Going Beyond Familiar Features for Deep Anomaly Detection
CoRR(2023)
摘要
Anomaly Detection (AD) is a critical task that involves identifying observations that do not conform to a learned model of normality. Prior work in deep AD is predominantly based on a familiarity hypothesis, where familiar features serve as the reference in a pre-trained embedding space. While this strategy has proven highly successful, it turns out that it causes consistent false negatives when anomalies consist of truly novel features that are not well captured by the pre-trained encoding. We propose a novel approach to AD using explainability to capture novel features as unexplained observations in the input space. We achieve strong performance across a wide range of anomaly benchmarks by combining similarity and novelty in a hybrid approach. Our approach establishes a new state-of-the-art across multiple benchmarks, handling diverse anomaly types while eliminating the need for expensive background models and dense matching. In particular, we show that by taking account of novel features, we reduce false negative anomalies by up to 40% on challenging benchmarks compared to the state-of-the-art. Our method gives visually inspectable explanations for pixel-level anomalies.
更多查看译文
关键词
familiar features,deep,detection
AI 理解论文
溯源树
样例
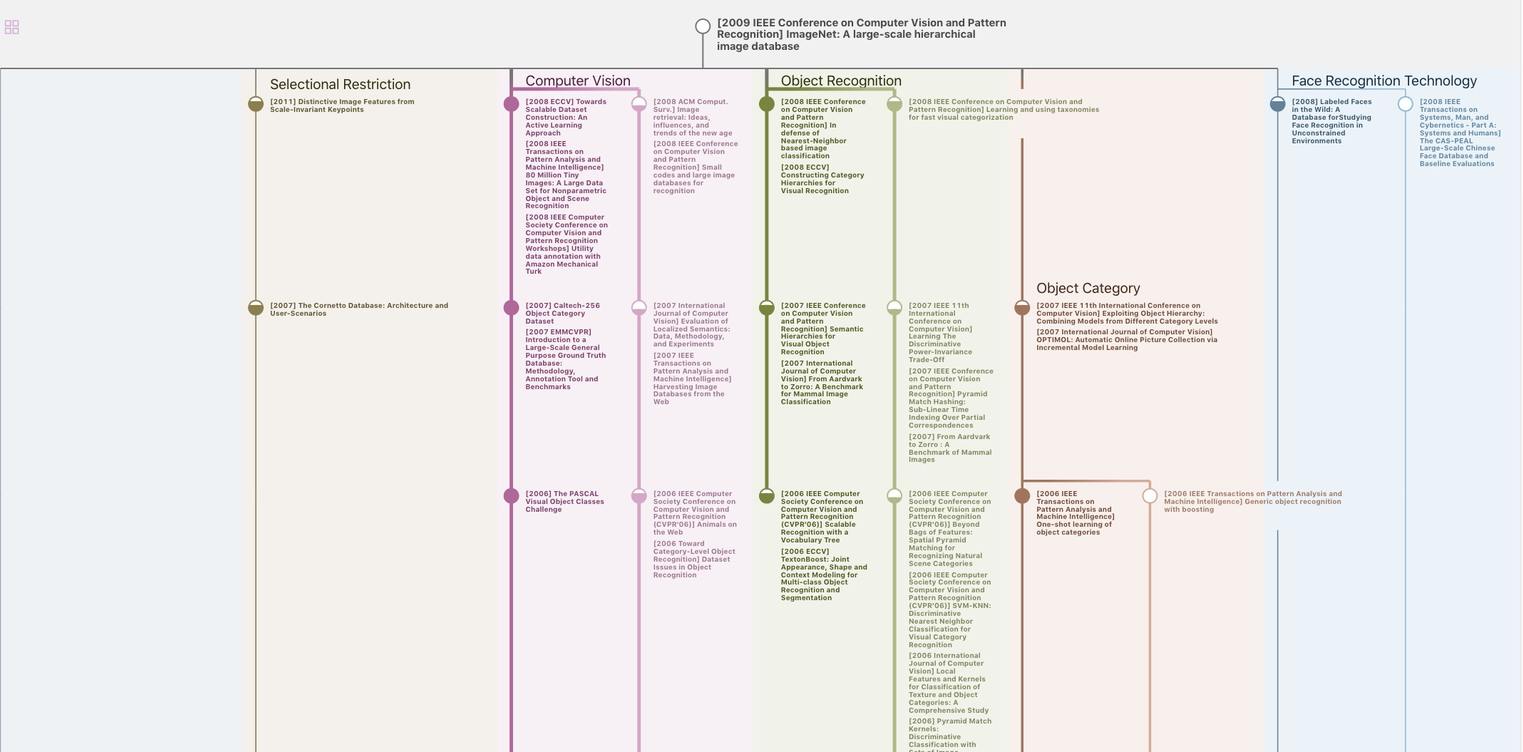
生成溯源树,研究论文发展脉络
Chat Paper
正在生成论文摘要