SVRNet: First Investigation of Single-View Reconstruction Network for Fluorescence Molecular Tomography
IEEE Transactions on Computational Imaging(2023)
摘要
Fluorescence molecular tomography (FMT) remains challenging for accurate monitoring of fast biological processes in vivo due to the long data acquisition time and complex iterative computational process. The limited-view-based algorithms allow for three-dimensional (3D) FMT reconstructions using fewer projections by reducing data acquisition time. However, the previously limited-view FMT studies usually need to acquire at least three projections or more, and almost all are based on time-consuming regularization methods, which brings a huge bottleneck to fast imaging of living animals. Single-view FMT reconstruction has not been investigated due to extremely insufficient measurement data and the severely ill-conditioness of the inverse problem. To solve this intractable problem, we propose a novel single-view reconstruction network (SVRNet) to achieves FMT reconstruction. First, a global-local hybrid multi-head self-attention mechanism was carefully elaborated to capture both local and long-range pixel interactions simultaneously. Further, rich and accurate contextual associations in the spatial domain can be obtained to exploit practical projection information. Second, we integrated neural network priors as the regularizers to explore deep features within limited measurements and impose constraints on the solution space of the imaging domain, significantly mitigating the ill-conditioned nature of the inverse problem. Results from numerical simulations, physical phantoms, and in vivo mouse experimental results prove that the proposed SVRNet method using a single projection achieves excellent imaging quality in terms of target localization accuracy, target shape recovery, and spatial resolution. This single-view reconstruction method can enable ultrafast FMT imaging and may help simplify the hardware design of FMT systems.
更多查看译文
关键词
fluorescence molecular tomography,single-view
AI 理解论文
溯源树
样例
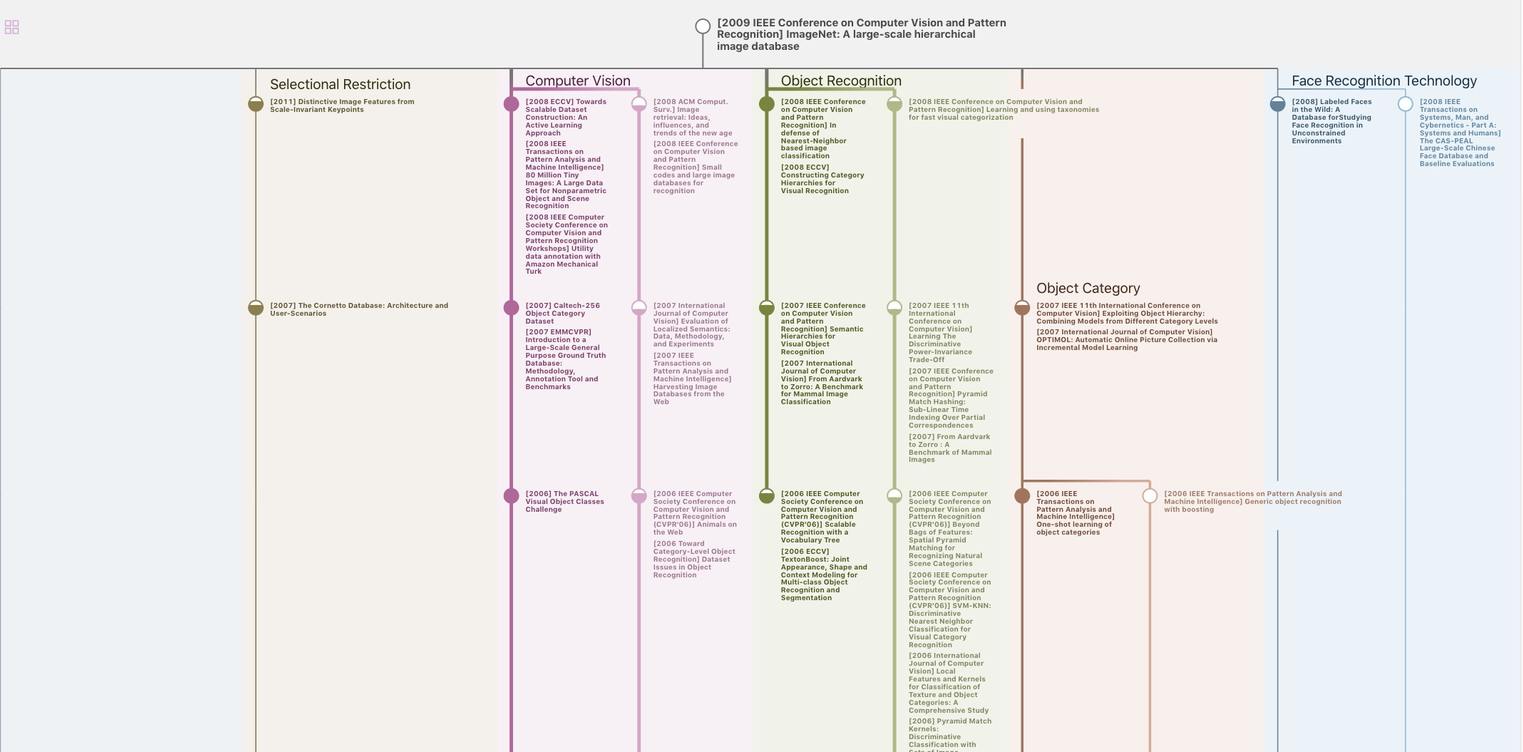
生成溯源树,研究论文发展脉络
Chat Paper
正在生成论文摘要