Knowledge Crosswords: Geometric Reasoning over Structured Knowledge with Large Language Models
CoRR(2023)
摘要
Large language models (LLMs) are widely adopted in knowledge-intensive tasks and have achieved impressive performance thanks to their knowledge abilities. While LLMs have demonstrated outstanding performance on atomic or linear (multi-hop) QA tasks, whether they can reason in knowledge-rich scenarios with interweaving constraints remains an underexplored problem. In this work, we propose geometric reasoning over structured knowledge, where pieces of knowledge are connected in a graph structure and models need to fill in the missing information. Such geometric knowledge reasoning would require the ability to handle structured knowledge, reason with uncertainty, verify facts, and backtrack when an error occurs. We propose Knowledge Crosswords, a multi-blank QA dataset where each problem consists of a natural language question representing the geometric constraints of an incomplete entity network, where LLMs are tasked with working out the missing entities while meeting all factual constraints. Knowledge Crosswords contains 2,101 individual problems, covering various knowledge domains and further divided into three difficulty levels. We conduct extensive experiments to evaluate existing LLM prompting approaches on the Knowledge Crosswords benchmark. We additionally propose two new approaches, Staged Prompting and Verify-All, to augment LLMs' ability to backtrack and verify structured constraints. Our results demonstrate that while baseline approaches perform well on easier problems but struggle with hard ones, our proposed Verify-All outperforms other methods by a large margin and is more robust with hard problems. Further analysis reveals that LLMs' ability of geometric reasoning over structured knowledge is still far from robust or perfect, susceptible to confounders such as the order of options, certain structural patterns, assumption of existence of correct answer, and more.
更多查看译文
关键词
structured knowledge,geometric reasoning,models
AI 理解论文
溯源树
样例
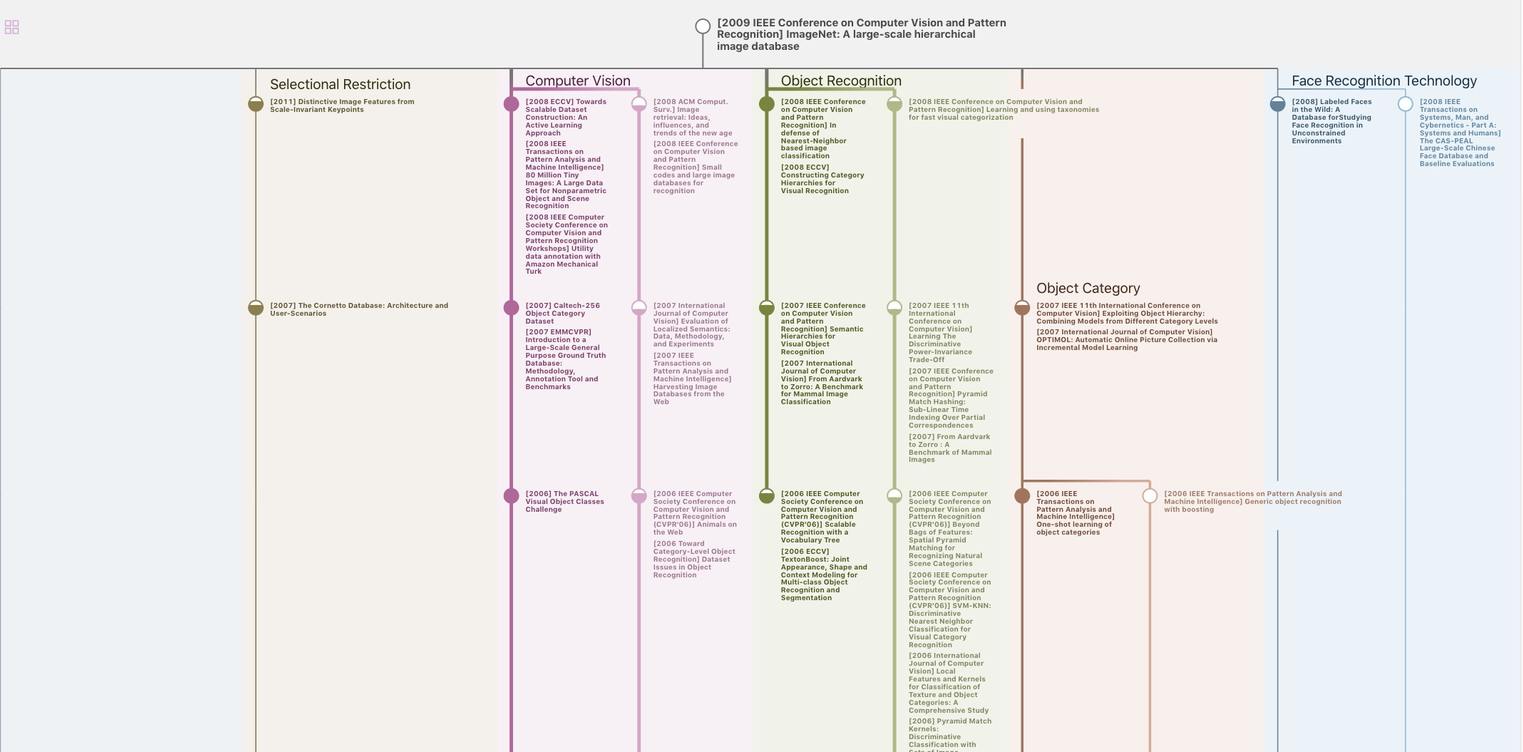
生成溯源树,研究论文发展脉络
Chat Paper
正在生成论文摘要