is critical for future medications and treatment. Mild Cognitive Impairment (MCI) is a vital stage for patients because from this stage a majority of patients turn into AD patients. Early MCI (EMCI) and Late MCI (LMCI) are vital two stages of MCI. Successful prediction of Cognitive Normal (CN), AD, MCI, EMCI, and LMCI stages is a big challenge. Although there are models for predicting these stages but all models are not near to accurate. One major concern for these lacking is not having sufficient datasets to train the model. Data augmentation can be a solution to create an abundance of MRI data. One major issue regarding Machine Learning (ML) is the black box nature. Due to this limitation, user satisfaction as well as trust in the model’s prediction is missing. Explainable Artificial Intelligence (XAI) is the torchbearer approach and through this, the reason behind every decision can be observed by the user. This paper proposes a comparison between four XAI models named Gradient-weighted Class Activation Mapping (Grad-CAM), Grad-CAM++, Score-weighted CAM (Score-CAM), and Faster Score-CAM. For performing the five classes (CN, AD, EMCI, MCI, and LMCI) prediction, EfficientNet models are used because these models have performed well on the augmented dataset. Among EfficientNet models (B0-B7), EfficientNetB7 has performed the best. The testing accuracy and loss are 96.34% and 0.12, respectively. After that, the last layer of the EfficientNetB7 model is passed to the XAI models. Comparing the four XAI models it is observed that Grad-CAM++ and Score-CAM are better performing than others.
更多查看译文
关键词
explainable explainable algorithms,disease prediction,alzheimers
AI 理解论文
溯源树
样例
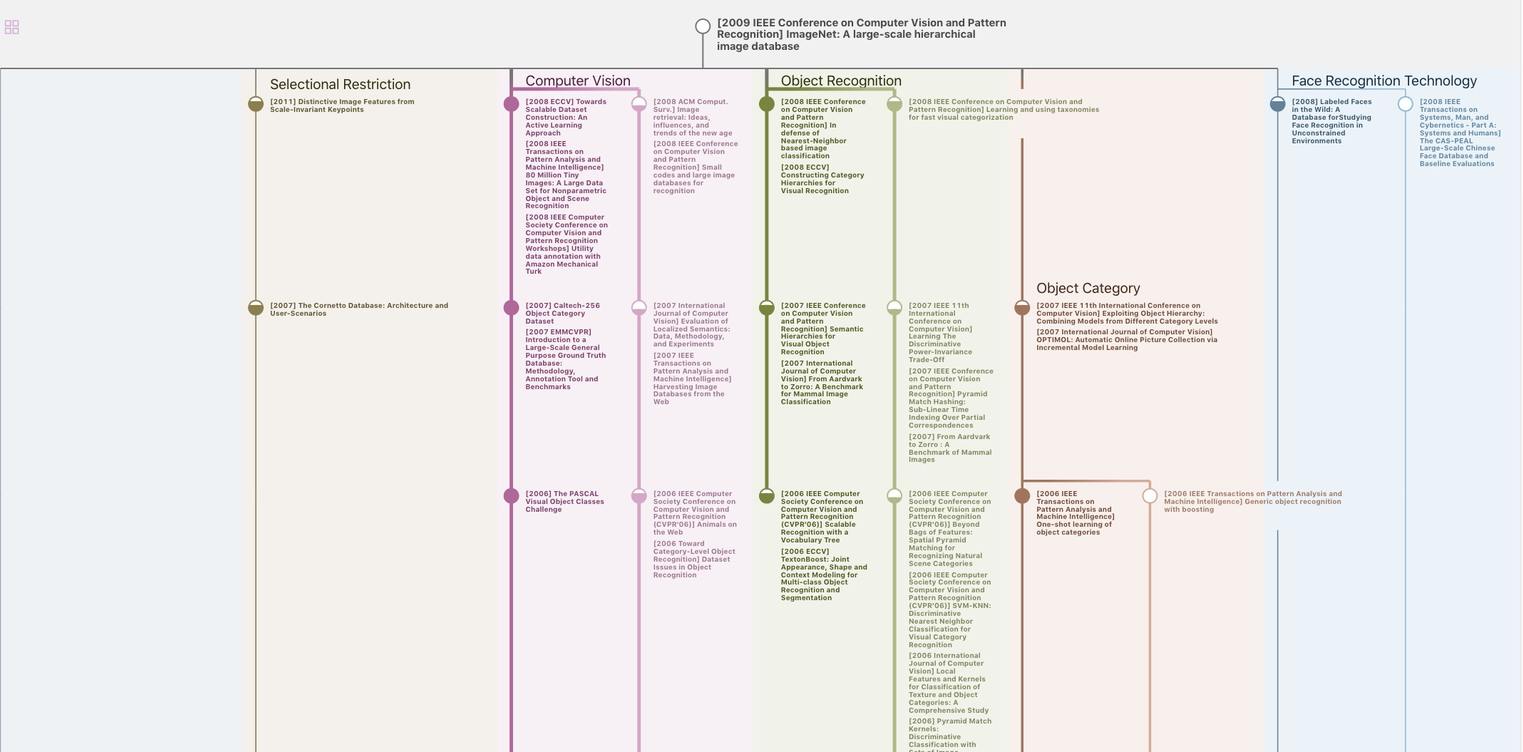
生成溯源树,研究论文发展脉络
Chat Paper
正在生成论文摘要