Revisiting Histogram Based Outlier Scores: Strengths and Weaknesses.
HAIS(2023)
摘要
Anomaly detection is a crucial task in various domains such as finance, cybersecurity or medical diagnosis. The demand for interpretability and explainability in model decisions has revived the use of traceable models, with Histogram Based Outlier Scores being a notable option due to its fast speed and commendable performance. Histogram Based Outlier Scores is a well-known and efficient unsupervised anomaly detection algorithm. Despite its popularity, it suffers from several limitations, including the inability to update its internal knowledge, model complex distributions, and consider feature relations. This work aims to provide a comprehensive analysis of the Histogram Based Outlier Scores algorithm status and its limitations. We conduct a comparative analysis of Histogram Based Outlier Scores with other state-of-the-art anomaly detection algorithms to identify its strengths and weaknesses. Our study shows that while Histogram Based Outlier Scores is efficient and computationally inexpensive, it may not be the best option in scenarios where the underlying data distribution is complex or where variable relations play a significant role. The presented alternatives and extensions to Histogram Based Outlier Scores provide valuable insights into the development of future anomaly detection methods.
更多查看译文
关键词
histogram based outlier scores
AI 理解论文
溯源树
样例
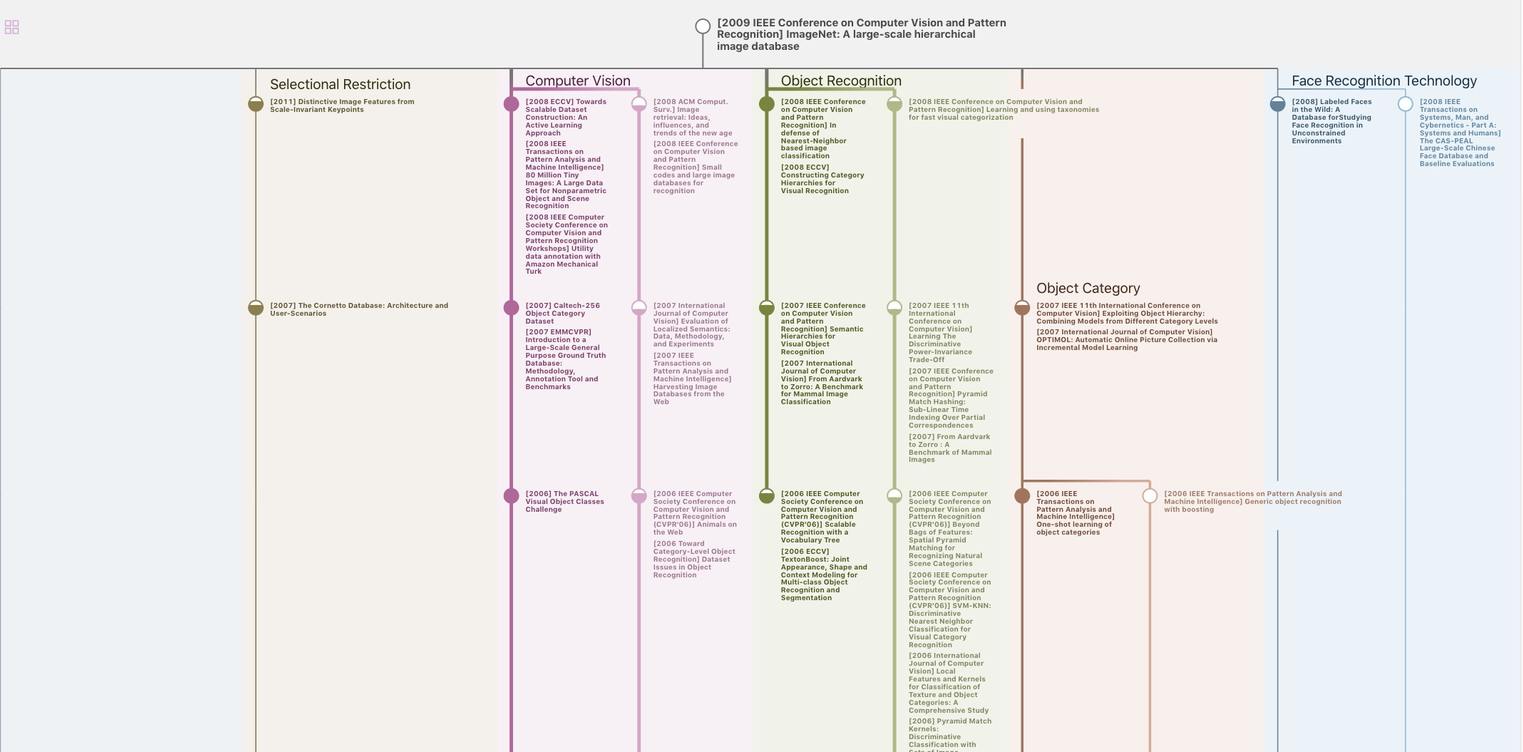
生成溯源树,研究论文发展脉络
Chat Paper
正在生成论文摘要