Efficient and Lightweight Neuron Morphology Classification Using Gabor Convolutional Networks
INNS DLIA@IJCNN(2023)
摘要
Due to the complex and diverse morphology of neurons, achieving accurate classification of neurons is not an easy task. Most existing methods for neuron classification are based on traditional machine learning algorithms, which not only have high design costs but also poor transferability. Gabor Orientation Filters (GoFs), obtained by modulating Gabor filters and traditional convolutional kernels, can better extract geometric features of neurons and enhance the adaptability of deep learning features to GoFs' orientation and scale changes. Therefore, this paper proposes an efficient and lightweight Gabor Convolutional Network (GCN) for neuron morphology classification based on the geometric shape data of neurons and the spatial transformation capabilities of Gabor filters. The network consists of five consecutive Gabor convolutional layers for extracting geometric features of neurons and two linear layers for neuron morphology classification. Experimental results show that this method achieves accuracy rates of 94.26%, 87.16%, and 87.72% for binary classification and 96.52%, 85.96%, and 86.73% for 12-class classification on the Img_raw, Img_resample, and Img_XYalign neuron morphology datasets, respectively. Meanwhile, compared with classical classification models, the experimental results show that this method can capture enough neuron morphology features with fewer convolutional layers, and it has good classification accuracy and fewer model parameters.
更多查看译文
关键词
neuron morphological classification,gabor filter,gabor convolutional network,deep learning
AI 理解论文
溯源树
样例
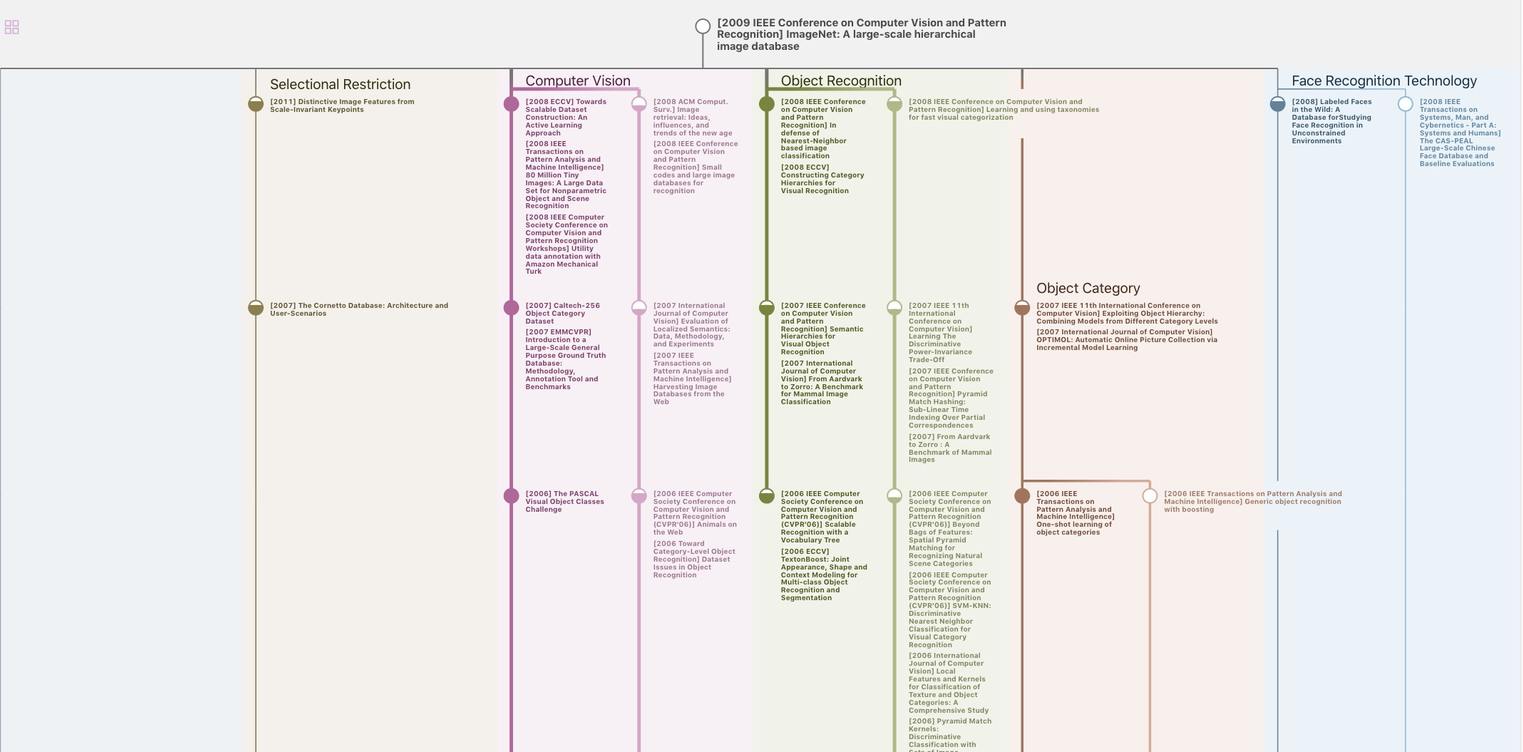
生成溯源树,研究论文发展脉络
Chat Paper
正在生成论文摘要