Visual SLAM Integration With Semantic Segmentation and Deep Learning: A Review
IEEE Sensors Journal(2023)
摘要
Simultaneous localization and mapping (SLAM) technology is essential for robots to navigate unfamiliar environments. It utilizes the sensors the robot carries to answer the question “Where am I?” Of the available sensors, cameras are commonly used. Compared to other sensors like light detection and ranging (LiDARs), the method based on cameras, known as visual SLAM, has been extensively explored by researchers due to the affordability and rich image data cameras provide. Although conventional visual SLAM algorithms have been able to accurately build a map in static environments, dynamic environments present a significant challenge for visual SLAM in practical robotics scenarios. While efforts have been made to address this issue, such as adding semantic segmentation to conventional algorithms, a comprehensive literature review is still lacking. This article discusses the challenges and approaches of visual SLAM with a focus on dynamic objects and their impact on feature extraction and mapping accuracy. First, two classical approaches of conventional visual SLAM are reviewed; then, this article explores the application of deep learning in the front-end and back-end of visual SLAM. Next, visual SLAM in dynamic environments is analyzed and summarized, and insights into future developments are elaborated upon. This article provides effective inspiration for researchers on how to combine deep learning and semantic segmentation with visual SLAM to promote its development.
更多查看译文
关键词
visual slam integration,semantic segmentation,deep learning
AI 理解论文
溯源树
样例
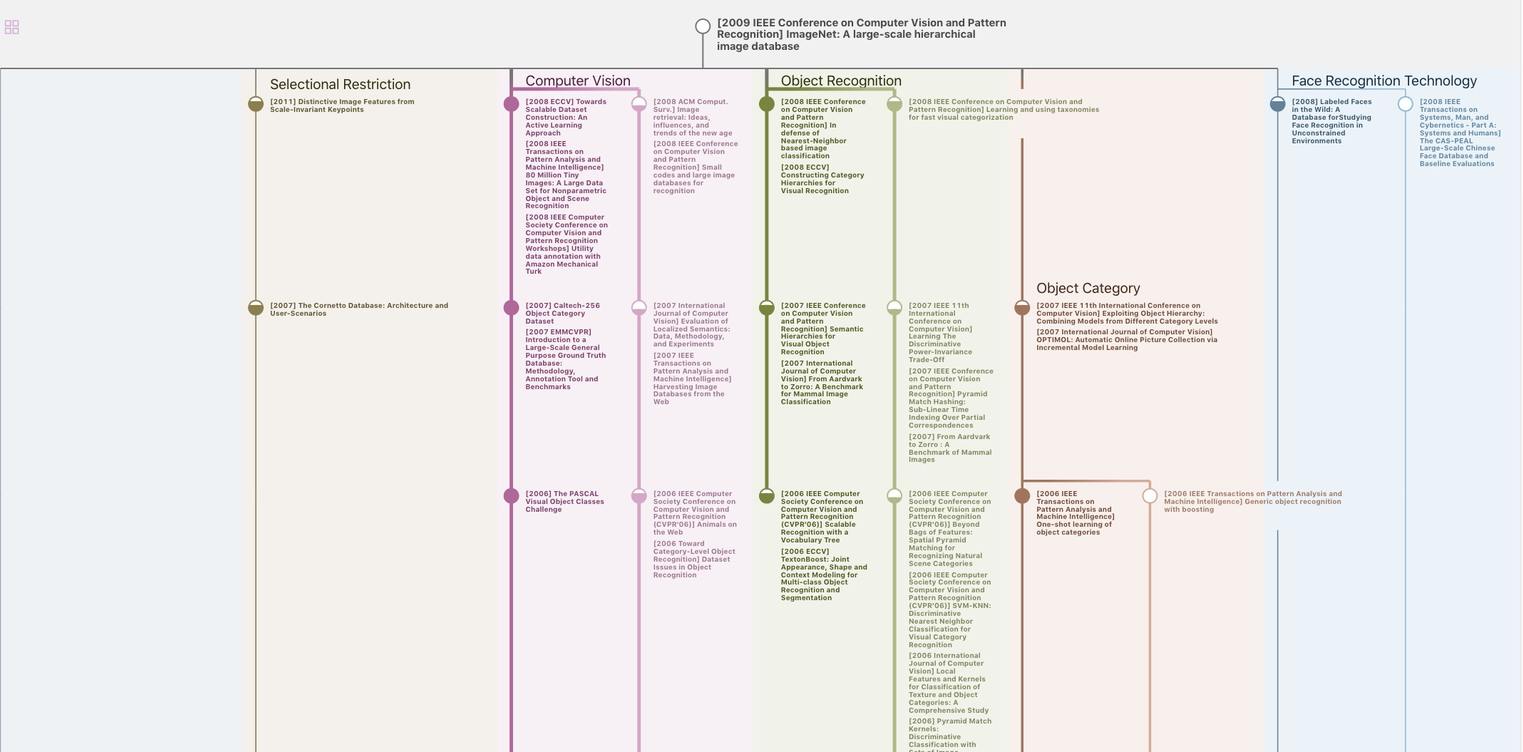
生成溯源树,研究论文发展脉络
Chat Paper
正在生成论文摘要