Designing Multi-Class Classifiers for Sub-mA Microcontroller Platforms
2023 International Conference on Consumer Electronics - Taiwan (ICCE-Taiwan)(2023)
摘要
With the prevalence of deep learning technology in various application domains, a recent trend is to deploy convolutional neural networks onto the sensors in the field enabling new smart applications, such as poaching detection, discovering endanger species, and estimating wildlife populations in environmental monitoring. Among the ultra-low-power hardware platforms with microcontrollers for the computations, the sub-mA platforms (i.e., lower-end ultra-low-power platforms) would be desired for monitoring in the field since they can achieve a longer lifetime given a fixed amount of power budget provided by the batteries. In this work, we propose a new approach to develop convolutional neural networks to run on such sub-mA platforms, usually with less than 10 KB memory for keeping the runtime data. The new approach combines the concepts of the One-vs-All (OVA) strategy and the transfer learning technology to build networks with small memory footprints. Our experimental results show our approach achieves the accuracy of 77.0% of a four-class classification problem for a poaching detection application as fast as 1.1 seconds.
更多查看译文
关键词
convolutional neural networks,deep learning technology,designing multiclass classifiers,environmental monitoring,estimating wildlife populations,four-class classification problem,one-vs-all strategy,OVA strategy,poaching detection application,small memory footprints,sub-mA microcontroller platforms,transfer learning technology,ultra-low-power hardware platforms
AI 理解论文
溯源树
样例
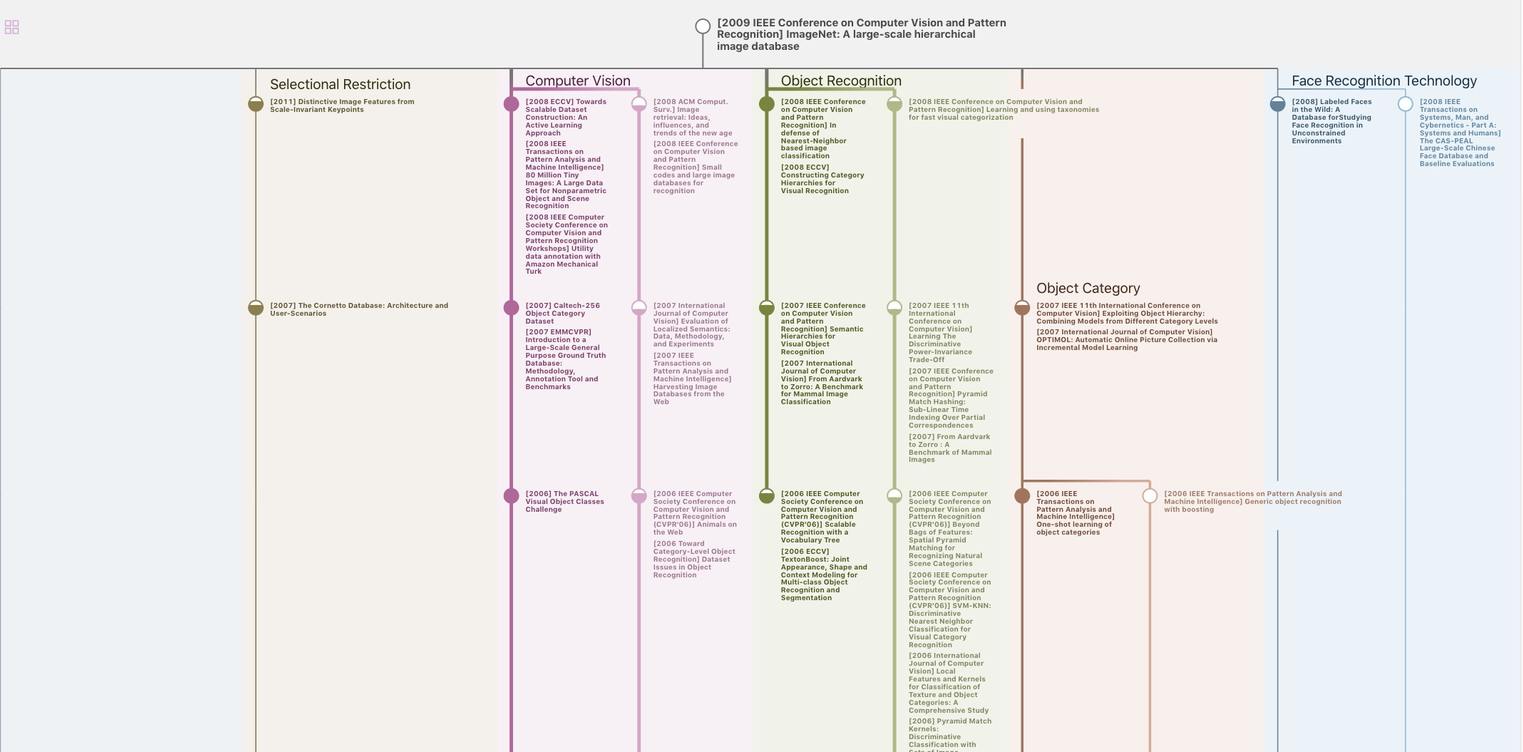
生成溯源树,研究论文发展脉络
Chat Paper
正在生成论文摘要