Exploratory Study of Data Sampling Methods for Imbalanced Legal Text Classification.
HAIS(2023)
摘要
This article investigates the application of machine learning algorithms in the legal domain, focusing on text classification tasks and addressing the challenges posed by imbalanced class distributions. Given the very high number of ongoing legal cases in Brazil, the integration of machine learning tools in the workflow of courts has the potential to enhance justice efficiency and speed. However, the imbalanced nature of legal datasets presents a significant hurdle for traditional machine learning algorithms, which tend to prioritize the majority class and disregard minority classes. To mitigate this problem, researchers have developed imbalance learning techniques that either modify supervised learning or improve the dataset class distribution to improve predictive performance. Data sampling techniques, such as oversampling and undersampling, play a crucial role in balancing class distributions and enabling the training of accurate machine learning models. In this study, a real dataset comprising lawsuits from the Court of Justice of São Paulo, in the state of São Paulo, Brazil, is used to evaluate the effects of different imbalance learning techniques, including oversampling, undersampling, and combined methods, in predictive performance for a binary classification task. The experimental results provided valuable insights into the comparative performance of these techniques and their applicability in the legal domain.
更多查看译文
关键词
imbalanced legal text classification,data sampling methods
AI 理解论文
溯源树
样例
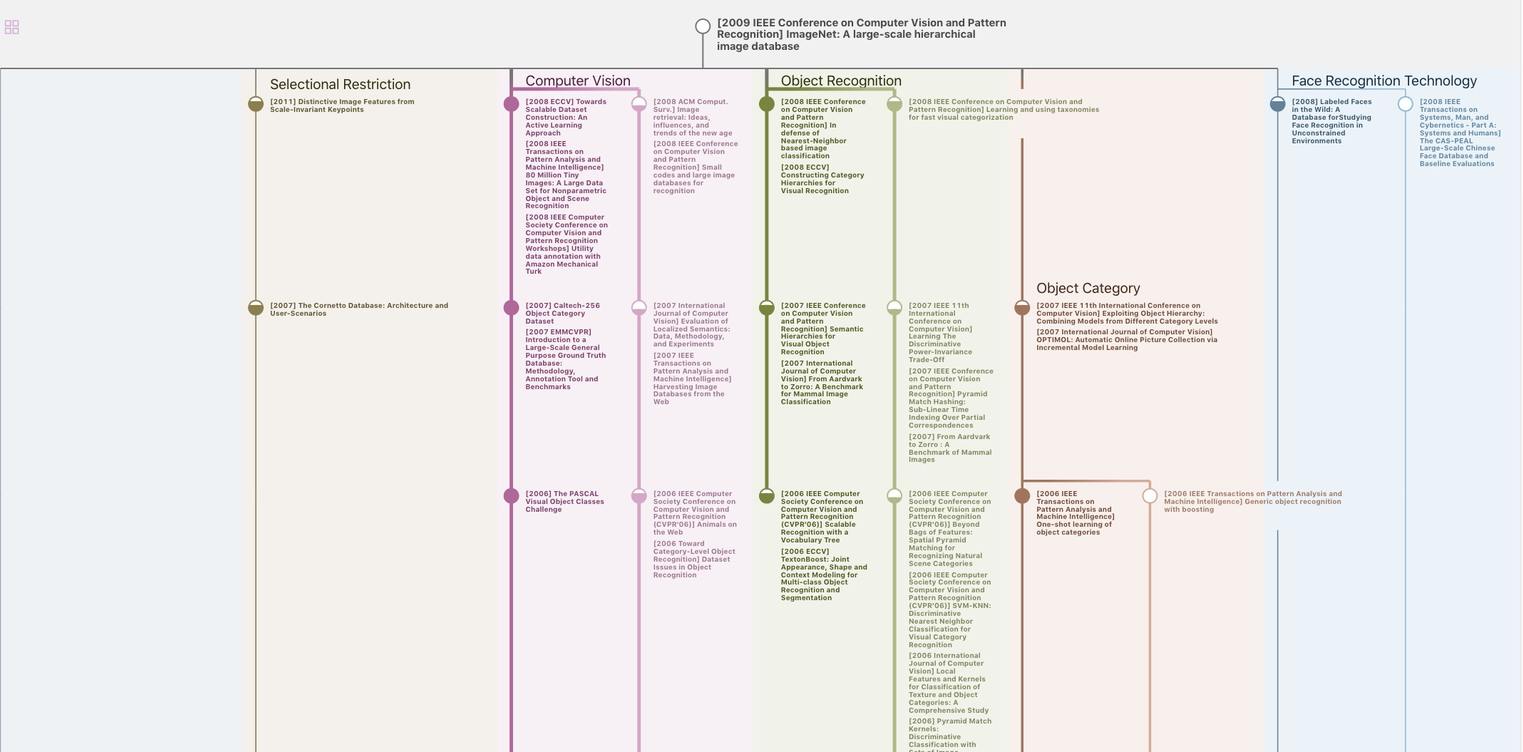
生成溯源树,研究论文发展脉络
Chat Paper
正在生成论文摘要