QoS Aware Slice Resource Management Using Deep Reinforcement Learning in IoT Networks
2023 19th International Conference on Distributed Computing in Smart Systems and the Internet of Things (DCOSS-IoT)(2023)
摘要
WiFi, a widely used technology in IoT, provides QoS through IEEE 802.11e EDCA Access Categories (AC) which have fixed configuration with strict priorities. This makes WiFi QoS unsuitable to the rising QoS diversity, changing wireless conditions and network dynamics. QoS slicing, where network resources are divided into chinks for diverse QoS requirements, is a potent technology that can provide more flexible, adaptable and highly configurable QoS in
$W$
iFi based IoT networks. However, resource management of QoS slices with diverse IoT applications, limited network capacity and varying channel conditions is a complex and challenging task. Traditional queuing theoretic and optimization models become intractable to solve such complex and dynamic problem. Therefore, we have developed a Deep Reinforcement Learning (DRL) based slice resource management scheme to meet IoT QoS requirements in a real world 5GEmpower controlled SDN network. Our proposed scheme outperforms Airtime Excess Round Robin (ATERR) scheme and no slicing-based scheme in terms of slice throughput (QoS) satisfaction. Moreover, our proposed scheme is tested in real world environment and can adapt to the changing slice requirements in an IoT network.
更多查看译文
关键词
WiFi Slicing,Deep Reinforcement Learning,QoS in WiFi,Adaptive Slice Management,Airtime Management
AI 理解论文
溯源树
样例
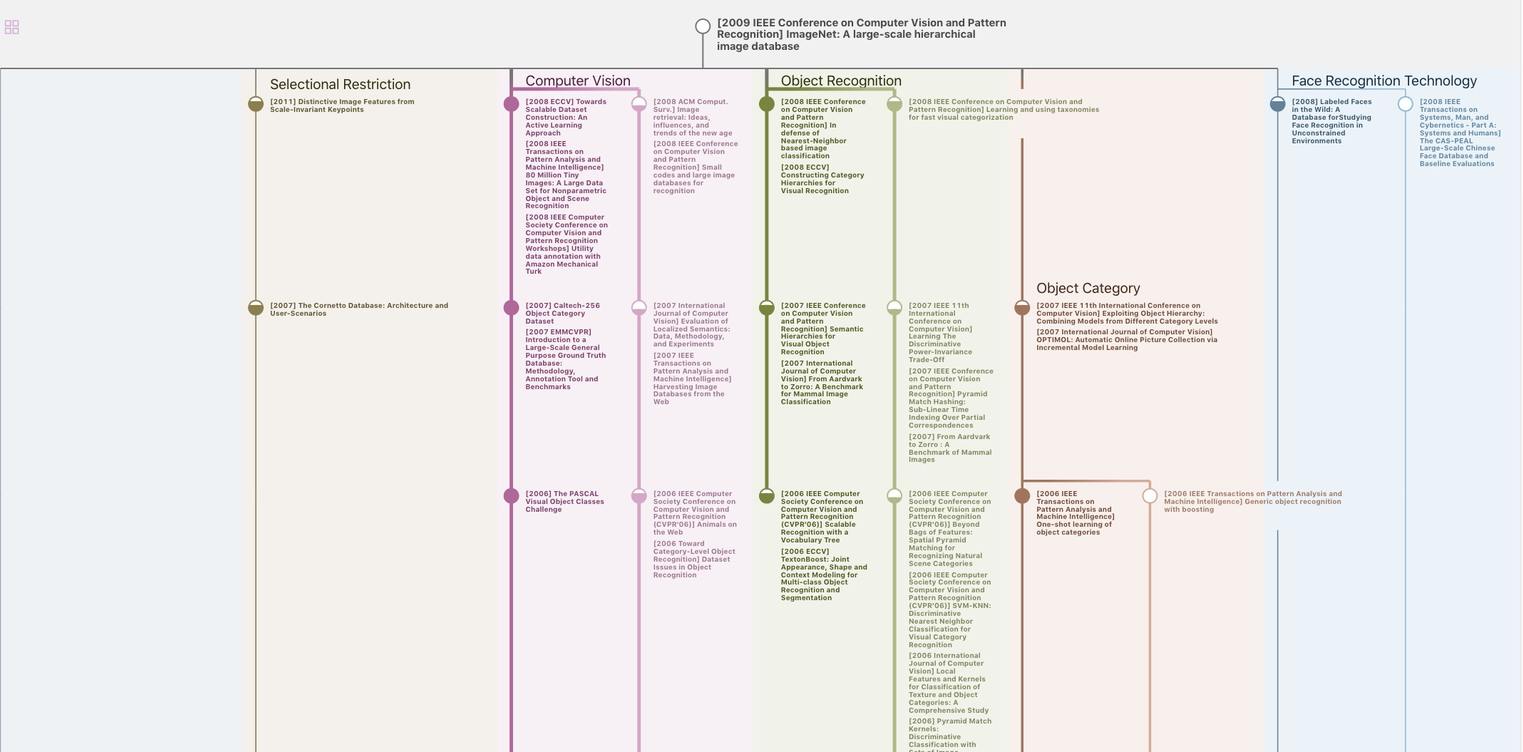
生成溯源树,研究论文发展脉络
Chat Paper
正在生成论文摘要