Fast Generation-Based Gradient Leakage Attacks against Highly Compressed Gradients.
INFOCOM(2023)
摘要
Federated learning (FL) is a distributed machine learning technology that preserves data privacy. However, it has been shown to be vulnerable to gradient leakage attacks (GLA), which can reconstruct private training data from public gradients with an overwhelming probability. Nevertheless, these attacks either require modification of the FL model (analytics-based) or take a long time to converge (optimization-based) and fail in dealing with highly compressed gradients in practical FL systems. In this paper, we pioneer a generation-based GLA method called FGLA that can reconstruct batches of user data, forgoing the optimization process. Specifically, we design a feature separation technique that extracts the feature of each data in a batch and then generates user data directly. Extensive experiments on multiple image datasets demonstrate that FGLA can reconstruct user images in milliseconds with a batch size of 256 from highly compressed gradients (0.8% compression ratio or higher), thus substantially outperforming state-of-the-art methods.
更多查看译文
关键词
Federated learning,data privacy,gradient leakage attack,image generation
AI 理解论文
溯源树
样例
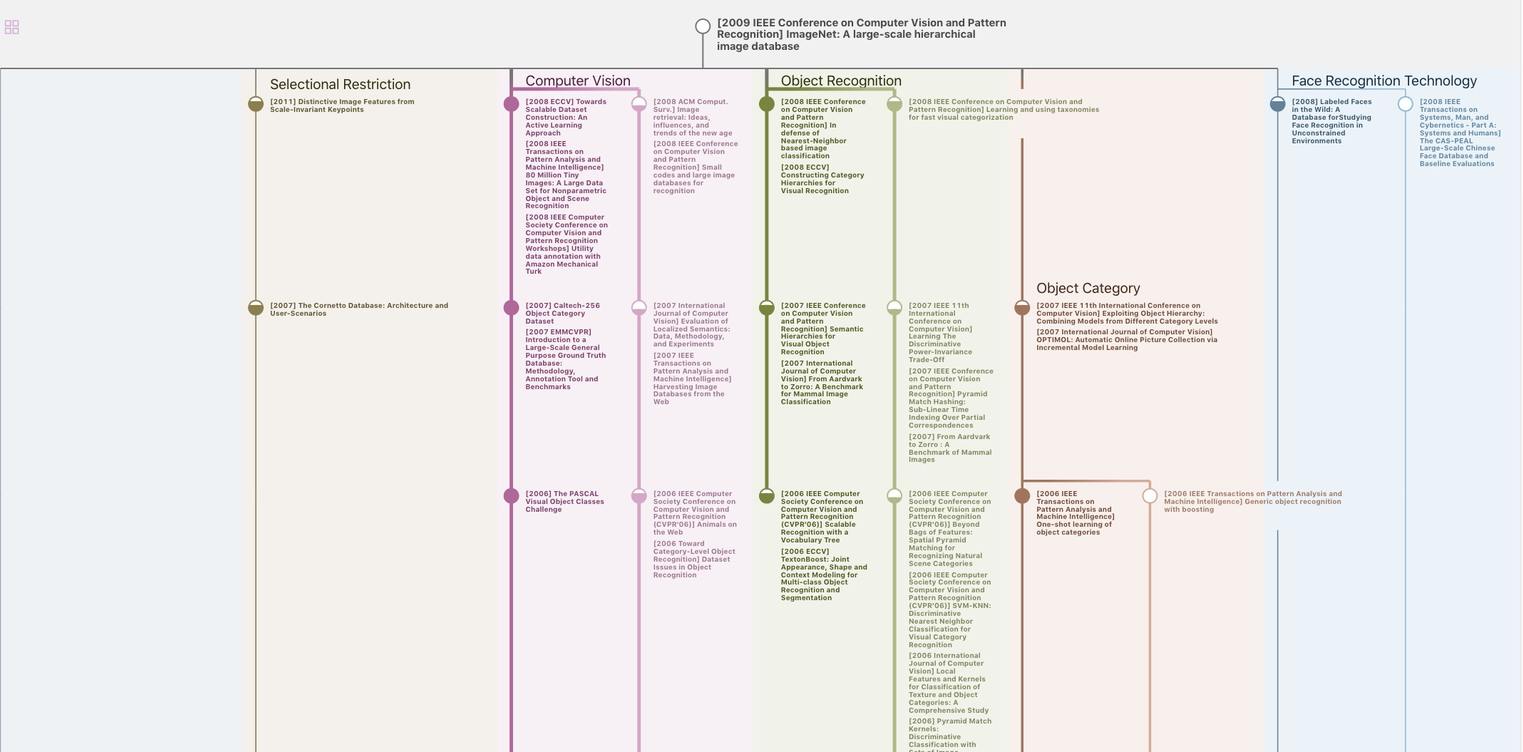
生成溯源树,研究论文发展脉络
Chat Paper
正在生成论文摘要