Multi-Agent Deep Reinforcement Learning for Digital Twin over 6G Wireless Communication in the Metaverse.
INFOCOM Workshops(2023)
摘要
Technology advancements in wireless communications and high-performance Extended Reality (XR) have empowered the developments of the Metaverse. The demand for Metaverse applications and hence, real-time digital twinning of real-world scenes is increasing. Nevertheless, the replication of 2D physical world images into 3D virtual world scenes is computationally intensive and requires computation offloading. The disparity in transmitted scene dimension (2D as opposed to 3D) leads to asymmetric data sizes in uplink (UL) and downlink (DL). To ensure the reliability and low latency of the system, we consider an asynchronous joint UL-DL scenario where the smaller data size of the physical world scenes captured in the UL will be uploaded to the Metaverse Console (MC) to be construed and rendered, and the larger-size 3D virtual world scenes need to be transmitted back in DL. The decisions pertaining to computation offloading and channel assignment are optimized in the UL stage, and the MC will optimize power allocation for users assigned with a channel in the UL transmission stage. To ensure the reliability and low latency of the system, we design a novel Multi-agent Deep Reinforcement Learning (MADRL) structure, namely Asynchronous Actors Hybrid Critic (AAHC). The simulation experiments demonstrate that compared to the proposed baselines, AAHC obtains better solutions with a preferable training time.
更多查看译文
关键词
Reinforcement learning,resource allocation,latency,reliability,wireless communications,Metaverse
AI 理解论文
溯源树
样例
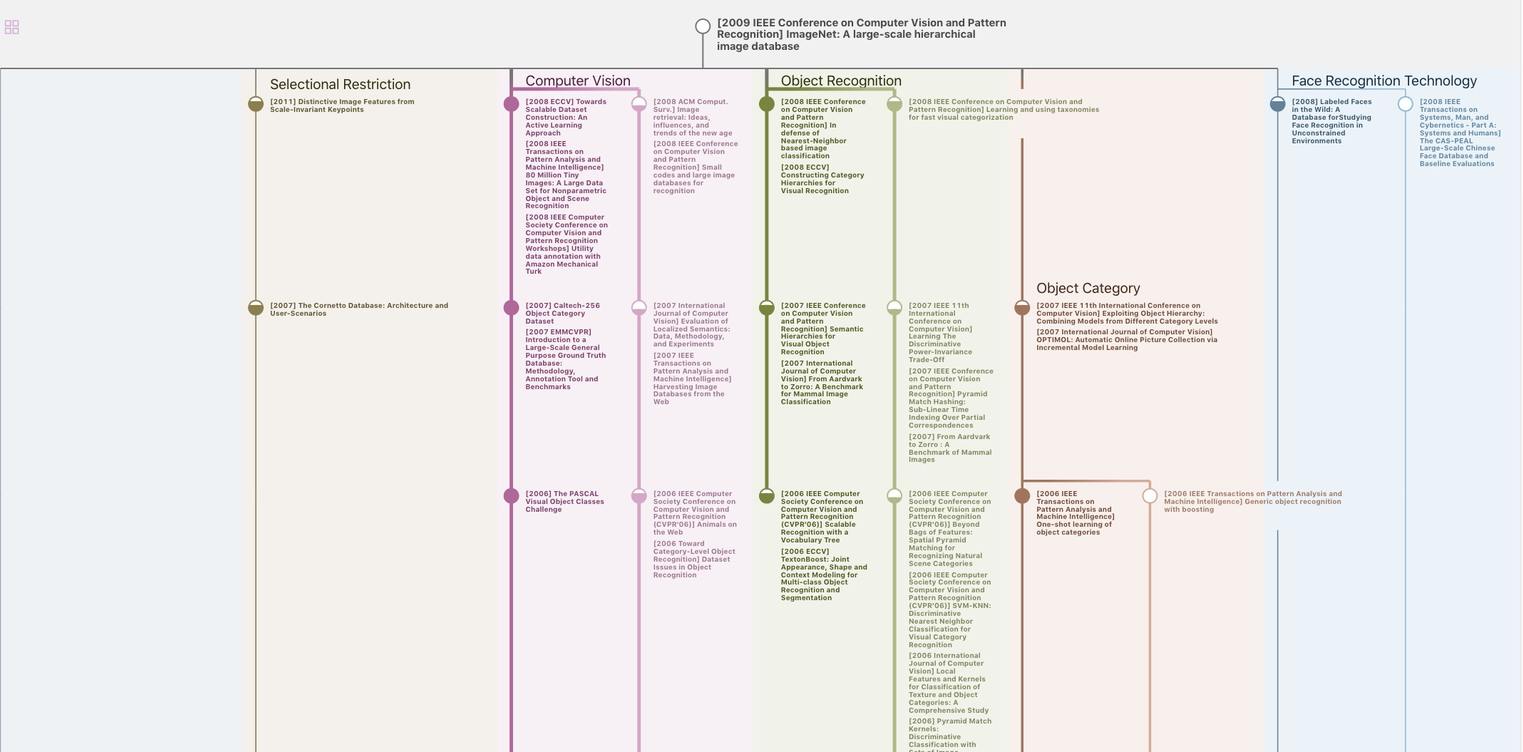
生成溯源树,研究论文发展脉络
Chat Paper
正在生成论文摘要