Nowhere to Hide: Detecting Live Video Forgery via Vision-WiFi Silhouette Correspondence.
INFOCOM(2023)
摘要
For safety guard and crime prevention, video surveillance systems have been pervasively deployed in many security-critical scenarios, such as the residence, retail stores, and banks. However, these systems could be infiltrated by the adversary and the video streams would be modified or replaced, i.e., under the video forgery attack. The prevalence of Internet of Things (IoT) devices and the emergence of Deepfake-like techniques severely emphasize the vulnerability of video surveillance systems under such attacks. To secure existing surveillance systems, in this paper we propose a vision-WiFi cross-modal video forgery detection system, namely WiSil. Leveraging a theoretical model based on the principle of signal propagation, WiSil constructs wave front information of the object in the monitoring area from WiFi signals. With a well-designed deep learning network, WiSil further recovers silhouettes from the wave front information. Based on a Siamese network-based semantic feature extractor, WiSil can eventually determine whether a frame is manipulated by comparing the semantic feature vectors extracted from the video’s silhouette with those extracted from the WiFi’s silhouette. Extensive experiments show that WiSil can achieve 95% accuracy in detecting tampered frames. Moreover, WiSil is robust against environment and person changes.
更多查看译文
关键词
WiFi Sensing,Video Forgery Detection,Deep Learning
AI 理解论文
溯源树
样例
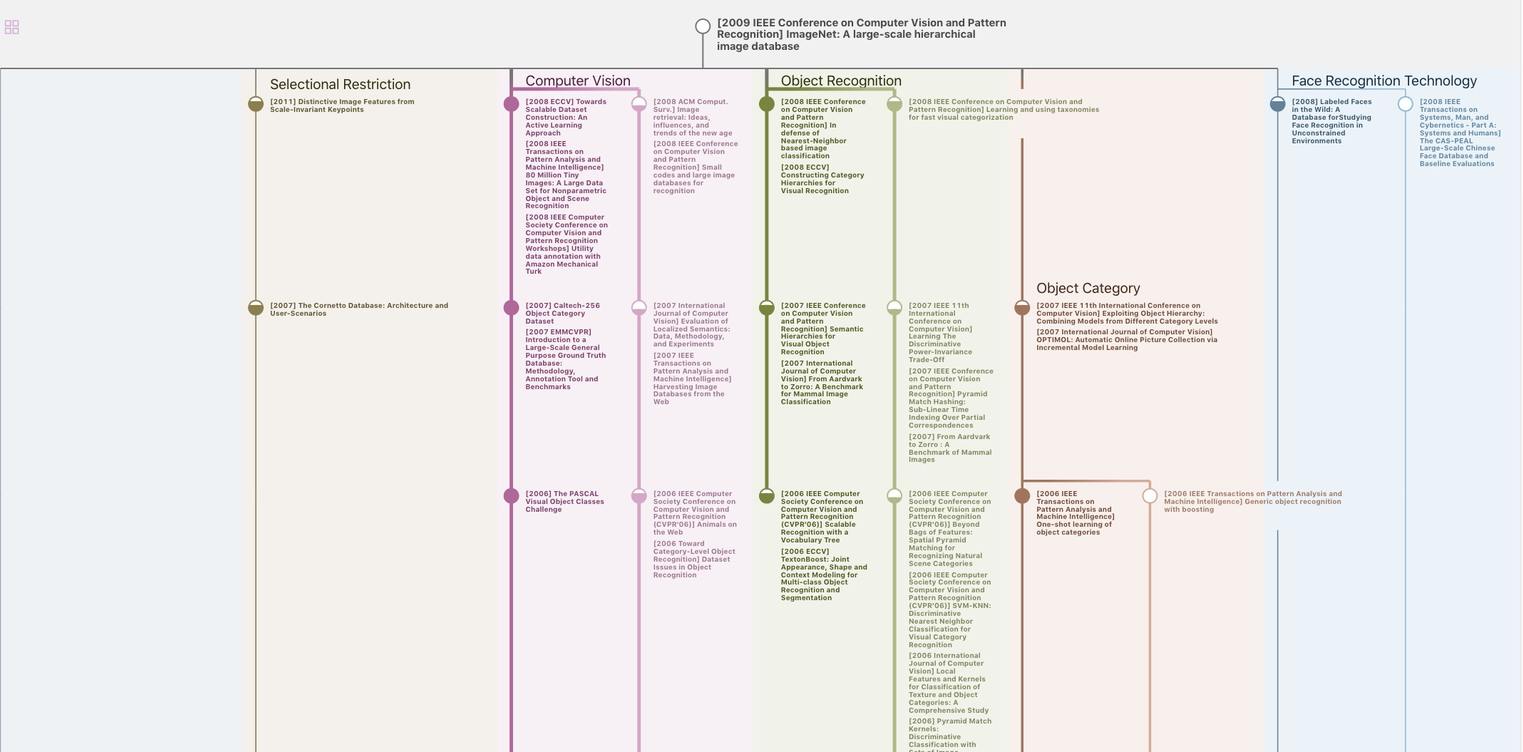
生成溯源树,研究论文发展脉络
Chat Paper
正在生成论文摘要