On the inter-dataset generalization of machine learning approaches to Parkinson's disease detection from voice.
International journal of medical informatics(2023)
摘要
BACKGROUND AND OBJECTIVE:Parkinson's disease is the second-most-common neurodegenerative disorder that affects motor skills, cognitive processes, mood, and everyday tasks such as speaking and walking. The voices of people with Parkinson's disease may become weak, breathy, or hoarse and may sound emotionless, with slurred words and mumbling. Algorithms for computerized voice analysis have been proposed and have shown highly accurate results. However, these algorithms were developed on single, limited datasets, with participants possessing similar demographics. Such models are prone to overfitting and are unsuitable for generalization, which is essential in real-world applications.
METHODS:We evaluated the computerized Parkinson's disease diagnosis performance of various machine learning models and showed that these models degraded rapidly when used on different datasets. We evaluated two mainstream state-of-the-art approaches, one based on deep convolutional neural networks and another based on voice feature extraction followed by a shallow classifier (i.e., extreme gradient boosting (XGBoost)).
RESULTS:An investigation with four datasets (CzechPD, PC-GITA, ITA, and RMIT-PD) proved that even if the algorithms yielded excellent performance on a single dataset, the results obtained on new data or even a mix of datasets were very unsatisfactory.
CONCLUSIONS:More work needs to be done to make computerized voice analysis methods for Parkinson's disease diagnosis suitable for real-world applications.
更多查看译文
AI 理解论文
溯源树
样例
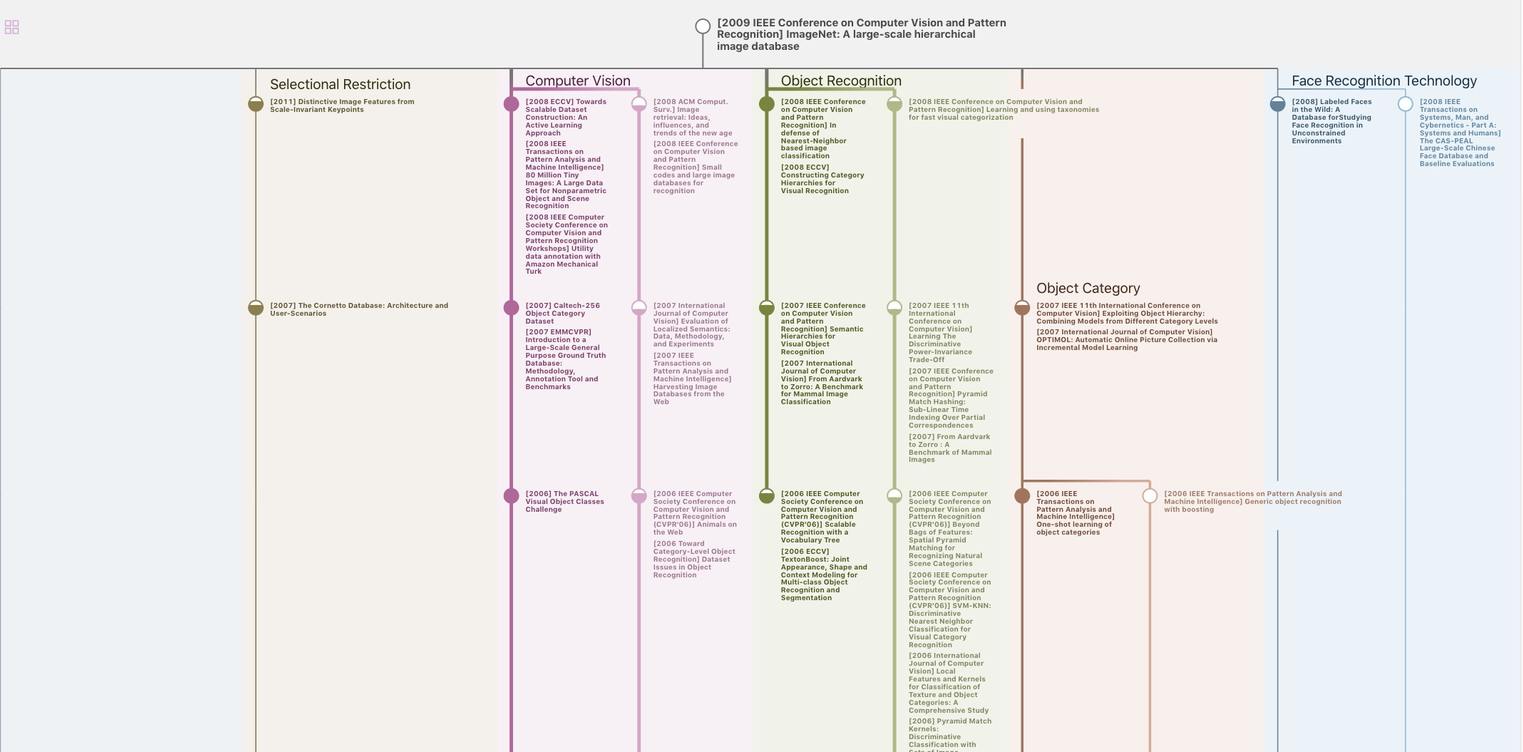
生成溯源树,研究论文发展脉络
Chat Paper
正在生成论文摘要