A Heterogeneous Computer Architecture Accelerating Reinforcement Learning-based Design for Silicon Photonic Devices
2023 IEEE 34th International Conference on Application-specific Systems, Architectures and Processors (ASAP)(2023)
摘要
This paper proposes a framework to substantially accelerate Reinforcement Learning (RL)-based design method for Photonic Integrated Circuit (PIC) devices. PICs are widely anticipated to underpin the forthcoming generation of semiconductor chips. However, the complexity of PIC design, which includes hundreds of degrees of freedom (DOF), presents considerable challenges. Machine Learning (ML) techniques, inclusive of RL, have demonstrated their effectiveness in the domain of PIC design. The primary hurdle, however, is the extended training duration, primarily constrained by the sluggish electromagnetic (EM) solver, specifically, the Finite-Difference Time-Domain (FDTD) solver. We have engineered a novel computational architecture that can be deployed on cloud-based systems using a cluster of Central Processing Units (CPUs), Field-Programmable Gate Arrays (FPGAs), and Graphics Processing Units (GPUs). An FPGA-FDTD accelerator, which capitalizes on the high memory bandwidth of on-chip memory (OCM), has been specifically designed to simulate planar PIC devices. Each FPGA-FDTD accelerator, also denoted as an FPGA kernel, functions as an autonomous RL environment. The host machine, in conjunction with the FPGA kernel, is designated as a worker node within the cluster. A functional prototype has been successfully implemented on the Amazon Web Services (AWS) cloud. The framework has effectively designed numerous PIC devices, and experimental results show the architecture outperforms existing methods significantly in design speed while maintaining or exceeding their design quality. Notably, the framework's versatility requires minimal adjustments for a broad range of devices, significantly reducing design time and promising to expedite PIC innovation.
更多查看译文
关键词
Silicon photonic devices, Finite Difference Time Domain, Machine Learning, Cloud Computing
AI 理解论文
溯源树
样例
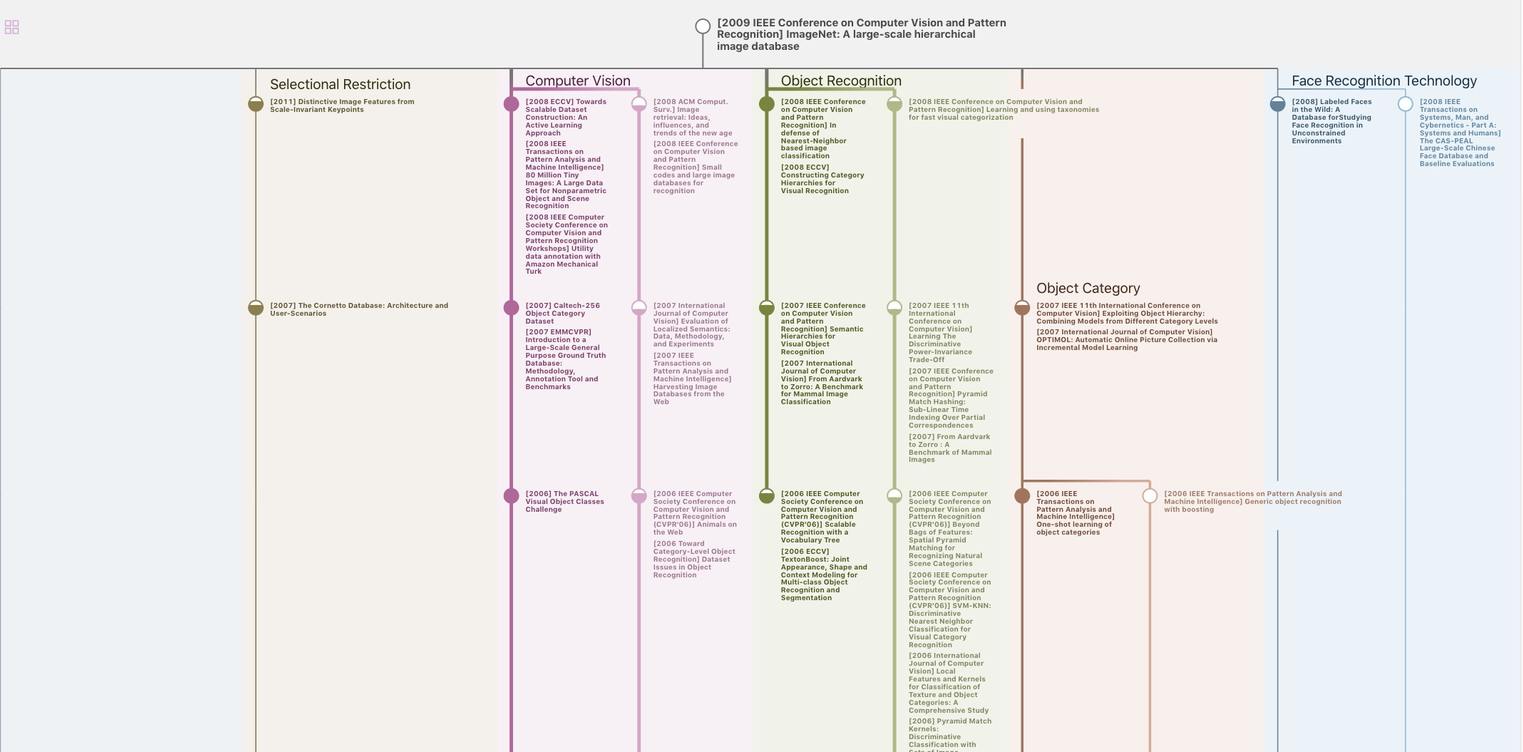
生成溯源树,研究论文发展脉络
Chat Paper
正在生成论文摘要