AutoML: advanced tool for mining multivariate plant traits
Trends in Plant Science(2023)
摘要
Automated machine learning (AutoML) is an automated version of machine learning (ML) that has the potential to become an integral part of plant science research for dealing with large and complex multivariate datasets. AutoML is rapidly advancing and has the potential to revolutionise the development and deployment of ML as it fully automates the ML process, from selecting the appropriate model and optimising its hyperparameters, to handling data preparation in some instances. This automation saves time and effort, enhances model quality, and increases its usability for novice users such as plant scientists. AutoML excels in extracting meaningful features from diverse datasets in plant science domains, enhancing understanding of precision agriculture, crop breeding, and disease forecasting; estimating abiotic stresses, molecular genetics, and proteomics analysis; and yield prediction. As AutoML tools evolve, they will become even more powerful and user-friendly, accelerating innovation in plant science. AutoML automates feature engineering and scalability, making it easier to extract meaningful information from complex multivariate and large biological datasets. Efficiency is increased by automating repetitive tasks, reducing errors, and automatically selecting the best features and models for improved accuracy. Reproducibility is improved by generating a detailed report of the model-building and evaluation process. ML is democratised by providing a drag-and-drop interface that eliminates the need for programming skills, making ML accessible and usable for beginners. It integrates seamlessly with existing tools and pipelines, streamlining research workflows and simplifying model building. Interpreting AutoML, particularly deep learning models, can occasionally present challenges in gaining insights from data, like traditional ML. Therefore, consideration of interpretable AutoML tools may be required in some projects. In some cases, AutoML training and implementation can be challenged by limited or missing data in plant science. It lacks the same customisation or flexibility as coding-based approaches, necessitating careful evaluation and domain-specific knowledge. Most AutoML platforms are versatile and can be used for plant science research. Some are already being used in this field, but others still need to be tested, deployed, and evaluated in the specific plant science domain. No interests are declared.
更多查看译文
关键词
traits,automl,advanced tool,plant
AI 理解论文
溯源树
样例
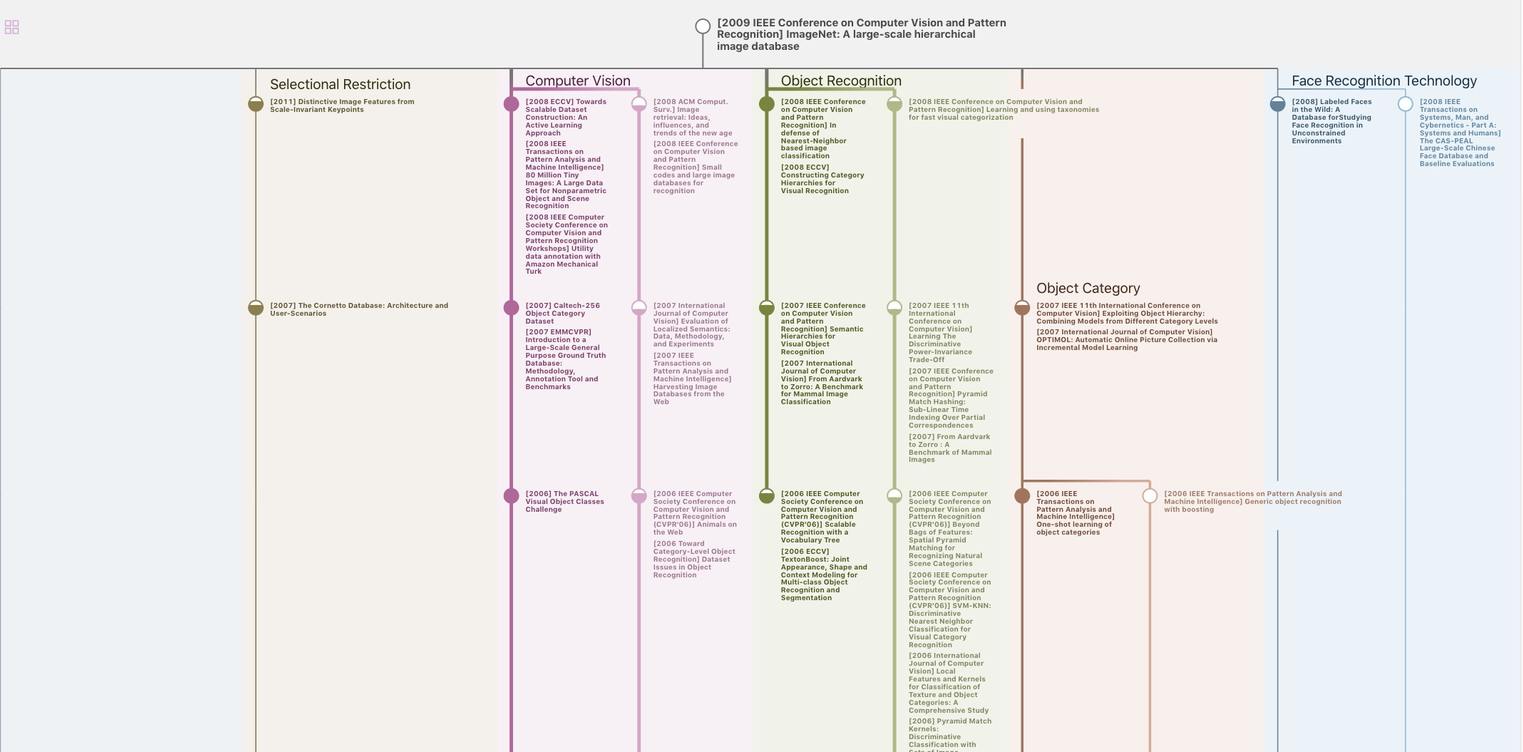
生成溯源树,研究论文发展脉络
Chat Paper
正在生成论文摘要