Nanotope: A Graph Neural Network-Based Model for Nanobody paratope prediction
bioRxiv (Cold Spring Harbor Laboratory)(2023)
摘要
Nanobodies are artificial antibodies derived from the immune system of camelid species, obtained through artificial processing and isolation of antigen-binding proteins. Their small molecular size and high specificity endow nanobodies with extensive potential applications in various fields. However, determining nanobody paratopes through experimental methods is both costly and time-consuming, while traditional computational approaches often lack sufficient accuracy. To enhance the prediction accuracy, we propose Nanotope, a structurebased model capable of efficiently and accurately predicting paratopes, utilizing graph neural networks and an antibody pretrained language model. Our approach primarily leverages sequence features obtained from the AntiBERTy antibody pretrained language model and three-dimensional spatial structure features of nanobodies as inputs. Employing one-dimensional convolution, EGConv graph convolution, and GATConv convolution, we predict the probability of nanobody paratopes with a much higher accuracy. Our method significantly improves the prediction of nanobody paratopes and requires only nanobody sequences and structural information, without the need for additional antigen information. Source code is freely available at
### Competing Interest Statement
The authors have declared no competing interest.
更多查看译文
关键词
prediction,network-based
AI 理解论文
溯源树
样例
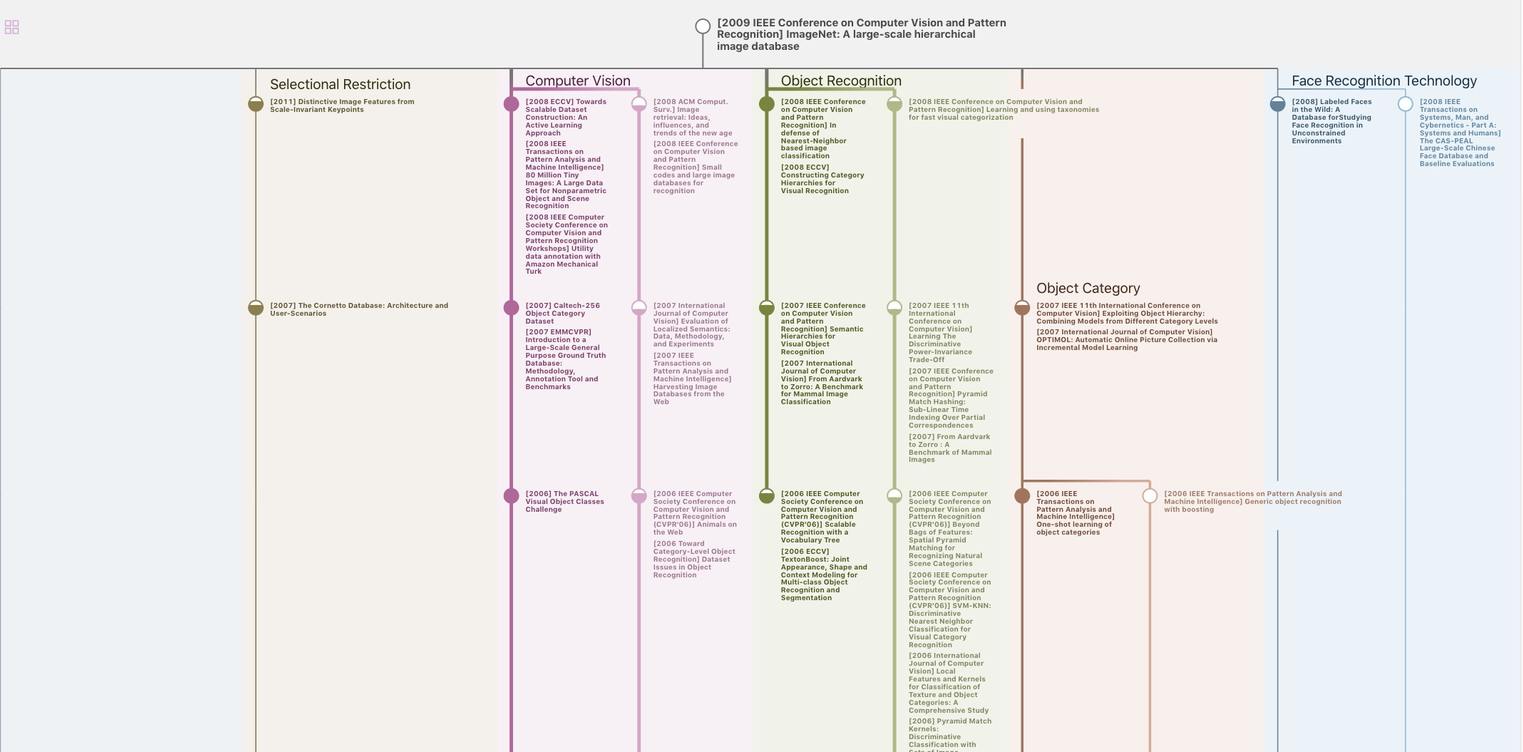
生成溯源树,研究论文发展脉络
Chat Paper
正在生成论文摘要